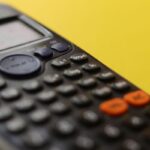
The devastating impact of victimisation is well documented, with studies showing that victimised children are at greater risk for both internalising and externalising disorders (McDougall & Vaillancourt 2015). Unfortunately, some experiences of victimisation are also quite common. As outlined in a previous Mental Elf blog by Lucy Bowes (2019), one study found that among 15-year olds in the UK, up to 30% reported being regularly bullied. This is quite frightening.
Fortunately, there is hope, as not all individuals subjected to victimisation experience later adjustment problems (Jackson & Deye, 2015). Take bullying for example, it has been found that around 15% of victims of frequent bullying have a diagnosis of depression by 18 years (Bowes et al., 2015). While this figure is still 15% too many, the findings show that a large proportion of victims are able to avoid ongoing problems with their mental health.
A key goal of recent research has therefore been to understand why some people, but not others, experience adverse outcomes following victimisation. A systematic review dedicated to exploring this revealed there are many factors, which the authors call ‘resilience factors’, that predict a reduced risk of mental health problems following adversities in childhood (Fritz et al., 2018). The authors noted, however, that most studies tend to investigate the individual contribution of each factor. Very few explored their combined predictive ability. The importance of more complex, multivariate models was therefore highlighted as a key avenue for future research.
In response to this beckoning call, I present the recent findings from Meeham and colleagues (2020). This study aimed to develop multivariate prediction models (or “risk calculators”) for victimised children.
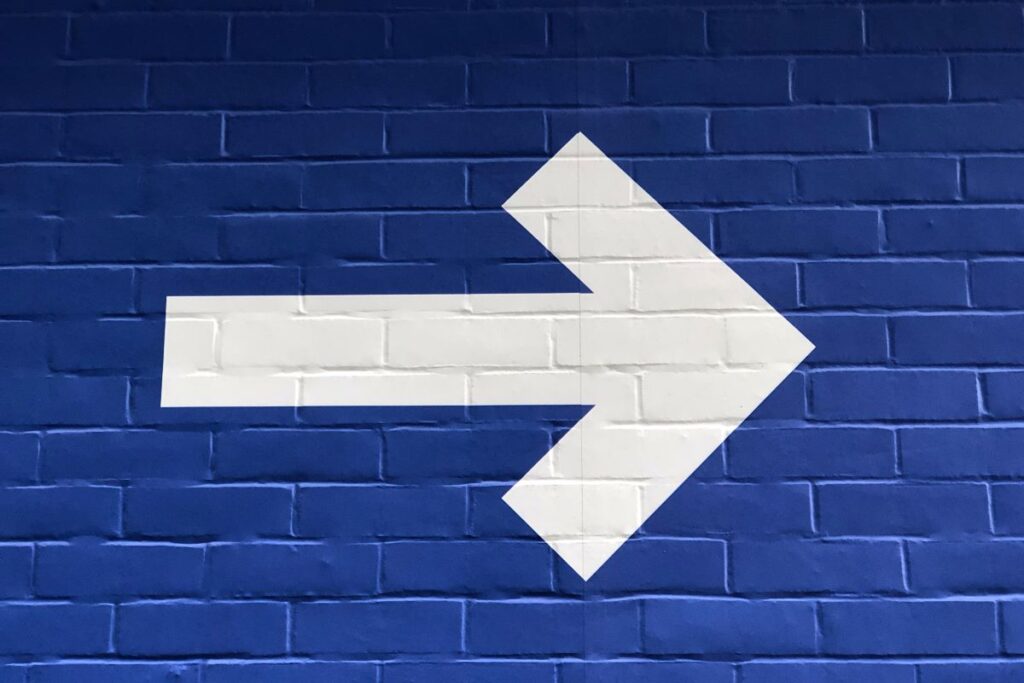
Understanding predictors of risk and resilience following victimisation is key to ensuring more targeted intervention strategies.
Methods
Participants
Participants were from a nationally representative British birth cohort, the Environmental Risk (E-Risk) Longitudinal Twin Study. This cohort comprises of 2,232 twins, born in 1994–1995. The current study made specific use of a subsample of individuals from this cohort (26.5%) who reported severe victimisation during childhood.
Victimisation
Experiences of victimisation were assessed between the ages of 5-12 years old, using scales related to physical domestic violence, bullying, sexual abuse, and physical and emotional neglect. Individuals were classed as a ‘victim’ if they rated any of these victimisation experiences as severe.
