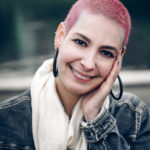
Artificial Intelligence (AI) is the field of computer science dedicated to developing systems that perform cognitive processes characteristic of humans, such as learning, the ability to reason and problem solve, pattern recognition, generalisation, and predictive inference. The development of AI and its increasing application to an array of sectors has brought with it the need to ethically scrutinise and regulate this application.
Mental health care, already a field that by its nature raises particular ethical and legal considerations as well as the need for regulation, has not been untouched by the revolution in digital technology and AI. The field of digital mental health is now firmly established and there is continued and emerging work on AI-driven solutions for mental health (Graham, et al., 2019). Some examples are:
- Chatbots (Vaidyam et al, 2019)
- Personal sensing or digital phenotyping (Mohr et al, 2017)
- Natural language processing of clinical texts and social media content (Calvo et al, 2017)
Thus, we are now at a new intersection point, where AI and data-driven technology meets mental health, which as well as bringing in ethical and legal considerations from its origins, raises its own novel considerations (Gooding, 2019; Klugman, 2017).
A recent editorial piece written by Sarah Carr (Carr, 2020) provides a brief overview and discussion of some of these considerations.
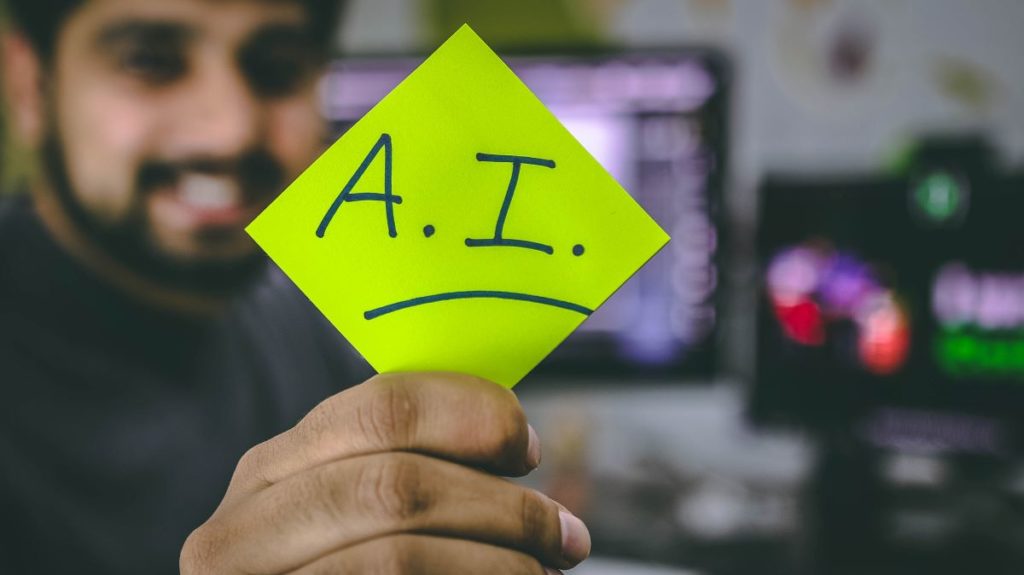
Artificial intelligence is increasingly playing a role in digital health, including mental health.
Methods
The article is a basic editorial piece, which supports its case with a variety of relevant literature references and quotations. Whilst the literature referenced was pertinent, it represents only a subset of relevant material on the matter, which is perfectly fine for an editorial piece to do. Engagement with the literature demonstrated a good understanding of the issue and its context.
Results
Three primary issues discussed in this piece are power, scrutiny and trust. Whilst these are all issues that are being amplified with the application of AI in general, they (particularly power) also have a certain significance in terms of mental health care. The use of power in mental health systems has historically been an issue, and it is important to use ethical frameworks and codes of conduct to ensure that AI is not used to facilitate the problematic exercise of power and authority in service systems and treatment.
