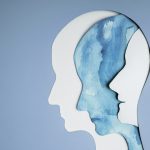
Besides harming themselves or others, being hospitalised is one of the worst trajectories patients can have. Psychiatric hospitalisations are associated with an array of adverse events following discharge, including self-harm, violent offending and victimisation, and early death (Walter et al., 2019). Whilst this is not a causal link – no one would suggest the hospitalisation itself causes these events – avoiding or at least predicting the requirement for admissions is a priority for clinicians.
Tools and studies at our disposal to predict patients’ trajectories either focus on specific diagnoses, certain subgroups of the population, or are based on trial data as opposed to taken from the real-world (Robinson et al, 2019).
That’s where Taquet et al. (2023) come in, by trying to answer whether clinical instability and severity across diagnoses can predict future hospitalisations.
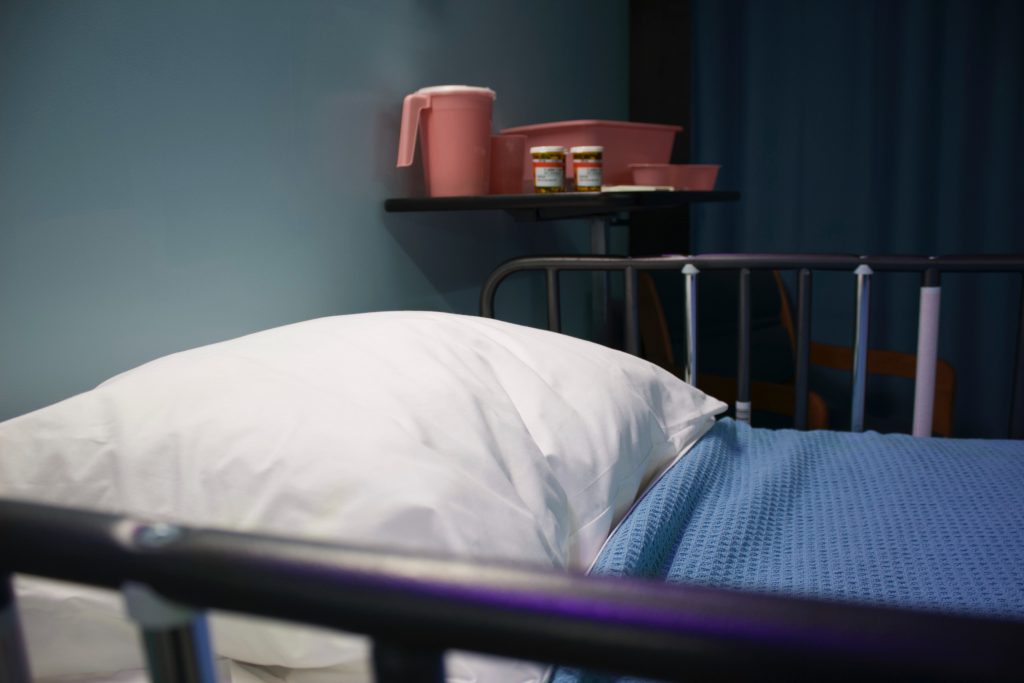
Psychiatric hospitalisation is associated with adverse outcomes, meaning that predicting hospitalisation is a public health priority. However, many current tools to predict future hospitalisations only work for certain diagnoses.
Methods
In this retrospective cohort study, the authors accessed electronic health records from over 36,000 US patients from the NeuroBlu database (Patel et al., 2022) who were diagnosed with a range of psychiatric diagnoses.
These patients came from a network of 25 mental healthcare providers who had their clinical severity assessed using the Clinical Global Impression Severity (CGI-S) measurement (Busner et al., 2007) during a 2-month period (“phenotyping period”). The researchers used a series of CGI-S measurements to calculate clinical severity and an instability score which accounts for varying lengths between CGI-S measurements. They then followed patients up for 6 months to see whether severity and instability were predictive of future hospitalisations.
Analyses were adjusted for age, sex, education, ethnicity, and psychiatric diagnoses, and then these factors plus clinical severity and instability in separate models. Results were presented as hazard ratios (HR).
Results
A total of 36,914 patient records were analysed in this study. Of those patients, 57.3% were female and 55.7% were white, with an average age of 29.7 years and 4.51 years of education. The most prevalent diagnoses were major depressive disorder (MDD; 42.8%), post-traumatic stress disorder (PTSD; 29.7%), attention deficit hyperactivity disorder (ADHD; 25.0%) and bipolar disorder (23.4%), and the median number of CGI-S measurements used to calculate severity and instability was 7 (interquartile range 6-10).