Predictors
Participants were also assessed between 5-12 years old for a range of individual-, family-, and community-level factors. These comprised of 22 predictors and were chosen based on a previous systematic review (Meng et al, 2018).
Outcome
At 18 years, participants completed a range of scales capturing internalising, externalising, and thought disorders. Internalising disorders included generalised anxiety disorder (GAD), major depressive disorder (MDD), post-traumatic stress disorder (PTSD) and eating disorders, while externalising disorders related to attention-deficit/hyperactivity disorder (ADHD), conduct disorder (CD), alcohol dependence, cannabis dependence and tobacco dependence. Thought disorders were based on experiences of psychotic symptoms such as hallucinations and delusions. Based on their responses, participants were assigned a score denoting the presence or absence of disorder for each of the three domains. An overall binary measure was also created to capture the presence of any internalising, externalising, or thought disorder. Main analyses used this overall measure and also explored internalising and externalising disorders separately. Thought disorders were not investigated individually given the low prevalence of psychotic symptoms.
Analysis
To explore the predictive ability of the individual-, family-, and community- level factors in determining risk for internalising and externalising disorders, logistic regularised regression models were used. Regularised regression allowed the authors to calculate individual rather than average risk, and helped to reduce the potential for over-fitting. To do this, regularised regression models essentially penalise overly complex models and place constraints on the error term. This helps exclude predictors that do not contribute much to the overall prediction model. The study authors used the Least Absolute Shrinkage and Selection Operator (LASSO).
Internal validation
Each model was internally validated to check predictive accuracy. This was carried out using the same population but with ‘unseen’ cases from the sample.
Results
Out of the 553 severely victimised participants, 60.4% (n=334) met the diagnostic criteria for at least one internalising, externalising, or thought disorder. When assessing risk for the disorders separately, 62.1% were shown to not have an internalising disorder, 56.7% did not have an externalising disorder, and 94.8% had no thought disorder.
Within the LASSO model predicting risk for all three psychiatric disorders, 17 of the 22 predictors remained. In models predicting just internalising disorders, 18 predictors remained, and 15 remained for externalising disorders. All personality dimensions were retained in the model of internalising disorders, but only extraversion contributed to the prediction of externalising disorders.
All models showed good calibration, meaning that the predicted probabilities performed well when plotted against the observed outcomes. The ability of the models to distinguish those with and without psychopathology was also adequate (AUC = 0.66 to 0.73) and in line with previous risk calculators in psychiatry (Hafeman, Merranko et al., 2017).
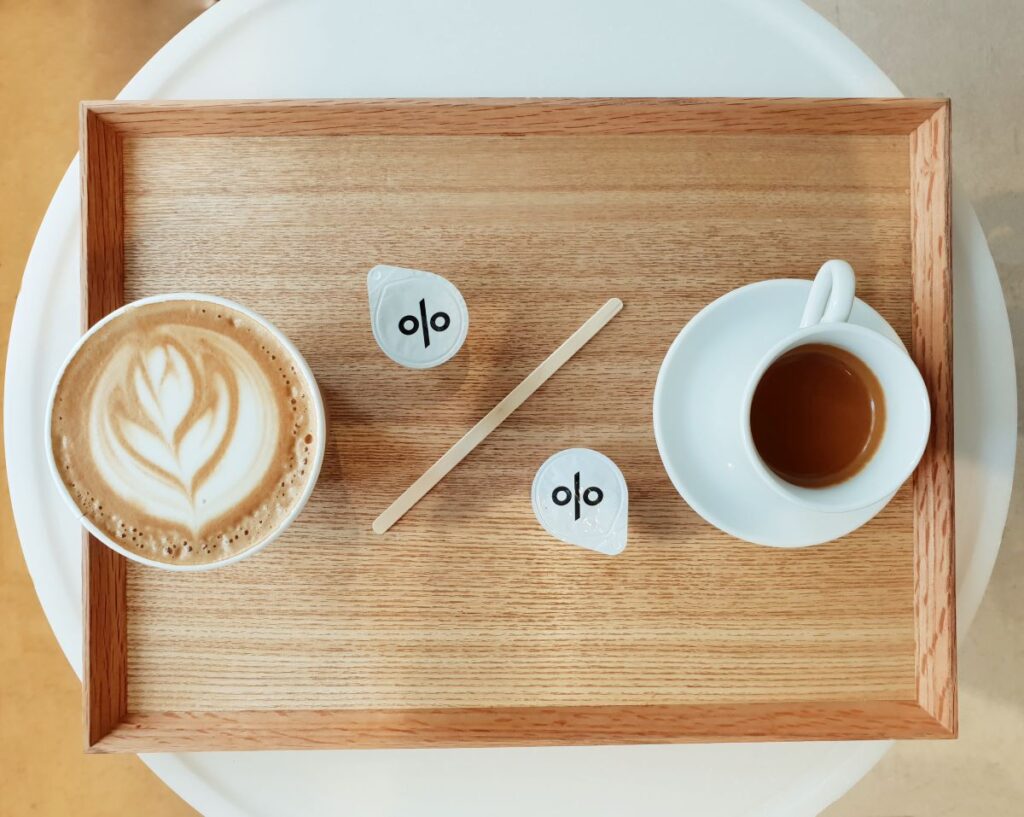
A large proportion (39.6%) of victimised children did not go on to develop internalising or externalising problems at 18 years.