A significant general issue in AI applications, particularly with the prevalence of machine learning methods, is bias. Since these AI systems rely on volumes of inputted data to generate their predictive outputs, if the data they are being given is biased, then the outputs are likely to be biased. As noted by Carr, bias is already an issue in human-made mental health decisions unaided by AI, thus we must remain cautious of the potential for AI to exacerbate this problem. We must also ensure that the data being used to drive AI algorithms for early interventions and clinical decision making does not selectively exclude unfavourable data and that it includes as much relevant, truthful data as possible.
The decisions made by AI systems should be regularly scrutinised and machine decisions, ultimately used to inform final human decision making, should be accompanied (where possible) by an explanation; a point endorsed by the emergence of the field of explainable AI, otherwise known as XAI (Schmelzer, 2019; Barredo Arrieta, et al., 2020). Work on algorithmic accountability is also being developed (Shah, 2018; Donovan et al, 2018). These measures will help to foster public trust in AI; in the case of mental health clients, such trust is especially important.
Another issue discussed in this piece concerns patient and public involvement (PPI) in mental health AI research. In the related area of digital mental health interventions (smartphone apps and web platforms), patients can be involved as co-designers of the system and in intervention development. Similarly, the research and development of AI systems for mental health should also in part be shaped by questions involving patient stakeholders. Further to this, it is important to recognise that predictive mental health AI systems are driven by the personal data of patients, and that these patients must be informed of and consent to the nature (generally to some extent privacy sensitive) of this data collection. Certain AI ethics guidelines emphasise the need to pay particular attention to applying AI to vulnerable and disadvantaged groups and that such individuals should be included in the development, deployment and use of AI systems. This suggests the need to prioritise the involvement of patients in mental health AI research.
Finally, there is a case to be made for positioning the patient, carer or service user as a “domain expert”. In AI, a domain expert is someone with special knowledge or skills in the topic being analysed or modelled. Their contribution is to provide meaningful context and data interpretation based on their expertise in the topic. For example, a financial expert might provide some rules of thumb in developing a financial prediction system and then help to give raw data results a real-world interpretation. Whilst mental health patients or those with lived experience might not possess this type of technical expertise, their involvement as domain experts is supported by the successful involvement in other situations of “domain experts” with localised or experiential knowledge in interpreting data and creating solutions.
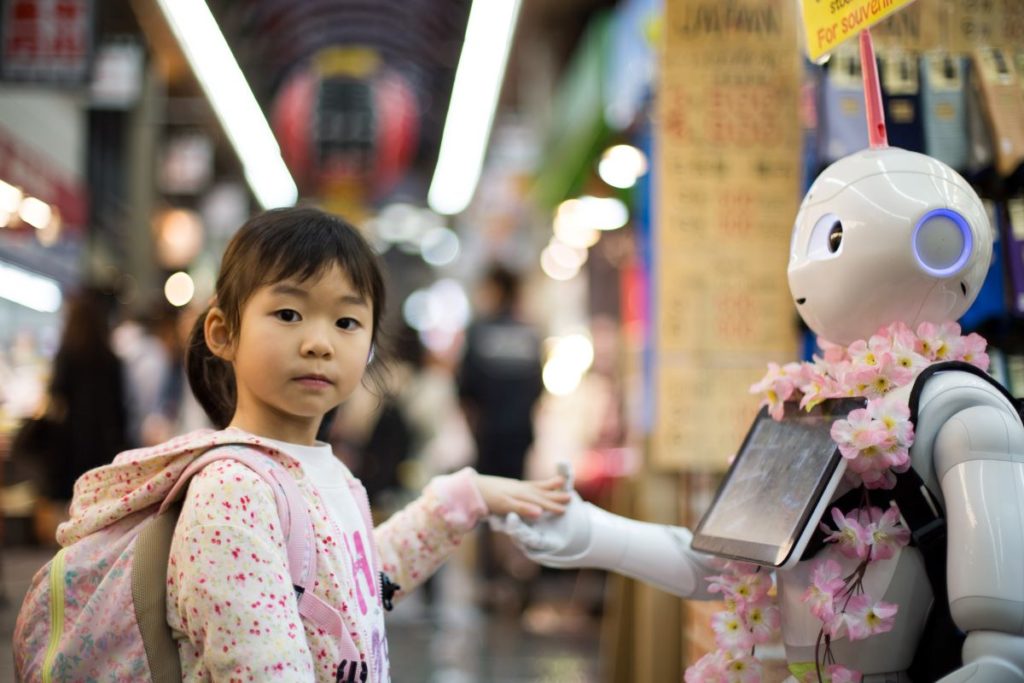
The human connection must remain central in AI mental health research, with concerns such as patient trust and involvement paramount.
