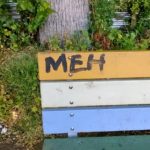
A new study looking at genetic traits for cardiovascular health and the associated risk for mental health disorders has evaluated the link between obesity and an individual’s perception of health (Wootten et al, 2018).
This recent work is the latest in a plethora of ‘mendelian randomisation studies’ designed to investigate the relationship between genes and downstream health risk and to provide new treatment avenues (Smith et al, 2008).
By linking genotype to clinical symptoms, researchers can delineate causal links with less compensation for confounding variables present in observational studies. Put simply, no action a participant can take will change the genes they inherit. This nullifies any ‘reverse causality’, a real issue in cementing true links between actions and results (Smith, 2016.)
Such studies, including Genome Wide Association Studies (GWAS), reveal causal associations (i.e. direct risk from inheritance of a gene) which could be for more than one outcome (higher risk of stroke alone) or more (higher risk of stroke and diabetes). One such interest area is mental health and its links to physical traits, a subject predominating social arguments of health.
Obesity is independently linked with a variety of medical problems, ranging from the physical to mental, both as a cause (Ogunbode et al, 2011) and a result (Hruby et al, 2015). We also know that a higher level of ‘subjective wellbeing’ (how we feel about our health) is linked with better health outcomes (Steptoe et al, 2014). What is less clear is the casual link between weight and mental health, whether a change in body weight precipitates psychiatric disease or indeed is a result.
Although data can be found to support both increases or decreases in weight in mental health disorder (Jung et al, 2017) there is strong evidence that obesity can worsen depressive symptoms and a suggestion that a decreasing BMI can lead to better ‘subjective’ view of health (van de Broek et al, 2018). However, until now, the strength for this latter conclusion has been relatively underwhelming.
In this recent study (Wootten et al, 2018), the research team looked at data gathered on many participants included in GWAS (n= 83,198 – 332,224), evaluating measures of physical health and then following up observations for a significant proportion of patients on the UK Biobank (n= 331,112.) Measures of several metrics for cardiovascular risk were compared with personal accounts of wellbeing and then subjected to complex analysis to determine any causal links.
The data showed that although there was no direct link between a person’s perspective of their health and their ‘cardiometabolic’ health outcomes, there was a direct link between poor cardiometabolic health lowering subjective wellbeing, within the sole domain of body mass. Put simply, the higher the weight, the lower the perception of wellbeing.
Although this new work does provide us a strong link between weight and health satisfaction, the nature of the link may still be up for interpretation, whether due to social perceptions of health or indeed the presence of co-morbid physical health issues.
However, the causal link between genes and outcome is strong and provides us with a clear-cut risk. And when related to previous data, suggesting that high BMI worsens depressive symptoms, regardless of the nuance of determinant, we can say that obesity can make us feel worse and risks more severe psychiatric disease.
Although contentious, this may provide us with further cause to tackle obesity and raises serious questions about ‘feeling healthy at any weight’, but how this is translated to clinical practice is a delicate subject.
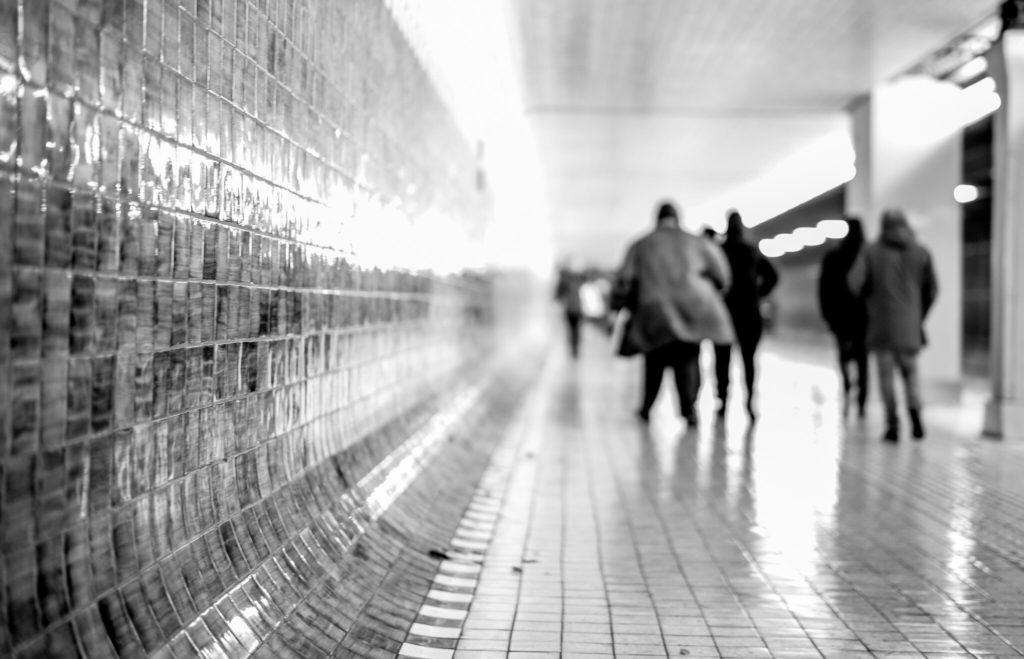
Genomic data links obesity to poor health satisfaction and possible treatment avenues.