The authors found that:
- For all diagnoses combined (as well as for each of the included diagnoses separately) the risk of hospitalisation was significantly elevated for each standard deviation increase for clinical instability and clinical severity.
- Both measures had a similar effect size when combining all diagnoses, with HRs being 1.09 for instability (95% confidence interval 1.07 to 1.10) and 1.11 for severity (95% CI 1.09 to 1.12) . This was similar for individual diagnoses.
Comparing patients in the top and bottom halves in terms of severity and instability, those in the top half had a 45% increased risk of being hospitalised within 6 months.
- Clinical severity and instability were independent predictors of hospitalisations, and adjusting for the effect of one did not have a big impact on the effects size of the other.
Keeping in mind that not all clinicians use CGI-S measurements, the authors also examined whether using the Patient Health Questionnaire-9 (PHQ-9) to calculate severity and instability would work as well (Kroenke et al., 2001). They concluded that doing so would still significantly predict hospitalisations, despite the smaller number of patients included in this subsample (n = 4,557) and CIs being closer to 1. In addition, the effects were also still statistically significant when adjusting for the number of CGI-S measurements used.
Taquet et al. (2023) attempted to apply those results to a hypothetical example of an intervention to reduce hospitalisations based on the use of severity and clinical instability. In their example, an intervention which reduces the risk of being hospitalised by 80% can be applied to only 250 of 1,000 persons. Those 1,000 persons have a 50% risk of requiring a hospital admission. When applying the intervention to 250 people at random, 100 admissions would be prevented. If this intervention was instead not applied randomly to 250 people, but to those in the top half in terms of both severity and instability, 115 admissions would be prevented. Using this approach would therefore have prevented an additional 15 hospitalisations.
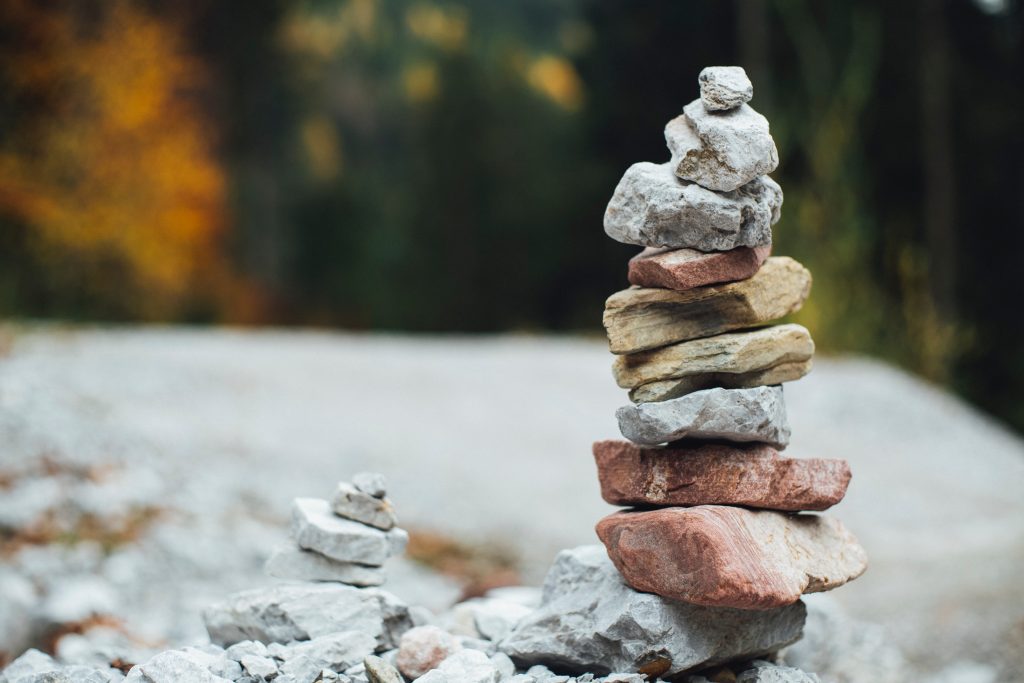
Clinical instability and severity independently predict future psychiatric hospitalisations, irrespective of diagnoses, gender, and age.
Conclusions
Clinical instability and severity can reliably predict future psychiatric hospitalisations, irrespective of diagnoses or other baseline characteristics. There seems to be flexibility regarding whether the CGI-S or PHQ-9 are used, with both being suitable ways to assess patients. Those individuals in the top half of both severity and instability appear to be at the highest risk compared those in the bottom half, which allows clinicians to identify which patients would benefit most from targeted interventions.