Conclusions
Despite mental health professionals, politicians and tech companies increasingly seeing AI and data-driven technologies as having a significant role to play in mental health treatment and care, it appears, as Carr notes, that key stakeholders are currently excluded from the conversation on AI and mental health. Without scrutiny, transparency and algorithmic accountability there are risks of creating or worsening inequalities and problematic power structures. As the author concludes:
If AI is to be increasingly used in the treatment and care of people with mental health problems then patients, service users and carers should participate as experts in its design, research and development. Their data will be used to train and drive many of the AI applications designed for predictive modelling, to inform clinical decisions and to determine the timing and types of intervention.
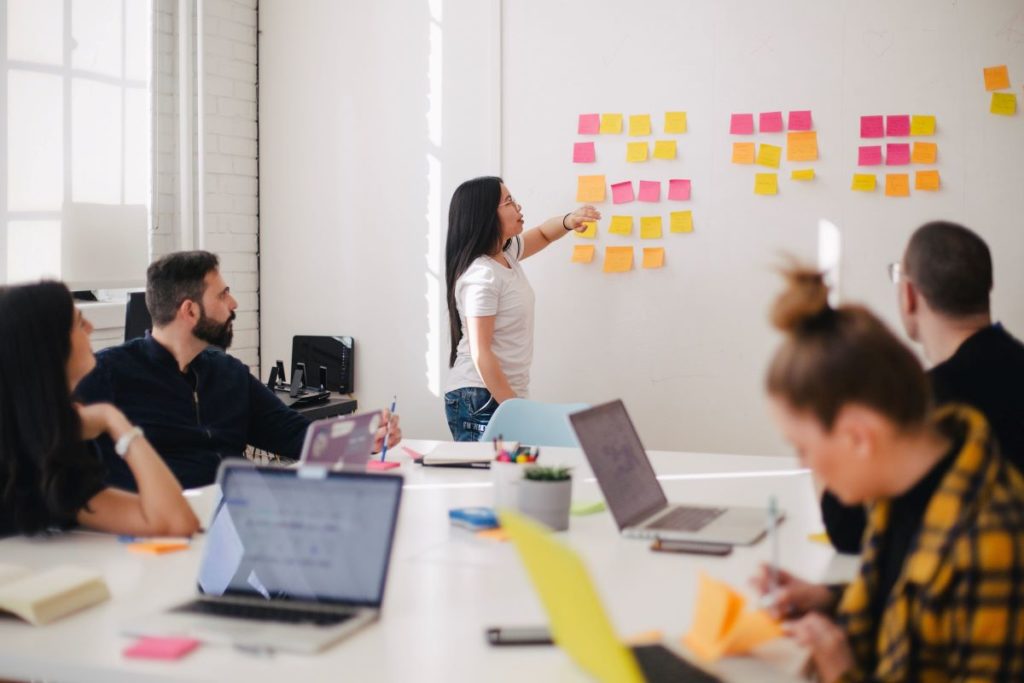
Patients, service users and carers should participate as experts in the design, research and development of AI mental health solutions.
Strengths and limitations
The various points made throughout this editorial piece are in general sound and reasonable. The discussion of power, trust and scrutiny resonates with an established awareness of the need to consider these ethical dimensions of AI research and applications. Some of these concerns may be particularly relevant for mental health applications, given historical problems in mental health treatment and the vulnerability of those with mental health issues.
