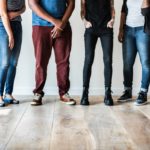
Depression is a leading cause of global disability worldwide. There are two main classes of treatment: pharmacological (medication) and psychological (talking therapies). Both classes of treatment are successful in around 50% of cases, but there is often quite a bit of trial and error in getting people onto the right treatment for them.
This review examines evidence for factors associated with outcomes for antidepressant medication. The factors examined include not just clinical and demographic variables, but also biomarkers including both genetic and neuroimaging data.
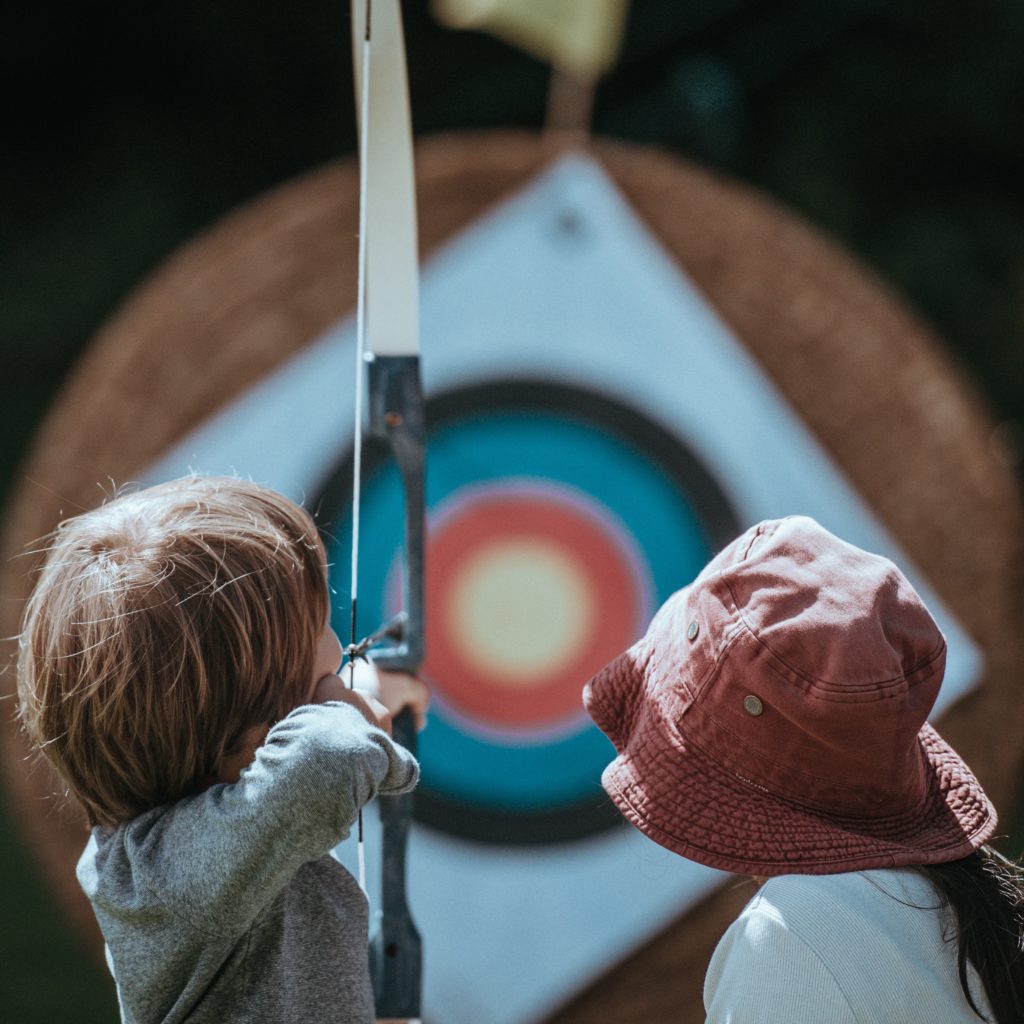
If we knew more about what works for whom and why, we would be able to offer more targeted personalised treatment for people with depression.
Methods
The authors searched Embase and MEDLINE for relevant reviews and meta-analyses on predictors of antidepressant response in adults with major depression as their primary diagnosis. Papers had to have been published between 1996 and 2017. From the papers identified, a list of predictors was created, which they allocated to one of 6 groups.
Three of these are traditional clinical variables:
- Demographic
- Symptom profile
- Comorbidities.