Overall, the authors concluded:
This study showed the relevance and effect of clinical instability as a simple and transdiagnostic risk factor, which could be integrated into more elaborate predictive models.
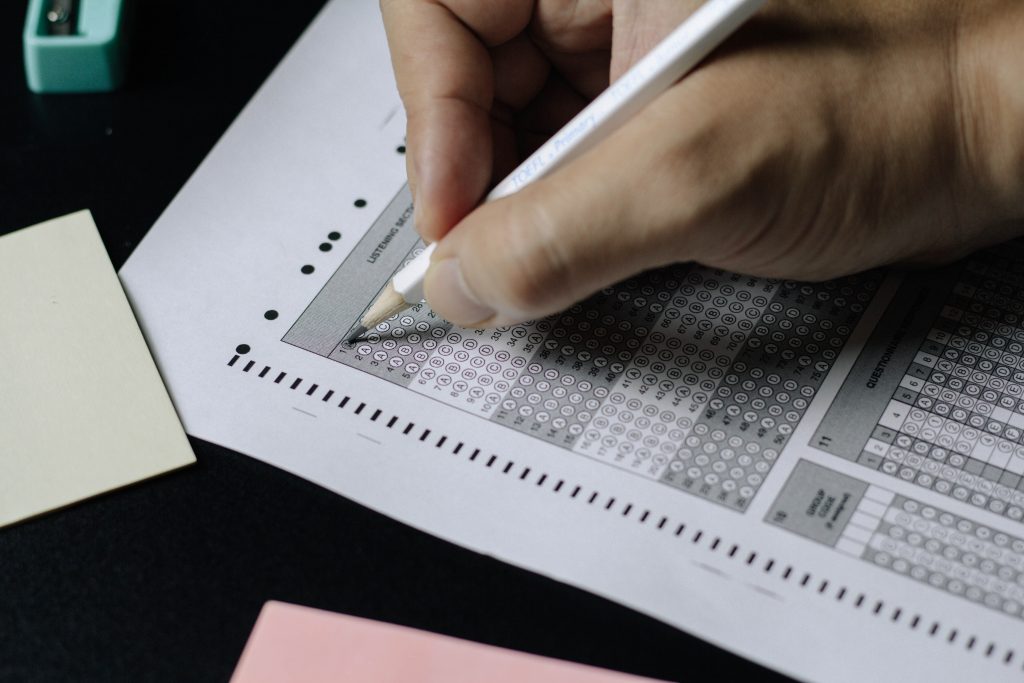
Clinical instability and severity can reliably predict hospitalisations when based on either Clinical Global Impression Severity or the Patient Health Questionnaire-9.
Strengths and limitations
Taquet and colleagues (2023) produced an interesting and well-conducted piece of research, which showcases how to apply sophisticated statistical methods on medical records collected in a real-world setting in addition to presenting clinical applications.
This study comes with a set of strengths, including:
- A sample size of almost 37,000 patients across 25 healthcare providers, adding to the study’s relevance and ability to detect significant differences.
- A range of psychiatric diagnoses, which sets it apart from many other studies which are often limited to specific subgroups of the population.
- Data retrieved from real-world medical records as opposed to trial data, making findings more realistic. Although this study is not free from inclusion and exclusion criteria, the findings appear to be more generalisable than many derived from trials.
- A detailed and transparent description of the methodology. Admittedly, understanding the underlying calculations used to derive clinical instability does require a certain level of statistical knowledge. However, the provided appendix and explanations by the authors are extremely valuable.
- The use of a practical example (the introduction of a hypothetical intervention to show the number of avoided hospitalisations) is great for putting abstract statistical measures into perspective. I wish more authors would use this approach.
However, this study is of course not without its limitations:
- The authors used existing medical records, meaning there is a-trade off between data quantity and the level of detail (as with all studies using similar data).
- This study included clinical information in the form of diagnoses, together with demographic data. However, we don’t know about the treatments people have received prior to their hospitalisations, or details of the nature of their admissions.
- Whilst the study is based on 25 healthcare providers in the US, it is not clear whether results can be generalised to other healthcare settings.
- Whilst the study has suggested an increased risk of hospitalisation among those with high severity and instability, one cannot assume that scoring low equals a low risk in absolute terms.
- It is also not certain whether this study actually measures the association of instability with the risk of hospitalisation, or whether high levels of instability (as well as severity) are a proxy for suboptimal treatment.