Conclusions
- This study offers promising evidence that individualised risk estimates for mental ill-health following victimisation can be attained using multivariable prediction modelling.
- The models used to predict risk were well calibrated and showed typical discrimination abilities when compared to existing models in psychiatry.
- With further external validation, such models could be used to support more targeted intervention strategies.
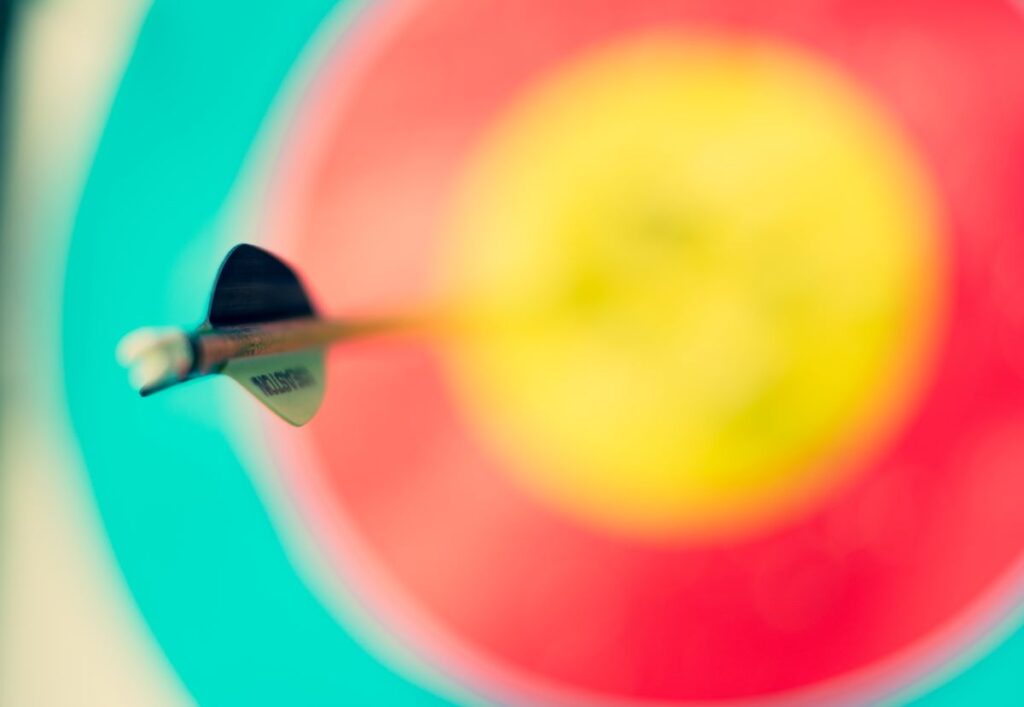
Identifying those at greatest risk following victimisation could help ensure more targeted and personalised care is provided for those most in need of support.
Strengths and limitations
The strengths of this study are that it helps further reinforce that many victims are resilient. The study also demonstrates the importance of studying protective factors across multiple domains, and in separating internalising and externalising disorders. This is vital to ensuring more targeted support.