Three are types of biomarkers:
- Peripheral (e.g. urine, blood, saliva etc.)
- Genetic
- Neuroimaging.
Results
A total of 199 studies were included in the review. The results are summarised here in terms of the 6 categories of predictors.
Demographic variables
This category was quite broad and included BMI (body mass index) and measures of the social environment as well as typical demographic variables such as education and employment.
- Predictors of poor antidepressant response included old age, lack of social support, living with first-degree relatives with psychiatric symptoms, poor health in a caregiver and/or reported difficulty in caring for the index patient, a history of child trauma/abuse, cigarette smoking and alcohol use.
- Employment, high income, and high level of educational attainment are associated with better outcomes.
- Ethnicity and gender were found to be useful covariates. Neither variable had a consistent main effect on poor outcome, but the predictive effect of some other variables differed across ethnic groups or genders.
Symptom profiles
This included both the range of symptoms reported by the patient and variables associated with symptoms such as age of onset, duration and severity.
- Symptom level predictors of poor antidepressant response included greater symptom severity, anhedonia, cognitive impairment, neuroticism, and anxiety symptoms.
- Episode level predictors of poor antidepressant response included both early and late first onset, increased duration, recurrence, and prior hospitalisations.
- Response within two weeks of commencing treatment is associated with better outcomes.
- Some features are associated with different antidepressants in different ways so may be useful treatment specifiers (Kennedy et al., 2016).
Comorbidities
This included both comorbid medical and psychiatric disorders.
- Predictors of poor antidepressant response include a wide array of comorbid medical and psychiatric illness.
- Comorbid anxiety disorder is generally associated with poorer response to most antidepressants, but there is also some evidence for differential response to different types of antidepressants, so this may also be a useful treatment specifier.
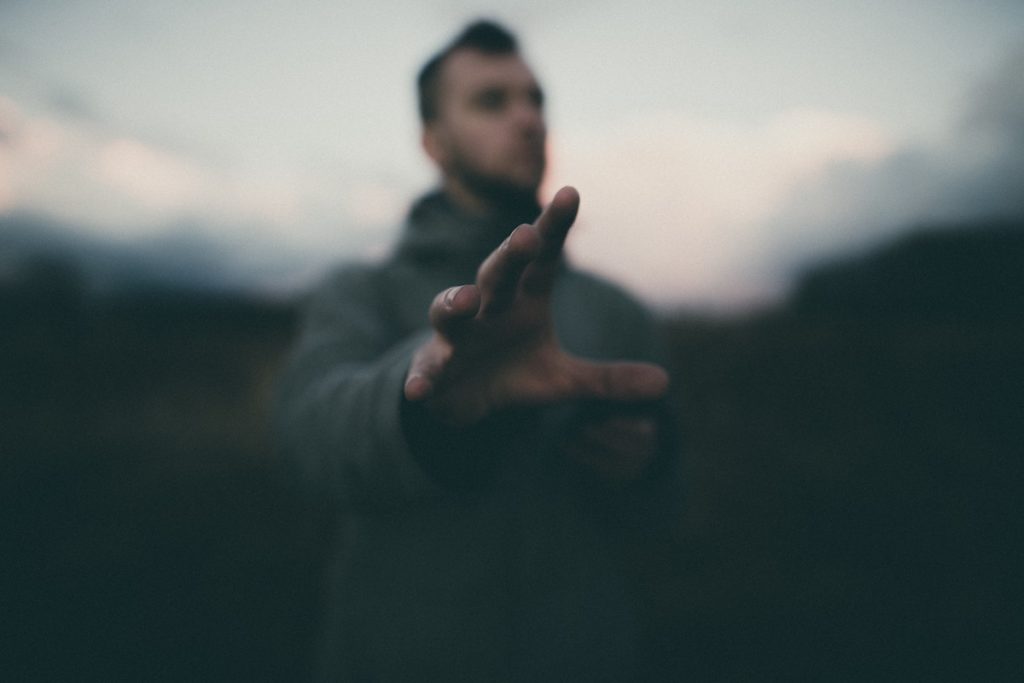
Predictors of poor antidepressant response included old age, lack of social support, living with first-degree relatives with psychiatric symptoms, poor health in a caregiver and/or reported difficulty in caring for the index patient, a history of child trauma/abuse, cigarette smoking and alcohol use.