The positioning of the mental health patient as a “domain expert” is particularly interesting, though on this matter this piece does have some limitations. One issue is that there is no consideration or mention of AI and mental health cases that do involve patient and public involvement; there are certainly counter examples to the generalisation that “key stakeholders are currently excluded from the discussions about AI in mental health”.
I can think of some examples of PPI in my own AI mental health research. For example, with a smartphone digital phenotyping study currently in progress, we initially consulted with a focus group of young people with lived experience during the design phase of the study, asking them for feedback on the data we intended to collect and how it would be used. Furthermore, each participant in the trial will be given an information sheet on the study, including technical aspects, and they have complete control over what they do and don’t share in terms of tracked sensors. Finally, upon their completion of the trial, participants have the opportunity to receive data insights we capture about them.
Another point for consideration concerns potential tensions between AI and data-driven predictions and domain expertise in the PPI sense. The author writes that “AI research and development for mental health care cannot continue without full PPI and full participation in research”. But what exactly is “full participation” and where, if at all, is the line drawn between the inclusion of PPI and the exclusion of PPI?
In developing AI algorithms or models, clearly there are technical decisions to be made by those with AI expertise, decisions that should not be influenced by patients without the required technical expertise. This much might be obvious. However, another point (admittedly somewhat philosophical and tangential) also comes to mind, regarding potential tensions between AI and data-driven outcomes and patient reports or beliefs. Take the following example, which although somewhat simplistic, suffices to convey the overarching point. Suppose that an AI analysis of assorted data collected from an individual’s ‘digital exhaust’ (data gathered from their digital device and social media interactions) determined with great predictive certainty that they were in a prodromal stage of psychotic illness. The individual themselves doesn’t feel that anything is amiss and there are no manifest signs; rather, the AI analysis picks up on some problematic patterns that can only be feasibly ascertained via algorithmic data analysis. Though the individual is notified of this detection and informed of the possibility of beneficial early intervention, they choose not to receive treatment because going by their experience nothing feels wrong.
Once again, this is a simplistic example, but it serves to make a point: if AI and data could truly tell us things about ourselves before we are even aware of them, then what is the outcome when an AI, data-driven prediction is contrary to the individual’s present experience or belief? In one important sense the patient has the right to exercise their freedom, though in this case the “domain expertise” of present subjective experience would be contrary to an objective AI prediction with high accuracy.
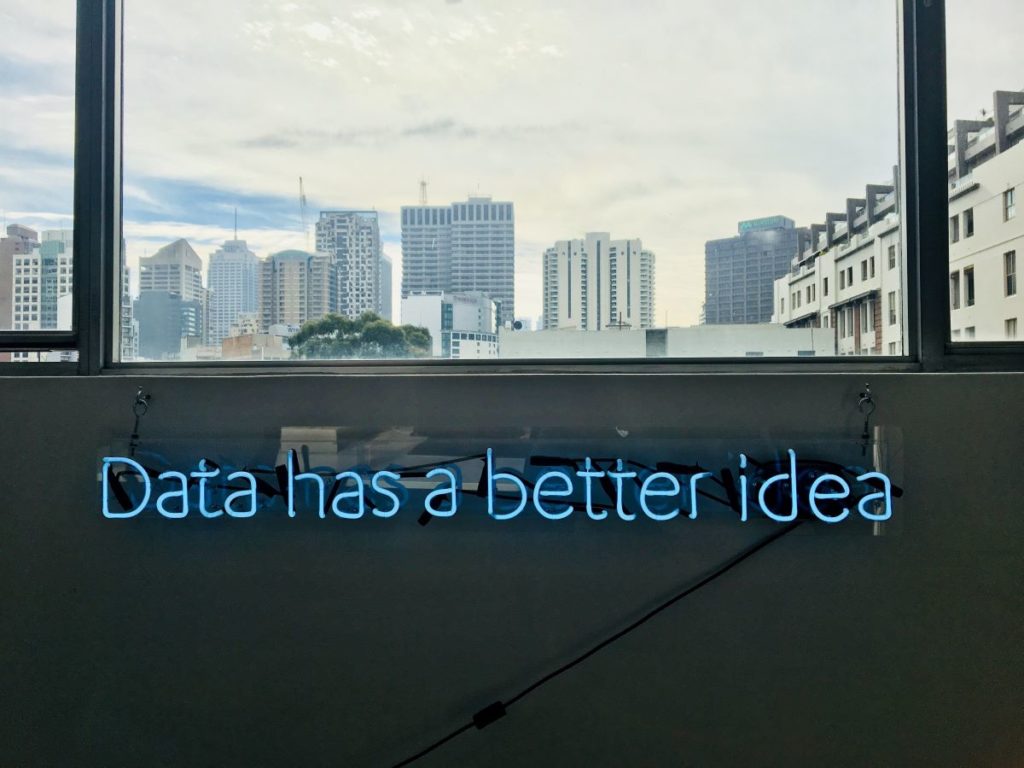
What if AI and data could serve as a ‘crystal ball’ and predict imminent mental health issues?