One drawback of the findings for me lies in the classifications for internalising/externalising disorders. Each represented up to four or five different disorders, meaning predicting the risk of specific disorders is restricted. This may limit the clinical usefulness of the models as different intervention strategies are likely to be necessary for different problems. It would also have been nice to see the study include more protective factors, such as self-esteem, a factor that has been repeatedly identified as predictive of resilience to victimisation (Bowes et al., 2010; Fritz et al., 2018; Sapouna & Wolke, 2013). They also only include protective factors in childhood. While these will inevitably help to inform early intervention, protective factors post-childhood should not be overlooked. Adolescence is a key developmental period, characterised by several physiological and emotional changes that are likely to impact how individuals handle and respond to victimisation.
Finally, as the authors knowledge, by using LASSO regularisation, the individual coefficients from the regression models are limited in their interpretability. This means that it is not possible to infer the exact relationship between the predictor and the outcome. It is likely, however, that resilience to victimisation is governed by many factors, each of small effect. The ability of these models to offer an overall multifactorial risk profile, may therefore actually not be a limitation at all, but may be more suitable than standard linear regression models.
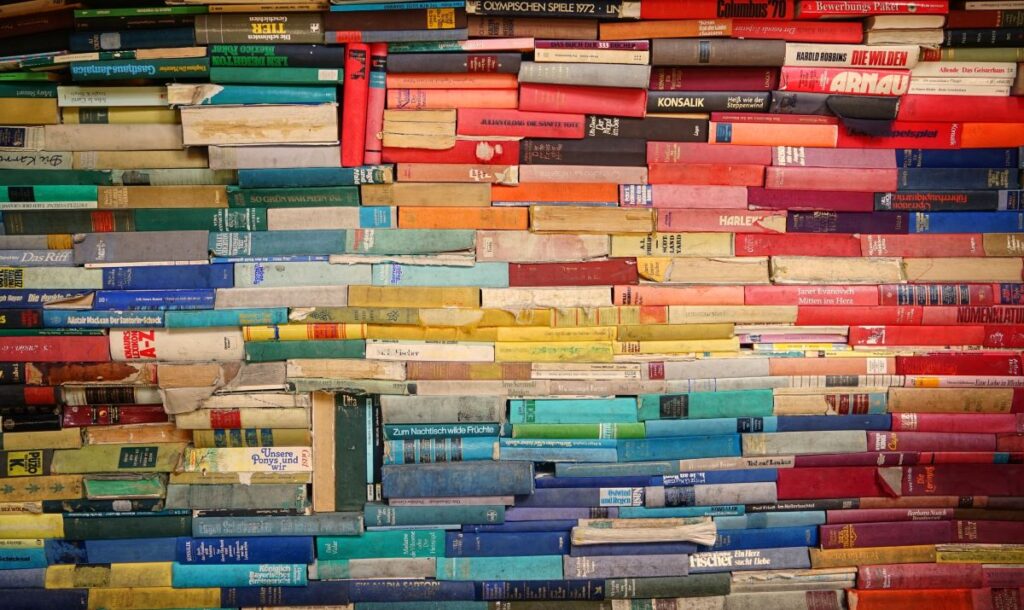
Predicting the risk of specific internalising or externalising disorders is restricted due to studying multiple disorders simultaneously.
Implications for practice
- This study demonstrates that individualised risk prediction models could be used to support more tailored intervention strategies for victimised children.
- This could help ensure that limited resources are appropriately allocated to those most in need.
- The word ‘support’ however, is crucial here as these models should not be used to replace current practices.
- Further external validation is first also necessary before these are considered for use in clinical practice.