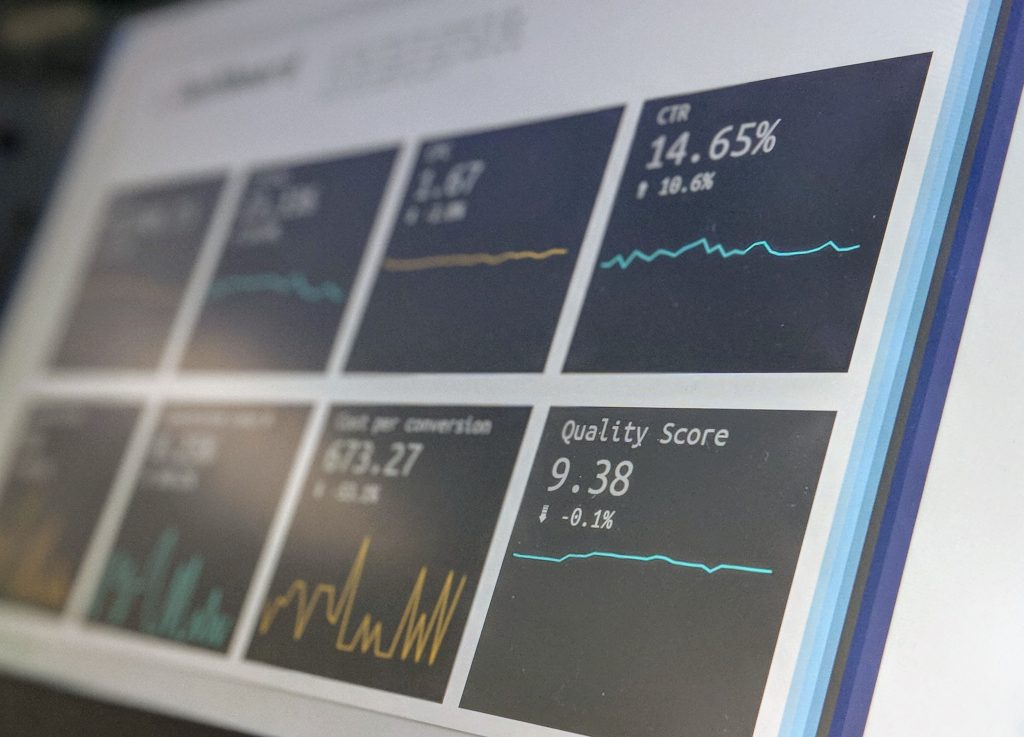
Sophisticated methodology, based on real-world medical records, was used to predict future hospitalisations, likely making the study findings more generalisable than trial data.
Implications for practice
Is measuring clinical instability and severity like a magic recipe that works for all mental health patients? Results from the current study certainly make it seem so, but I think there are two potential applications we need to consider: individual risk predictions and the identification of populations most suitable for interventions.
The study seems to suggest that severity and instability are reliable predictors for future hospitalisations across different diagnoses and demographic factors. Could this be a new approach for clinicians? It is an interesting idea. However, it is unlikely to be the only aspect to consider. Clinicians should include instability and severity in predictive models, as part of a wider, more general framework for predicting individual risks of hospitalisation. In other settings, such as forensic psychiatry, violence risk assessments are a hot topic (Douglas et al., 2017), and many believe they should only be used in conjunction with other information. The same is true for the prediction of future hospitalisations. It might be convenient to have a single metric as predictor, but by relying solely on those we could lose sight of the bigger picture.
Even though it might not be possible to predict individuals’ risks of future hospitalisations, how about identifying groups of patients who might benefit most from certain interventions? After all, we are not considering individuals but larger groups, allowing for less precision. The authors even provide an example of a hypothetical intervention and then calculated the number of avoided hospitalisations. Again, I think this is a valid approach and will certainly help utilising resources to create the most benefit. One caveat to keep in mind though is that calculations like the one provided in this study, rely on a range of assumptions which don’t necessarily apply to other settings. Just as for the prediction of individual risks, severity and instability should be one of many factors in a broader framework.
Overall, I feel that the authors have provided a lot of food for thought. Their novel approach, combining severity and instability in a time series to predict hospitalisations, will add value not only to clinical practice, but future research as well. The highly relevant topic, paired with the sophisticated methodological aspects, makes this a fascinating read for clinicians and researchers.