Implications for practice
This editorial piece raises a range of interesting points that should be considered by those working on applying AI to mental health. Furthermore, those working in this area should employ practices that align with the proposal in this paper to involve patients, service users and carers as domain experts in AI mental health research. Following are some suggestions:
- Involve patients and the public in discussions about the ethical use of AI in mental health
- Consider responsible AI principles such as the following when conducting AI mental health research and development: Fairness, Inclusiveness, Reliability & Safety, Transparency and explainability, Privacy & Security, Accountability (Microsoft, 2020)
- Ensure that those whose data is being analysed are aware of and provide informed consent to the tracking and analysis of their data
- When developing AI methods, consult with these domain experts to get their feedback on what data is being collected and for what objective or inferential purposes it is being used
- Particularly in research that explores the associations between data patterns and behavioural patterns or mental states, consult with study subjects and potentially their carers and clinicians to get their perspectives on what inferences have been made about them using the data.
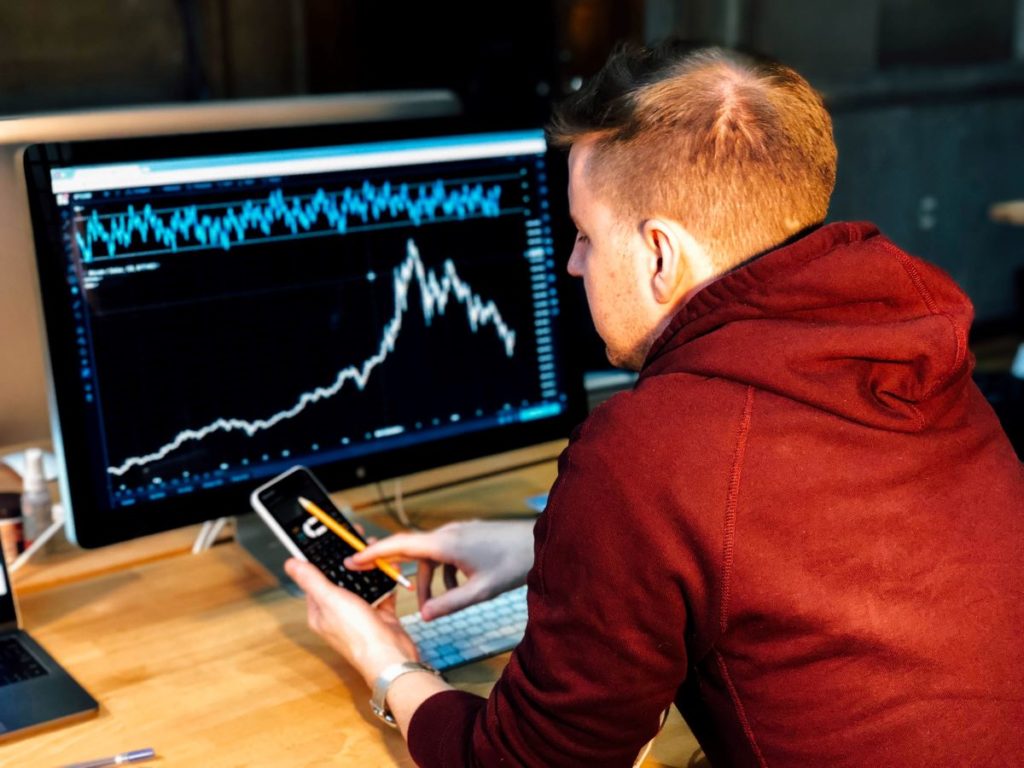
Patients, service users and carers should be involved as domain experts in AI mental health research, including the interpretation of personal research results.