Peripheral markers
This group of markers included urine, blood saliva and/or cerebrospinal fluid. There was a particular focus in this group of markers on those relating to immune function, given the increased attention to the “immune hypothesis” of depression (Lopresti et al, 2014).
- Predictors of poor antidepressant response include elevated proinflammatory cytokine levels, cortisol levels (though the direction of association is inconsistent – perhaps due to timing or source of cortisol sample and variable physical health of participants), lack of increase in serum BDNF levels after 2 weeks of treatment, greater baseline triglyceride and cholesterol levels,
- Higher C-Reactive Protein levels show variable direction of association with different antidepressants, and thus may be a useful treatment specifier.
- Low urinary MHPG (a metabolite of norepinephrine) is associated with improved response to serotonin selective reuptake inhibitors (SSRIs) including fluoxetine and desipramine (a tricyclic antidepressant).
Genetic markers
The review primarily focuses on candidate genes. However, as written about on Mental Elf previously, this approach has now been shown to be flawed and contemporary genome-wide approaches should be used. The difficulty with these is that very large sample sizes are needed, but with collaborative efforts, such as the work of the Psychiatric Genomics Consortium, useful findings can be achieved.
- Individuals with a family history of affective disorders are less likely to respond well to antidepressants. Furthermore, those with a first-degree relative who has responded well to antidepressants are more likely to respond well. These findings support a genetic role on outcomes.
- Numerous candidate gene markers have been examined but the evidence is inconsistent and should not be interpreted with any confidence.
- The strongest evidence of genetic influence on antidepressant response comes from a paper which was not included in the current review, presumably because it does not involve a single predictor. A genome-wide association study of antidepressants in ~2,800 patients with major depression (Tansey et al., 2013) found that common genetic variants accounted for 42% of the variation in response; more than double what common genetic variants contribute to depression itself and indicating that larger studies may yield useful predictors.
Neuroimaging markers
This group of markers includes both structural and functional measures of the brain.
- Predictors of poor antidepressant response using electroencephalography (EEG) include increased frontal theta band activity, and the antidepressant response index (which combines relative and absolute alpha power). This latter index is also potentially useful as a predictor of differential response to different antidepressants. Evidence for other EEG predictors is weaker.
- Predictors of poor antidepressant response using structural MRI include cortical thickness, grey matter volume and white matter integrity. For example, reduced hippocampal volume is associated with poorer treatment outcomes.
- Predictors of poor antidepressant response using functional MRI include reductions in both anterior cingulate cortex and amygdala function.
- Predictors of improved antidepressant response using functional MRI include increased functional connectivity between frontal and limbic brain regions and decreased connectivity within the default mode network.
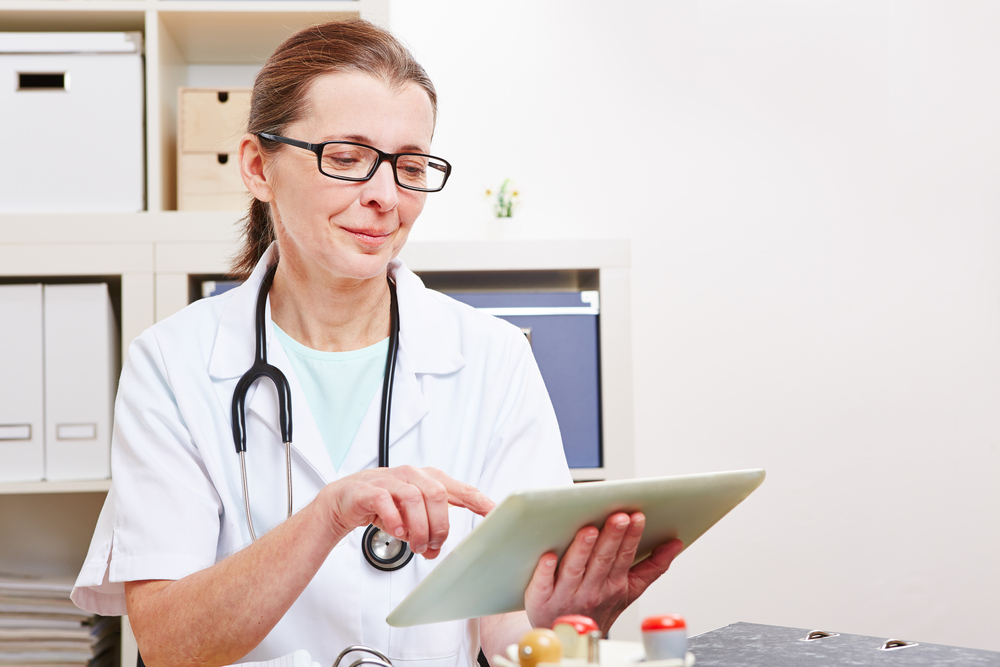
This review highlights a huge range of predictors of treatment response that vary widely in their clinical utility.