Methods
Wootten et al (2018) used a large cohort of participants (n= 83,198 – 332,224) gleaned from multiple GWAS to investigate the link between inherited risks to cardiometabolic disease, which they then compared to the participants view of their own health. This data was also reviewed with respect to presence of genes linked with risk, gender proportion and area of ancestry (European or non-European).
Data taken at baseline of ‘subjective wellbeing’ measured a quantitative (numerical) representation of several domains, including happiness, family and relationships, work, health and finances. Responses were coded in a Likert Scale (range 1-6, running from ‘Extremely Unhappy to Extremely Happy’) with omissions made for ‘I do not know’ of ‘prefer not to answer.’
Measurements of cardiovascular factors taken from across multiple studies included blood pressure, BMI, body fat percentage, presence of heart attack and cholesterol levels. Baseline socioeconomic factors, level of education, alcohol and cigarette use were also compared with outcomes.
To further test the link between BMI and wellbeing, the authors formulated a polygenic score comprised of the 97 genetic variants associated with BMI and compared a weighted score (calculated as the sum of increasing allele number) and compared this with subjective wellbeing scores.
Initial links were found within multiple GWAS samples and then reviewed with follow up analysis with UK Biobank data (n= 337,112) to reduce the risk of horizontal pleiotropy (a severe confounding factor for mendelian randomisation (Verbank et al, 2018.)) The mean age of participants was 56.87 years with a female predominance of 54%.
Data was subjected to complex statistical analysis to ensure significance of causal links and reduce the risk of horizontal pleiotropy. Linear regression was used to generate observational associations, data subjection to mendelian randomisation and extraneous variables such as age and gender were controlled.
Results
Data showed that there was no causal link between most measures of cardiovascular risk and genotype with subjective wellbeing score, or indeed with wellbeing score predicting subsequent risk. There was no strong evidence for measures such as blood pressure and cholesterol levels.
However, the study did show that within the sole domain of obesity and defined by a novel polygenic scale, that presence of obesity directly led to reduced scores of subjective wellbeing with BMI linked to ‘reduced health satisfaction’ (p<0.001). This data was persistent regardless of age and sex.
Evidence was also found for the body mass index genetic score and socioeconomic position, educational attainment, smoking behaviour and alcohol consumption. However, for socioeconomic position and educational attainment, the association was weaker for the genetic score than gross observed BMI alone.
Associations with subjective wellbeing were limited to ‘health’ alone and did not extend significantly to other domains such as ‘family and relationships.’
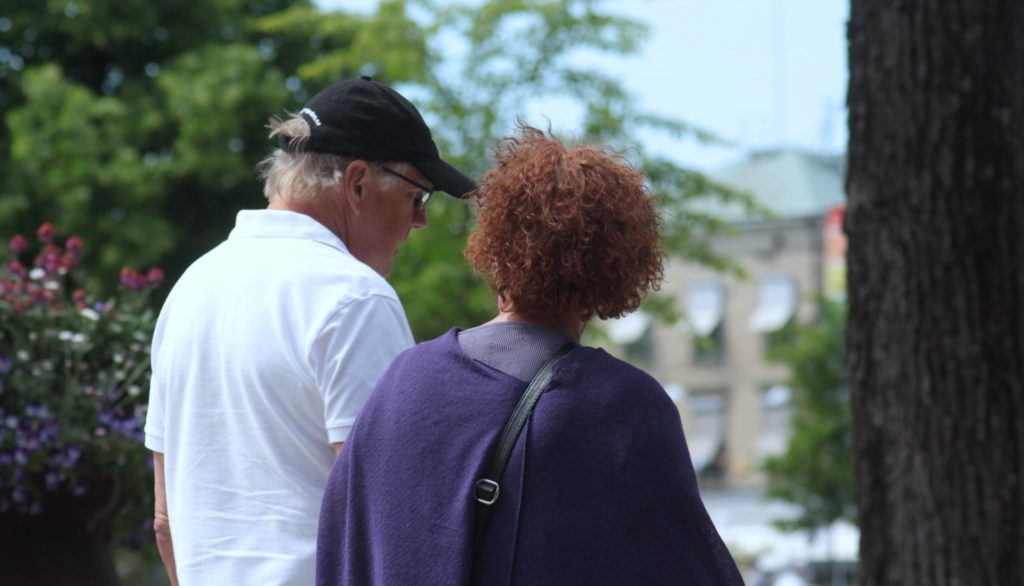
According to this evidence, obesity drives reduced health satisfaction, regardless of age and sex.