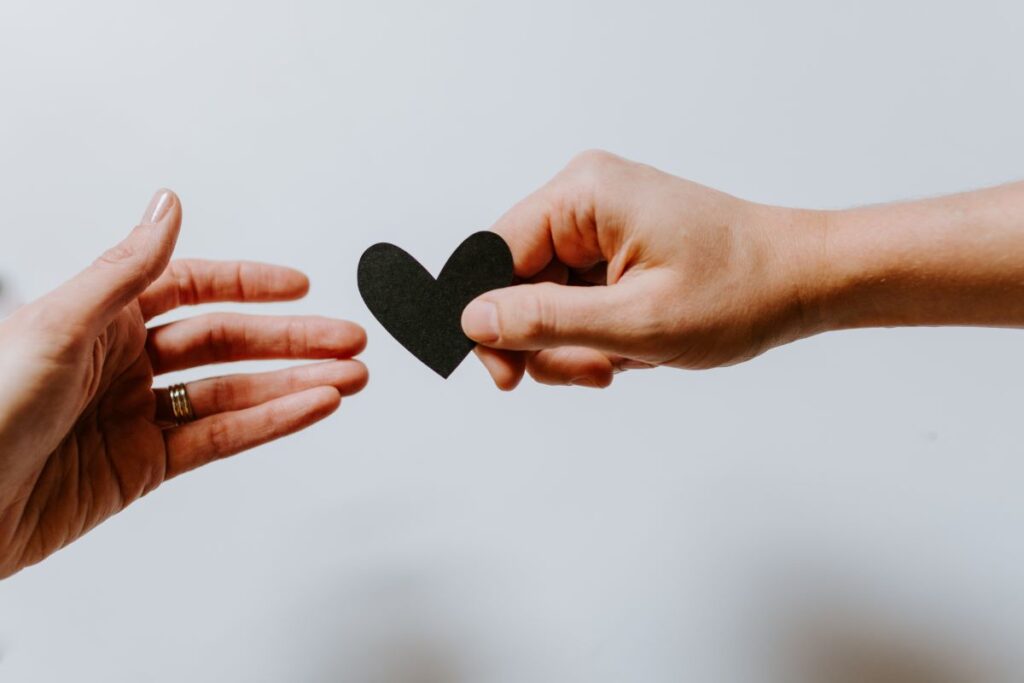
Could individualised risk calculators help ensure that limited resources are appropriately allocated to victimised children who most need help?
Statement of interests
No conflicts of interest.
Links
Primary paper
Meehan, A. J., Latham, R. M., Arseneault, L., Stahl, D., Fisher, H. L., & Danese, A. (2020). Developing an individualized risk calculator for psychopathology among young people victimized during childhood: A population-representative cohort study. Journal of Affective Disorders, 262, 90-98.
Other references
Bowes L. Who gets bullied. Using genetic information to identify individual vulnerabilities. The Mental Elf, 10 Jun 2019.
Bowes L, Joinson C, Wolke D, & Lewis G (2015). Peer victimisation during adolescence and its impact on depression in early adulthood: prospective cohort study in the United Kingdom. BMJ 2015 350 h2469.
Bowes L, Maughan B, Caspi A, Moffitt, TE, & Arseneault L (2010) Families promote emotional and behavioural resilience to bullying: evidence of an environmental effect. J Child Psychol Psychiatry. 2010 51(7):809-817. doi: 10.1111/j.1469-7610.2010.02216.x.
Fritz J, de Graaff AM, Caisley H, van Harmelen AL, & Wilkinson PO. (2018) A Systematic Review of Amenable Resilience Factors That Moderate and/or Mediate the Relationship Between Childhood Adversity and Mental Health in Young People. Front Psychiatry 2018 9:230.
Jackson AM, & Deye K (2015) Aspects of Abuse: Consequences of Childhood Victimization. Curr Probl Pediatr Adolesc Health Care 2015. 45, 86-93.
Hafeman DM, Merranko J, Goldstein TR, Axelson D, Goldstein BI, Monk K, et al. (2017) Assessment of a person-level risk calculator to predict newonset bipolar spectrum disorder in youth at familial risk. JAMA Psychiatry 2017 74, 841-847. doi: 10.1001/jamapsychiatry.2017.1763
McDougall P, & Vaillancourt T (2015) Long-Term Adult Outcomes of Peer Victimization in Childhood and Adolescence. Am Psychol 2015 70(4) 300-310. doi: 10.1037/a0039174
Meng X, Fleury MJ, Xiang YT, Li M, D’Arcy C (2018) Resilience and protective factors among people with a history of child maltreatment: a systematic review. Soc Psychiatry Psychiatr Epidemiol 2018 53, 453-475.
Sapouna M, Wolke D. (2013) Resilience to bullying victimization: the role of individual, family and peer characteristics. Child Abuse Negl. 2013 37(11):997-1006. doi: 10.1016/j.chiabu.2013.05.009.
Photo credits
- Photo by Clayton Robbins on Unsplash
- Photo by Nick Fewings on Unsplash
- Photo by Karim MANJRA on Unsplash
- Photo by Ricardo Arce on Unsplash
- Photo by Robert Anasch on Unsplash
- Photo by Kelly Sikkema on Unsplash