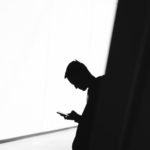
In July 2008 the App Store opened on the iPhone. This was a first for digital consumer products and promised unprecedented access to software anytime, anywhere (as long as your battery didn’t die after about 10 minutes of use). It had huge implications for games, tools and productivity, with loads of market space for new start-ups to get in on the action; from Angry Birds to Evernote. However, the app revolution didn’t only create opportunities to shoot zombie pigs at range with a macaw, it also represented huge potential for a new type of health care.
The ability to input your own health data, track your progress, and receive advice on one device means that care can be personalised, affordable, and accessible to anyone with a smartphone. Apps can also be used in conjunction with services, acting as a self-care step before seeing a clinician or being used to show your keyworker how you’ve been in the last few weeks at the touch of a button.
Nevertheless, just as with any health care intervention, apps need to be carefully tailored toward those they aim to help. For example, carrying out research into adherence (that is, helping people stick with using the app) may hugely improve a digital intervention’s potential for curbing the symptoms of psychosis.
- But what are the precise predictors of adherence?
- Does the severity of symptoms affect the way in which someone interacts with a digital intervention?
- Does involving service users in the design of the app generate a more appealing piece of software?
A new study from Clare Killikelly and colleagues aimed to answer these questions by systematically reviewing the current scientific literature around potential predictors of adherence to apps for people with psychosis (Killikelly et al, 2017).
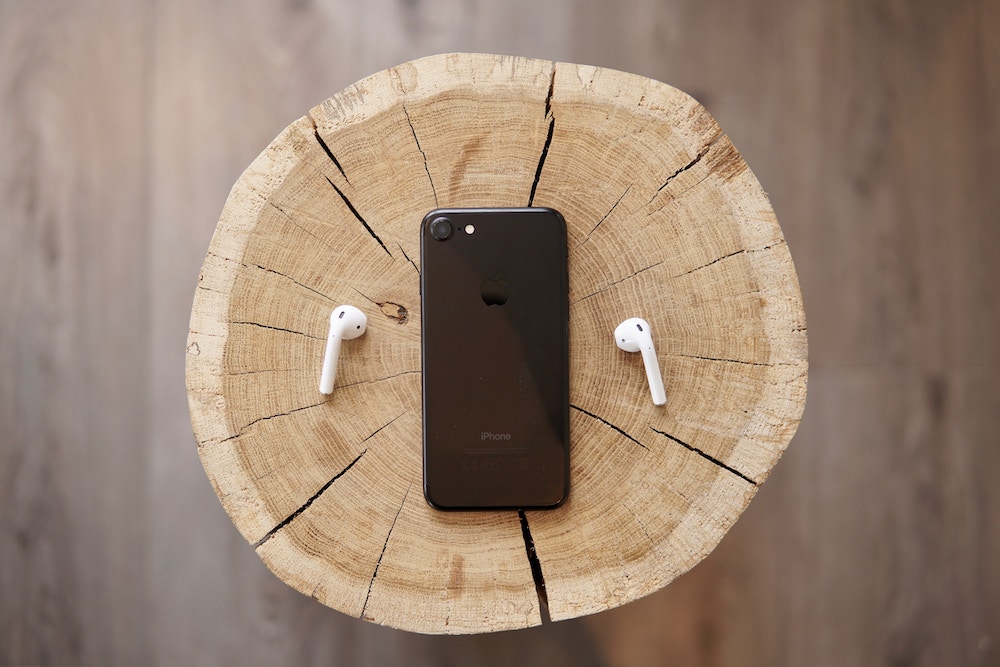
This new review explored the factors that predicted adherence to digital technology in people with psychosis.
Methods
Searching through 5 online databases, authors identified studies adhering to the following criteria:
- Adults aged 18-65 with a schizophrenia spectrum disorder
- Was an intervention, trial, or observational study. Randomised Controlled Trials (RCTs), feasibility studies, cross-sectional, longitudinal comparison studies (with or without a control group) were all accepted
- Used a digital mental health app (this could be web- or mobile-based). The app could have either been a psychoeducation, therapeutic, monitoring, self-help or symptom-checking tool
- Measured adherence in some form.