Conclusions
The authors begin their discussion by noting that despite this vast array of data in this area, few if any of these predictors have made it into clinical practise. This is partly due to the mixed levels of support for these predictors, many of which have yet to be firmly replicated. They note the emergence of several large scale studies focused on treatment prediction, and the potential these have to provide replicable findings on biomarkers.
Three key themes emerged for us as we read the discussion:
Differential response
The authors note that for these findings to be useful in clinical practise we need to identify predictors of differential response; showing that one medication will suit someone better than another. Or to take this further, to show whether medication, psychological treatment or both are most likely to be optimal for any one individual. Such personalised medicine will come, but it will take far larger interdisciplinary studies than have been conducted to date.
Multivariate prediction
The predictors reviewed were considered individually, within their relevant groups. But as the authors point out, it is likely that our best chances of useful prediction will come from combining predictors for multivariate prediction of outcomes. Statistical approaches such as multiple linear and polynomial regression/machine learning, and the use of big data (e.g. record linkage) should lead to significant advances in the next decade.
Practical implementation
For predictors to be useful in clinic they need to be cost-effective. Thus EEG is considerably more likely to be of clinical utility than MRI. Genome-wide association data can now be obtained for around £30 per person, meaning that this information can also be used in primary care at reasonable cost. However, critically, this approach relies on sufficiently large reference studies of relevant outcomes, e.g. treatment response, being carried to develop useful predictors. Another critical issue is the timing of collection of the predictor variable; those that can be collected at baseline are likely to be most useful.
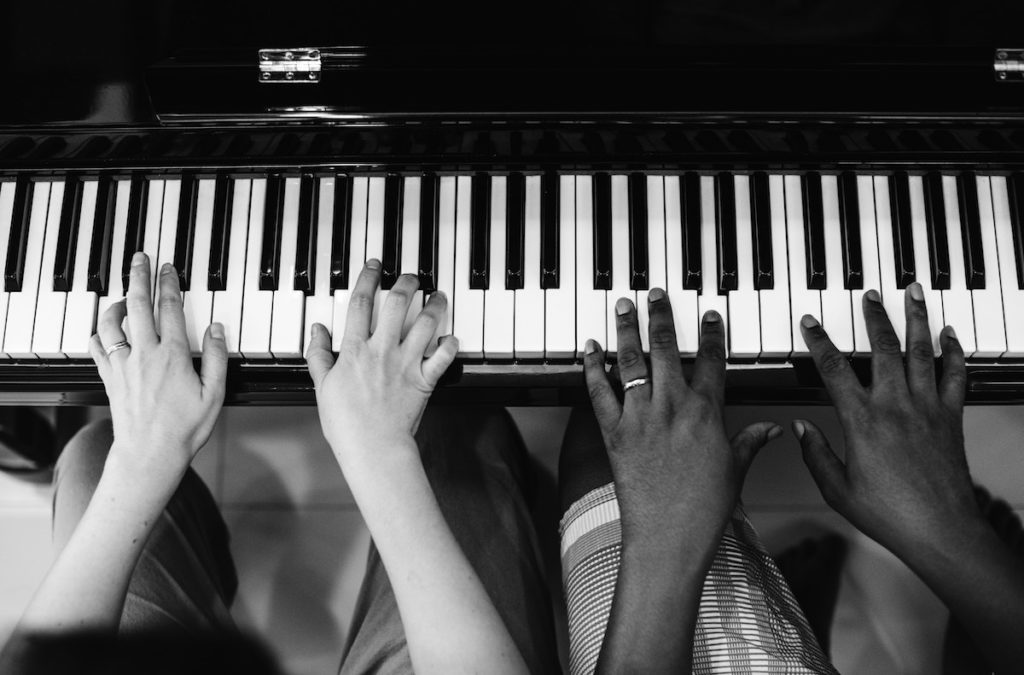
Personalised medicine for depression will come, but it will take far larger interdisciplinary studies than have been conducted to date.
The #GLADstudy
The authors conclude in their abstract with:
We highlight the importance of large-scale research initiatives and clinically accessible biomarkers, as well as the need for replication studies of current findings.
