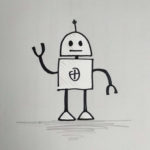
The good news is, we have discovered a range of interventions based on learning positive psychology and cognitive behavioural therapy skills that can help prevent common mental health conditions. These include things like expressing gratitude, practicing kindness, doing things we enjoy, and reminiscing about fond memories. These activities are helpful provided people engage in them consistently. The problem is that getting people to do these things regularly over time is hard; even though they sound like quite pleasant things to do.
Programmes using this sort of intervention are most engaging when delivered on a one to one basis. They are less engaging if delivered as a group and least of all if they are delivered in the form of a book or some other form of self-help. It doesn’t seem to be a matter of one form of delivery being more effective than any other, provided the content is evidence-based. For those who engage, these methods are more or less equally effective. What makes the difference is engagement. Engagement is particularly poor for fully automated systems.
While it would be ideal to provide as many therapists as necessary to ensure perfect engagement, the reality is that everybody could benefit from learning these techniques. We will never have enough trained, completely vetted and supervised therapists to be able to provide this for everyone that needs it. Even if we just focus on those who are unwell, the numbers are staggering. The only scalable solution is to make those automated systems as engaging as possible. This is a problem with which I have been contending for the past 5 years. I spend most of my time designing fully automated interventions and trying to make them as engaging as possible. It is for this reason that I was drawn to a paper by Kien Hoa Ly, Ann-Marie Ly and Gerhard Andersson (Ly et al., 2017).
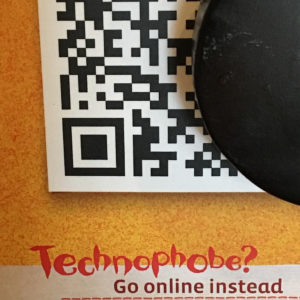
Unsurprisingly, mental health interventions (digital or face-to-face) are most effective when people engage with them. Unfortunately, engagement is particularly poor for fully automated systems.
Chatbots in mental health
The authors describe what they call a ‘fully automated conversational agent for promoting mental well-being’. The study is a randomised controlled trial combined with a qualitative study. Their hypothesis is that reflecting on how having a therapist mainly results in better engagement, it might be that the loss of interactivity and accountability in fully automated programmes that is behind the engagement problem. The authors reflect on the fact that there are commercial conversational agents, which seem to be engaging, such as Cortana, Alexa, Siri and others. However, I am not sure I entirely agree with the authors, as there are no published studies on how engaging these agents really are.
There have been some conversational agents (also commonly known as chatbots) developed for mental health. The first one that reached any notoriety was ELIZA, developed in the mid 1960s at MIT. It did meet with some success in terms of engagement, if not in terms of effectiveness. There have been a few other attempts to use chatbots to improve mental well-being; mainly recruiting participants with no particular diagnosis, but that might be stressed or at risk. I have yet to come across one that showed better engagement than the control group. The authors also review the literature in their introduction and fare no better than I.
The chatbot therapist
Not deterred by that, the authors proceeded to create a conversational agent for mobile phones. The chatbot they created uses the same interface as text messages. They programmed it with a range of predetermined responses to certain keywords.
The chatbot also has a range of scripted conversation trees, where it would make a comment or ask a question of the user and responded according to what the authors anticipated the person might write back. The chatbot is by and large the one who initiates these exchanges. It is programmed to prompt users to show kindness, gratitude, undertake enjoyable activities and reflect on positive experiences. It can give ‘empathic responses based on the user’s mood’, it does a daily checking in, and it can bring up personalised content based on previous exchanges with it. The service also provides a weekly summary of activities and accomplishments.