From 2,639 studies initially identified, authors eventually whittled down 20 studies to include in the final analysis; excluding other studies for not being clinically relevant, having irrelevant research designs (e.g. posters and case-studies), or not including the relevant outcome measures.
RCTs and feasibility studies were assessed separately using the Clinical Trials Assessment Measure (CTAM), and observational studies were assessed using the Downs and Black scale; these are effectively different sets of recommended guidelines to enable a critique of their quality.
Results
The included studies covered 656 participants with schizophrenia spectrum disorders, between the ages of 20-48 years.
There’s a dense range of results reported in the article, so I’m going summarise key findings as best I can:
Study characteristics
6 RCTs, 7 feasibility trials, and 7 observational studies were reviewed. In the full article, the characteristics of the participants in each type of study is broken down, e.g. mean age, n, etc.
Quality assessment
The mean CTAM score for RCTs was 77.3/100, with only this study scoring below 65; the general benchmark score indicating adequate quality.
Due to lack of randomisation, feasibility studies had a lower mean of 44.7. Of the observational studies included, only 3 were deemed ‘good’, and 4 scored ‘fair’, so had a mean quality rating of 20.
Adherence: types of assessment across studies
The figure below probably best depicts the different approaches to adherence that each study recorded –the percentage of the intervention completed (average 83.4%) by participants indicated for the top set of studies, and the percentage of completed interventions (average 73.4%) indicated for the bottom set.
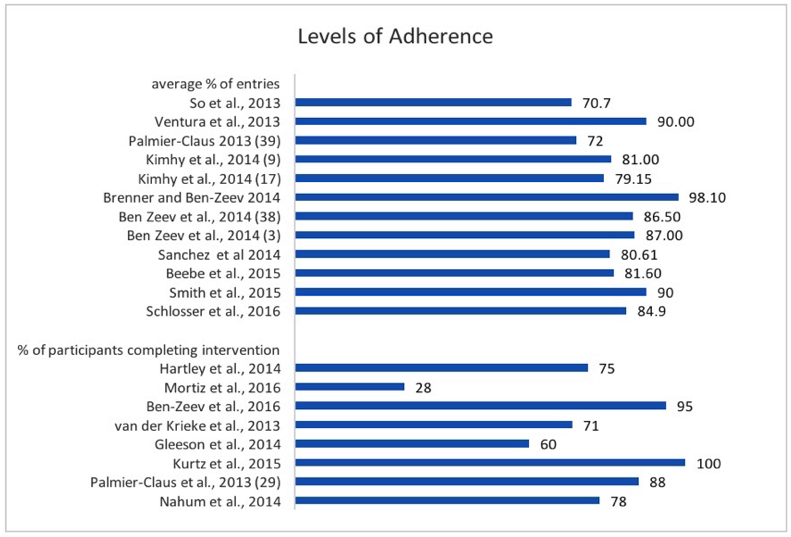
Figure taken from: Killikelly et al, 2017. Improving Adherence to Web-Based and Mobile Technologies for People With Psychosis: Systematic Review of New Potential Predictors of Adherence.
Which factors lead to dropout?
- Age and gender were found to have no effect in most studies
- Symptoms were concluded not to affect dropout (except in this one study)
- Cognitive function was looked at in one study, and not found to predict dropout
- Mobile phone based apps improved adherence vs. text-message-only technologies
- The degree of social support users had while taking part also improved adherence
- Service user involvement in the design of the tool improved adherence
Only four studies actually asked participants if they were keen to continue to use the intervention, and this averaged to 73.1% saying ‘yes’.
Strengths and limitations
This is a thorough review and the first of its kind, thereby making a positive contribution to the literature. The review identified important implications, which may help inform the delivery and development of e-health interventions.
Despite the comprehensive search that was undertaken, there were a relatively small number of studies to address each objective. As the authors noted, there was also heterogeneity in terms of the study interventions and outcomes. Overall, these issues made it difficult to make cross-study comparisons and draw more definitive conclusions about the significance of the review findings.
It was also noted that the authors excluded “conference abstracts and theses not published in a peer-reviewed journal”. Including articles from the grey literature could have helped identify novel technologies that have not yet undergone rigorous scientific testing (e.g. RCTs) but are nonetheless potentially valuable. This is particularly relevant to the field of e-health, where the development of technologies is outpacing research (Nilsen et al., 2012). Inclusion of non peer-reviewed sources may also reduce the risk of publication bias (McAuley, Tugwell, & Moher, 2000).