With this in mind, we draw the reader’s attention to the new Genetic Links to Anxiety and Depression (GLAD) Study which hopes to change the landscape of research into these conditions in the UK. This initiative involves recruiting 40,000 participants who have experienced clinical depression or anxiety during their life into a recountable database of participants to enable future studies on these condition, particularly with regard to treatment outcomes. Taking part involves signing-up online, providing consent, completing an enrolment questionnaire and providing a saliva sample through the post. Recruitment is well underway, and as this goes to print, over 20,000 individuals have registered on the website of whom 10,000 have completed the enrolment questionnaires.
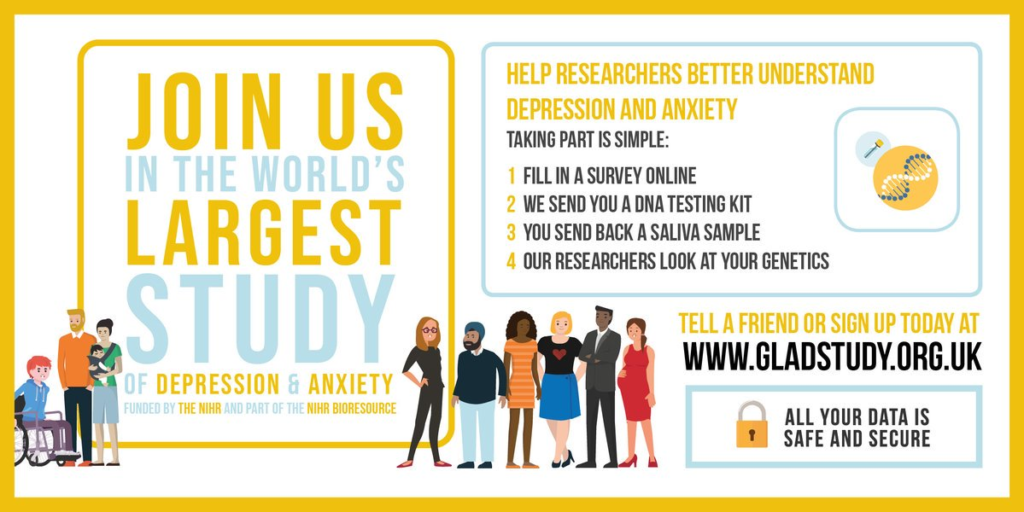
The GLAD study is recruiting now! Find out more at gladstudy.org.uk
Links
Primary paper
Perlman K, Benrimoh D, Israel S, Rollins C, Brown E, Tunteng J, You R, You E, Tanguay-Sela M, Snook E, Miresco M, Berlim MT. (2018) A systematic meta-review of predictors of antidepressant treatment outcome in major depressive disorder. Journal of Affective Disorders, Volume 243, 2019, Pages 503-515. [PubMed abstract]
Other references
Kennedy, S.H., Lam, R.W., McIntyre, R.S., Tourjman, S.V., Bhat, V., Blier, P., Hasnain, M., Jollant, F., Levitt, A.J., MacQueen, G.M., McInerney, S.J., McIntosh, D., Milev, R.V., Müller, D.J., Parikh, S.V., Pearson, N.L., Ravindran, A.V., Uher, R., the, C.D.W.G., (2016). Canadian network for mood and anxiety treatments (CANMAT) 2016 clinical guidelines for the management of adults with major depressive disorder: section 3. pharmacological treatments. Can. J. Psychiatry 61, 540–560.
Lopresti, A.L., Maker, G.L., Hood, S.D., Drummond, P.D., 2014. A review of peripheral biomarkers in major depression: the potential of inflammatory and oxidative stress biomarkers. Prog. Neuro-psychopharmacol. Biol. Psychiatry 48, 102–111.
Tansey KE, Guipponi M, Hu X, Domenici E, Lewis G, Malafosse A, Wendland JR, Lewis CM, McGuffin P, Uher R. (2013) Contribution of common genetic variants to antidepressant response. Biol Psychiatry. 2013 Apr 1;73(7):679-82. doi: 10.1016/j.biopsych.2012.10.030. Epub 2012 Dec 11. [PubMed abstract]
Photo credits
- Photo by rawpixel on Unsplash
- Photo by Annie Spratt on Unsplash
- Photo by Simon Migaj on Unsplash
- Photo by rawpixel on Unsplash