Statement of interests
Simon D’Alfonso leads the Digital Technology and Artificial Intelligence for Mental Health research project at the University of Melbourne.
Links
Primary paper
Carr, S. (2020). ‘AI gone mental’: engagement and ethics in data-driven technology for mental health. Journal of Mental Health. doi:10.1080/09638237.2020.1714011
Other references
Barredo Arrieta, A., Díaz-Rodríguez, N., Del Ser, J., Bennetot, A., Tabik, S., Barbado, A., . . . Herrera, F. (2020). Explainable Artificial Intelligence (XAI): Concepts, taxonomies, opportunities and challenges toward responsible AI. Information Fusion, 58, 82-115. doi:10.1016/j.inffus.2019.12.012
Calvo, R., Milne, D., Hussain, S., & Christensen, H. (2017). Natural language processing in mental health applications using non-clinical texts. Natural Language Engineering, 1-37. doi:10.1017/S1351324916000383
Donovan, J., Caplan, R., Matthews, J., & Hanson, L. (2018, April 18). Algorithmic Accountability: A Primer. Retrieved February 24, 2020, from Data & Society: https://datasociety.net/library/algorithmic-accountability-a-primer/
Gooding, P. (2019). Mapping the rise of digital mental health technologies: Emerging issues for law and society, 67. doi:10.1016/j.ijlp.2019.101498
Graham, S., Depp, C., Lee, E. E., Nebeker, C., Tu, X., Kim, H.-C., & Jeste, D. V. (2019). Artificial Intelligence for Mental Health and Mental Illnesses: an Overview. Current Psychiatry Reports, 21(116). doi:10.1007/s11920-019-1094-0
Klugman, C. M. (2017, August 8). Care for your Mind. Retrieved February 24, 2020, from The Ethics of Digital Mental Health: https://careforyourmind.org/the-ethics-of-digital-mental-health/
Microsoft. (2020, February 25). Responsible AI principles from Microsoft. Retrieved from Microsoft: https://www.microsoft.com/en-us/ai/responsible-ai
Mohr, D. C., Zhang, M., & Schueller, S. M. (2017). Personal Sensing: Understanding Mental Health Using Ubiquitous Sensors and Machine Learning. Annual Review of Clinical Psychology, 13, 23-47. doi:10.1146/annurev-clinpsy-032816-044949
Schmelzer, R. (2019, July 23). Understanding Explainable AI. Retrieved February 24, 2020, from Forbes: https://www.forbes.com/sites/cognitiveworld/2019/07/23/understanding-explainable-ai/#68fc333c7c9e
Shah, H. (2018). Algorithmic accountability. Philosophical Transactions of the Royal Society A, 376(2128). doi:10.1098/rsta.2017.0362
Vaidyam, A., Wisniewski, H., Halamka, J., Kashavan, M., & Torous, J. (2019). Chatbots and Conversational Agents in Mental Health: A Review of the Psychiatric Landscape. The Canadian Journal of Psychiatry, 64(7). doi:10.1177/0706743719828977
Photo credits
- Photo by Drew Graham on Unsplash
- Photo by Hitesh Choudhary on Unsplash
- Photo by Andy Kelly on Unsplash
- Photo by You X Ventures on Unsplash
- Photo by Franki Chamaki on Unsplash
- Photo by Chris Liverani on Unsplash
I wonder how useful the idea of a domain expert is when considering knowledge (see Shadbolt and Smart 2015) when we consider the wide range of different kinds of knowledge, for example tacit knowledge.