Discussion
Overall this study was dealing with a mixed bag of evidence: varying levels of quality, differing intervention designs, and a range of outcome measures. Quite rightly, authors write that the review provides an up-to-date and comprehensive look at the field and synthesises preliminary conclusions based on diverse existing research. As it stands, it is noted that future reviews should focus on splitting levels of adherence by intervention, i.e. adherence in web-based technologies vs. app-based technologies.
However, can we truly dismiss the claims that symptoms do not affect adherence to a digital health intervention? While the given evidence doesn’t seem to point toward symptoms having a marked effect, we do not have data on individuals who turned down the intervention (or weren’t selected) and those who dropped out. This might mask conclusions if participants’ symptoms weren’t taken into account upon sample selection, and the authors didn’t point this out clearly in the review or suggest ways in which this could be ascertained.
While differences between interventions were mentioned in the article, I still feel like the authors have missed out a vital aspect of digital technology that could really help users engage. User Experience and User Interface (commonly abbreviated to UXUI) is a huge aspect of design when it comes to commercial apps; they keep users engaged and make the process of navigating the app as low energy as possible. It’s something that I feel is often overlooked or left undiscussed in the clinical sphere, and it’s not clear why. While it is definitely important to get intervention content right, it would be interesting for future research to pursue whether highly developed graphical interfaces can produce increased adherence to, for example, computerised cognitive behavioural therapy compared with simple text-based apps with the same content.
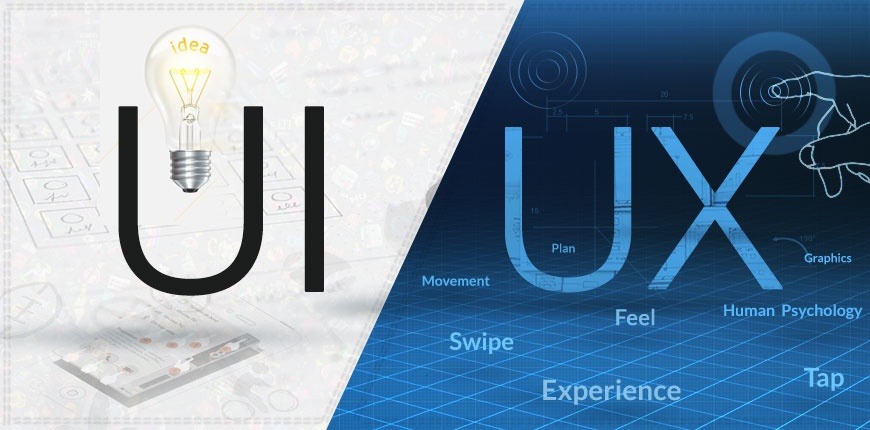
Do we need more User Experience as well as our current focus on User Interface?
Conclusion
This comprehensive review has given a great overview of the current literature, with all its variances, and suggested some useful steps to improve the field further. Moving forward, it might also be necessary to use some UXUI tactics to get service users engaged with the hope that it’ll further help the audience it’s intended for.
Conflict of interest
Joe Barnby is currently employed part-time for Thrive Therapeutic Software: a clinical software company developing digital interventions for mental health care. Thrive has not had any part in the writing or reviewing of this article.
Links
Primary paper
Killikelly C, He Z, Reeder C, Wykes T. (2017) Improving Adherence to Web-Based and Mobile Technologies for People With Psychosis: Systematic Review of New Potential Predictors of Adherence. JMIR Mhealth Uhealth 2017;5(7):e94 DOI: 10.2196/mhealth.7088
Other references
McAuley L, Tugwell P, Moher D. (2000) Does the inclusion of grey literature influence estimates of intervention effectiveness reported in meta-analyses?. The Lancet, 356(9237), 1228-1231.
Nilsen W, Kumar S, Shar A. et al (2012) Advancing the science of mHealth. Journal of health communication, 17(sup1), 5-10.
Photo credits
- Photo by Warren Wong on Unsplash
- Photo by Jaz King on Unsplash
[…] Les mer: Predictors of adherence to digital interventions for psychosis […]