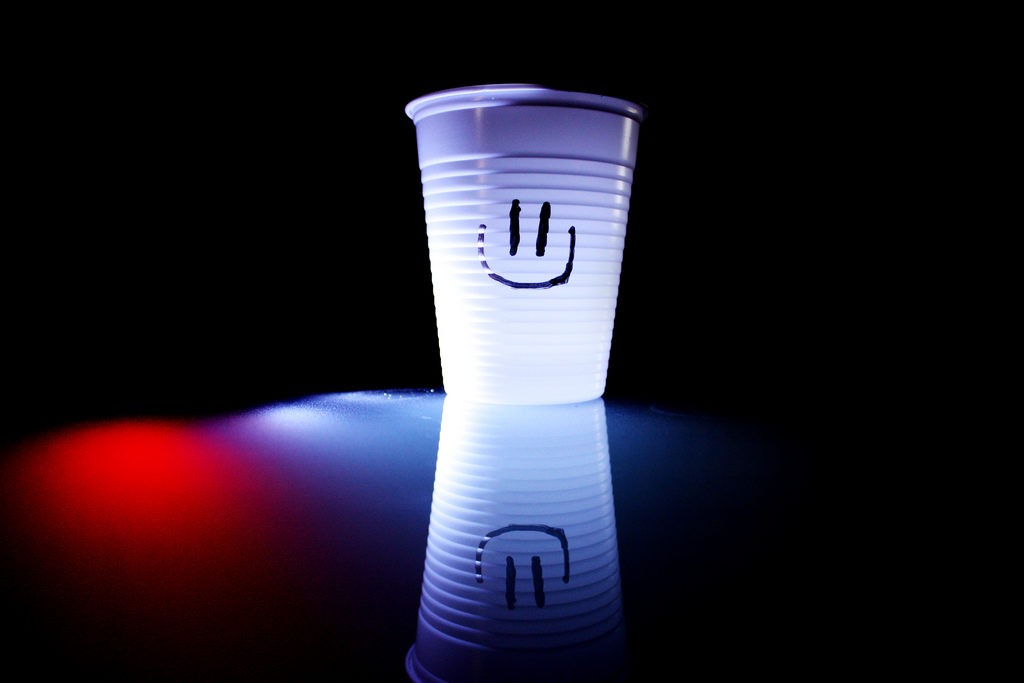
The chatbot in this study gave ‘empathic responses based on the user’s mood’, checked-in every day, and personalised content based on previous exchanges.
Methods
The researchers compared people using the chatbot for 2 weeks with people on a waiting list. They recruited volunteers from universities in Sweden and from Facebook and Twitter. They only included people aged 18 and over who were not in treatment and either not taking any mental health medication or on a stable dose of medication. They included iPhone users only. There are no details as to how many were university students and how many they recruited from social media. They ended up with 36 volunteers of which they excluded 8 because of failure to complete screening, having an active mental health condition and technical problems with the mobile app.
They randomised 14 to either the chatbot or a waitlist control. There is no mention of a power calculation so it is not clear to me if 14 in each arm was enough. This is important as we will see later. A bit more than half of the participants were women and the average age was 26 with a range of 20 to 49.
They used 3 scales as outcome measures. It is not clear which one is their main outcome measure. Two were well-being scales and one was a perceived stress scale.
The qualitative study was done using a semi-structured interview over the phone. Discussions were 30 minutes long. Nine of the 14 people who used the chatbot agreed to the interview.
Results
There was no difference between the groups in any of the outcome measures. The authors did what looks like a post hoc analysis using only the subgroup of those in the intervention group that adhered to the programme. In that subgroup analysis there appeared to be an effect but only in the stress measure. There is no mention of any correction for multiple comparisons, which is particularly relevant given the small numbers particularly in the subgroup analysis. Also, we already know that those who engage are more likely to benefit, so I did not find this subgroup analysis necessary or useful.
More interesting from my point of view were the adherence results. Almost 80% of participants engaged for at least 7 of the 14 days. Participants opened the app a little more than once daily on average. The authors compared their engagement with that of other interventions, and the chatbot seemed to perform well. However, they did not compare its performance with fully automated applications that we know are highly engaging, such as IBM’s Watson in its chatbot version or any successful commercial game.
I found the results of the qualitative study quite informative. The features that users really valued were the weekly summary, the prompts to take specific actions, the fact that it was always available and the invitations to reflect on particular topics. A lot of the users complained that the chatbot was repetitive and machine-like. They encountered some limitations in its ability to process natural language and a lot of them felt they were only able to establish a superficial relationship with the chatbot; they had the feeling that they could go no further with it. Many said that they felt they had to ‘decode’ the chatbot; it was not clear to them what it was all about.