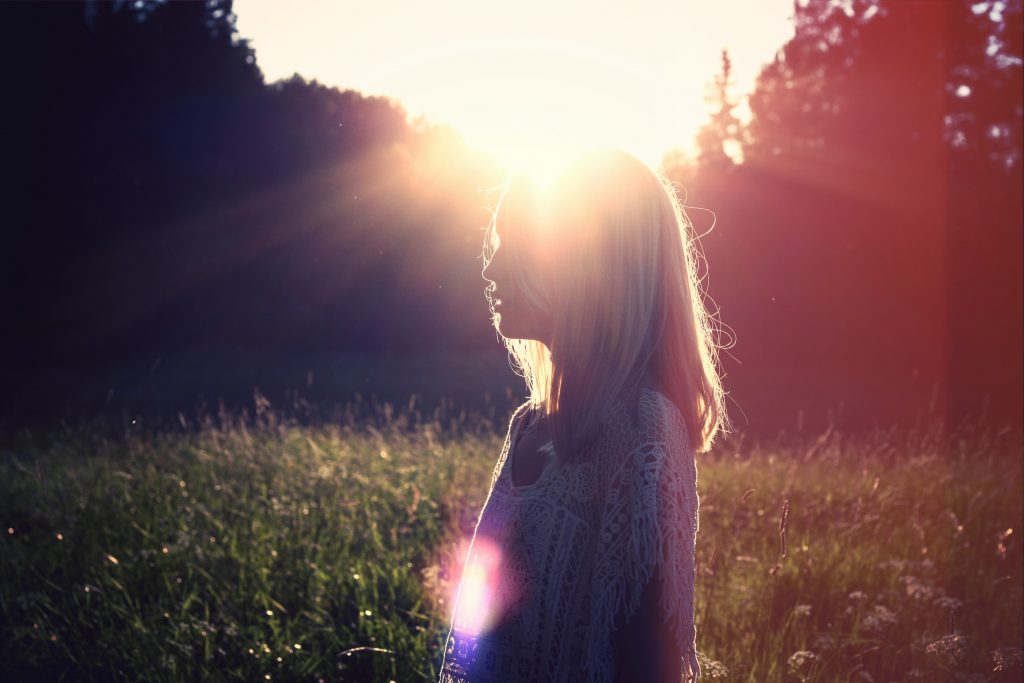
Rather than focusing on individual measures or risk assessments, clinicians should keep the bigger picture in mind, and consider clinical severity and instability in the context of additional factors.
Statement of interests
None to declare.
Links
Primary paper
Taquet, M., Griffiths, K., Palmer, E. O., Ker, S., Liman, C., Wee, S. N., … & Patel, R. (2023). Early trajectory of clinical global impression as a transdiagnostic predictor of psychiatric hospitalisation: a retrospective cohort study. The Lancet Psychiatry, 10(5), 334-341.
Other references
Busner, J., & Targum, S. D. (2007). The clinical global impressions scale: applying a research tool in clinical practice. Psychiatry (Edgmont), 4(7), 28.
Douglas, T., Pugh, J., Singh, I., Savulescu, J., & Fazel, S. (2017). Risk assessment tools in criminal justice and forensic psychiatry: The need for better data. European Psychiatry: The Journal of the Association of European Psychiatrists, 42, 134–137.
Kroenke, K., Spitzer, R. L., & Williams, J. B. (2001). The PHQ-9: validity of a brief depression severity measure. Journal of General Internal Medicine, 16(9), 606–613.
Patel, R., Wee, S. N., Ramaswamy, R., Thadani, S., Tandi, J., Garg, R., Calvanese, N., Valko, M., Rush, A. J., Rentería, M. E., Sarkar, J., & Kollins, S. H. (2022). NeuroBlu, an electronic health record (EHR) trusted research environment (TRE) to support mental healthcare analytics with real-world data. BMJ Open, 12(4), e057227.
Robinson, D. G., Schooler, N. R., Rosenheck, R. A., Lin, H., Sint, K. J., Marcy, P., & Kane, J. M. (2019). Predictors of Hospitalization of Individuals With First-Episode Psychosis: Data From a 2-Year Follow-Up of the RAISE-ETP. Psychiatric Services (Washington, D.C.), 70(7), 569–577.
Walter, F., Carr, M. J., Mok, P. L. H., Antonsen, S., Pedersen, C. B., Appleby, L., Fazel, S., Shaw, J., & Webb, R. T. (2019). Multiple adverse outcomes following first discharge from inpatient psychiatric care: a national cohort study. The Lancet Psychiatry, 6(7), 582–589.
Photo credits
- Photo by American Heritage Chocolate on Unsplash
- Photo by Bret Kavanaugh on Unsplash
- Photo by Markus Spiske on Unsplash
- Photo by Nguyen Dang Hoang Nhu on Unsplash
- Photo by Stephen Dawson on Unsplash
- Photo by Julia Caesar on Unsplash