Conclusions
The authors concluded, rather conservatively, that a high BMI predicts a lower subjective score of health, that is supported experimentally to high level of confidence using novel polygenic scores and is relatively absent of confounding variables. It is also fairly generalisable across age and sex.
This data is coherent with previous research in the field and reflects a contentious social memetic that obesity is linked with poor health perception and higher risk of severe depressive symptoms.
They also suggest that the mode of perception could be influenced by social factors (i.e. a societal stigmatisation of obesity), or indeed the presence of actual health problems associated with it leading to chronic disease. Both are fair explanations with a logical discourse, but the relative influence of either is not defined and requires further work.
The authors also signpost the influence of age, with a higher median age (57) possibly factorial in perception of health, which arguably may present a lower estimate based on individual experience. They suggest that this is a further area of research, where different ages may present a different profile in cause of dissatisfaction (i.e. social factors predominating in younger age groups, disease in older)
This data presents an experimentally strong case for obesity predicting lower health satisfaction and inferred validation of previous work linking obesity with more severe depressive symptoms.
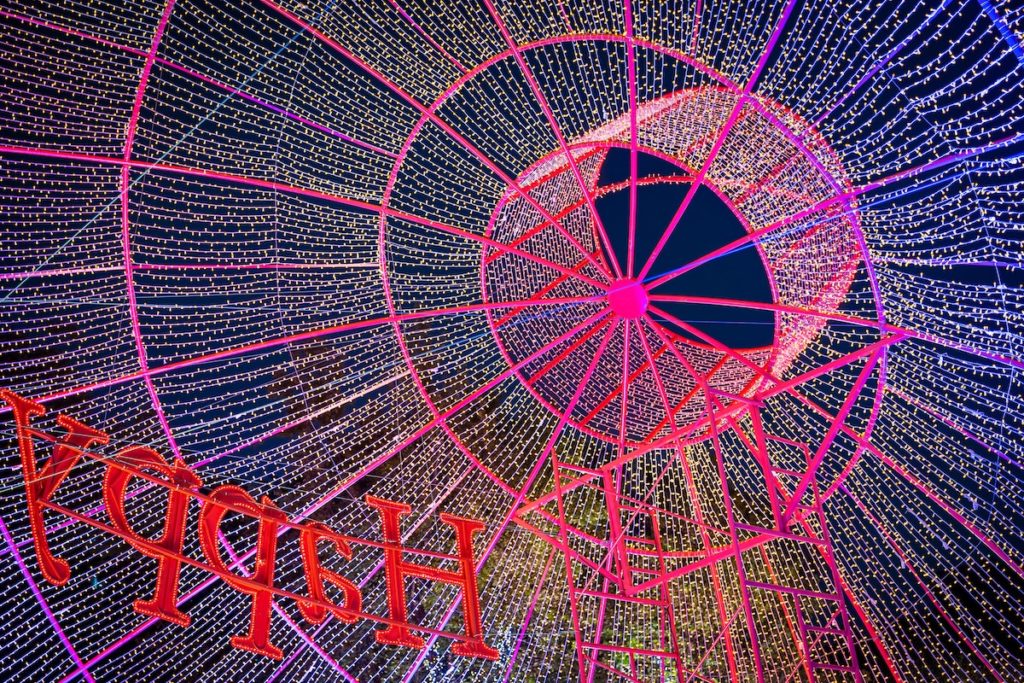
The nuance of unhappiness may differ by age, but genomic data predicts a pattern.
Strengths and limitations
The study’s primary strength lies in its use of mendelian randomisation and use of GWAS to instill confidence in non-reversible causal links between genotype and clinical correlate. This is a well known and supported novel research method that has taken observational knowledge and cemented it in more accurate and robust analysis.
The authors have utilised a large sample size, provided rigorous statistical intervention to reduce and account for confounding variables, adjusted for baseline socio-economic and societal metrics, sex and age. This has led to the production of a data set that has a high versatility in establishing causal links, but provides little further qualitative information on the aetiological nuance of these links. However, this was not the purpose of the study and has been recognised by the authors.
The pressing weakness of the study is arguably the use of subjective wellbeing scores taken at time and place. These scores, taken on a restricted range of domains and data points, means that the results are quite general and unspecific, applicable only at the point of collection and susceptible to variation in participant mood and perception, which in depressive states may be over critical and not represent the natural progression and change of mood over time.