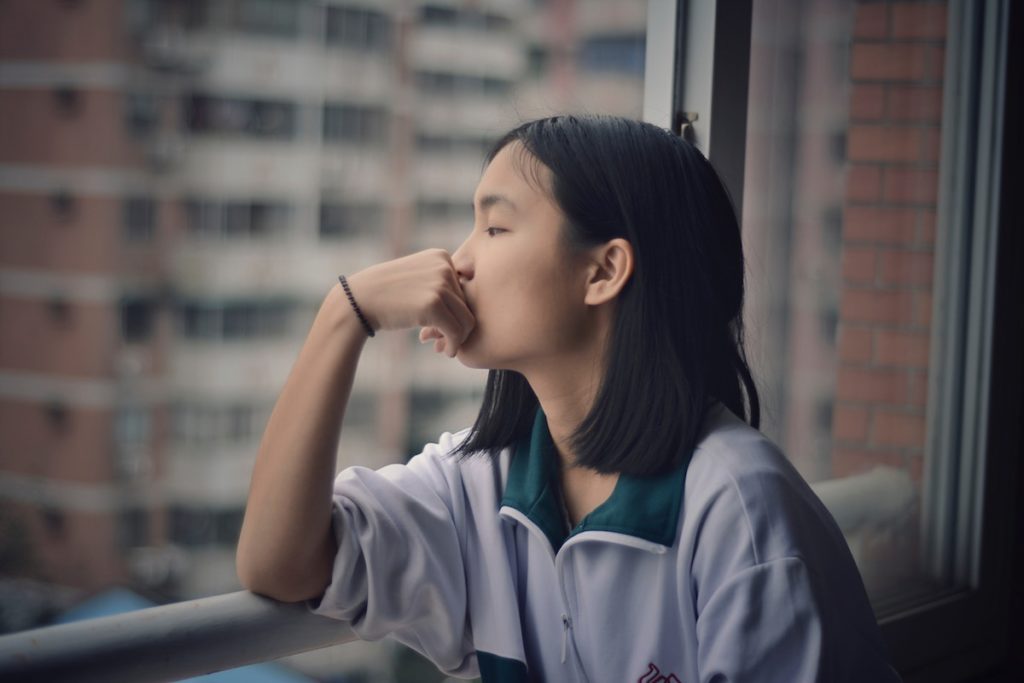
Chatbot features that users really valued were a weekly summary, prompts to take specific actions, 24/7 availability and invitations to reflect on specific topics.
Strengths and limitations
As I said, I am not sure the randomised controlled trial part of this study gave me any insights. It was very small and possibly underpowered and suffering from post-hoc multiple comparisons. Their recruitment methods make it hard to draw conclusions for anyone other than well-educated and affluent young adults; exactly the sort of people for whom access to 1 to 1 therapy is less of an issue.
I found the waitlist control design particularly unhelpful and I am not sure why they wanted to test efficacy, when the key issue they were trying to investigate was engagement. Their design failed to address that completely. It would have been more helpful to compare their chatbot with an already engaging chatbot, such as IBM’s Watson conversation agent or with another fully automated digital intervention that we know is very engaging, such as a mainstream game. Also, I felt that a measure of engagement should have been the main outcome measure.
Having said all this, I believe reading this paper is worth your while for the qualitative study it contains alone. Had I been one of the peer reviewers I would have advised this. It provides valuable insights into what features might help with engagement when designing an automated intervention and what to avoid. All the features mentioned by users are easy to implement and make good sense when trying to generate engagement. It is also very useful to hear that, unless you can make your chatbot fairly sophisticated it might not be the best option to include it. When users become aware that they are talking to a machine it seems to turn them off, possibly leading them to disengage. Those potentially activating and deactivating features are worth studying in more detail. I feel a new study coming on.
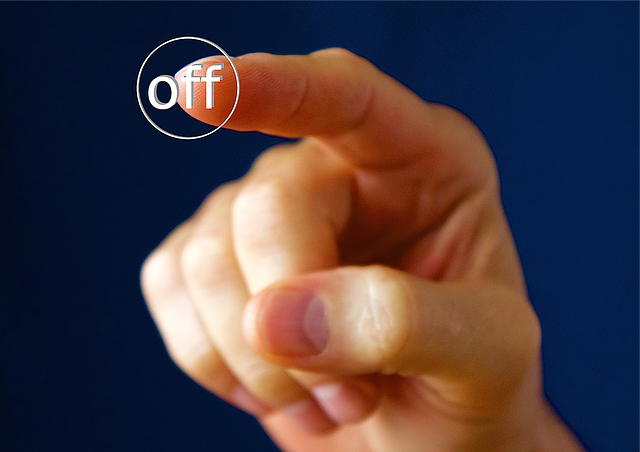
This study suggests that chatbots need to be fairly sophisticated or they might put users off completely.
#Mindtech2017 debate
At 2.15pm today the #MindTech2017 conference will feature a debate on a topic that’s very relevant to this blog: ‘This house believes that AI virtual human (chatbot) therapists can bridge the treatment gap in mental healthcare’. The debate will be chaired by Professor David Daley and panellists will include: Michel Valstar, Alison Faulkner, Elvira Perez Vallejos and Sophie Bostock. You can follow along on Twitter at #Mindtech2017.
Links
Primary paper
Ly KH, Ly AM, Andersson G. (2017) A Fully Automated Conversational Agent for Promoting Mental Well-Being: A pilot RCT using mixed methods. Internet Interventions (2017), doi:10.1016/j.invent.2017.10.002
[…] a re-emergence of the subject with an array of conversational agents for mental health, including a chatbot therapist study covered by Andres Fonseca in an elf blog a few months ago. I myself have spent the last four […]
What are your thoughts on engagement from Woebot’s white paper study done that shows a marked decrease in PHQ-9 measurements compared to the control group.
https://mental.jmir.org/2017/2/e19/