However, given the large sample size, it is likely that, given a heterogenous range of presentations at baseline, any skew to data would be cancelled out. Still, pause should be taken in generalisation of such amalgamated data as patients are individuals and do not wholly represent a statistical archetype.
A second weakness is the use of BMI as a measure of obesity, which is well known to be inaccurate (Romero-Correl et al, 2008.) However, once again due to the large sample size, any atypical changes (such as high muscle mass) are likely adjusted for. The use of the polygenic scale seems to reduce the risk of confounding inaccuracy more, but relies on the acceptance of allele number as a determinant of risk (additive modelling (Elston et al, 2012). However, this is generally conferred to be both predictive and number-related, with the presence and increased number of alleles present leading to a stronger phenotype expression.
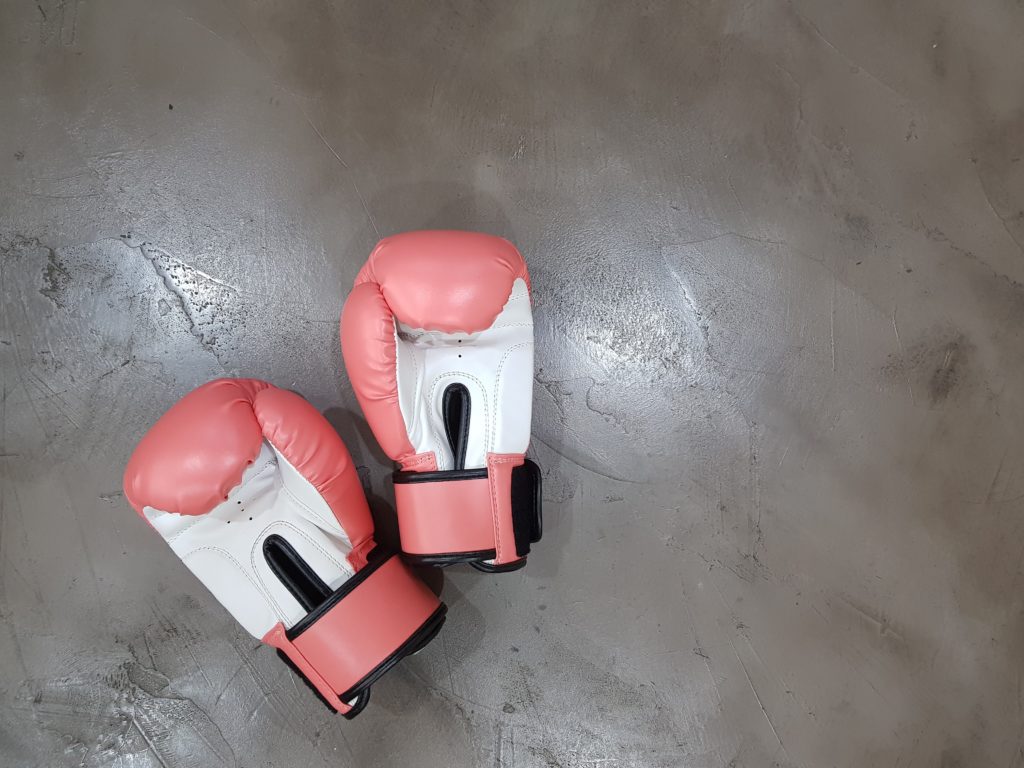
Genetic studies present us with strong data, but little explanation.
Implications for practice
Although the study was not able to usefully link subjective wellbeing as a predictor of poor health outcomes, it did show us that a lower health satisfaction is a reliable indicator of higher BMI and polygenic BMI score. This, taken carefully, could present an opportunity to direct treatment avenues, especially in the context where obesity is linked to worse health outcomes, including depression.
Given recent research into the use of exercise programs showing limited efficacy beyond early and heavily monitored intervention (Janaway, Mental Elf, 2018) in terms of physical risk, there was marked subjective improvement in patient experience with such programs. This was limited to presentations with psychosis, which likely present a lower attainment rate for such programs and arguably present consideration of greater uptake in less severe disease without psychosis.
This, alongside the conclusions drawn from this study, suggest that reduction of BMI could lead to better subjective wellbeing and improved psychiatric outcomes if utilised within sustainable diet and exercise programs. However, I would advise that antecedent research be carried out to explore specific environmental and social factors involved in obesity before designing such programs.
However, this may be met with some consternation around identity and politics, where the conclusion that being overweight leads to unhappiness will result in some reasonable backlash. As such, a patient-centred, non-judgmental and supportive approach will need to be taken with consideration of public consortium or consultation with patient advocacy groups.
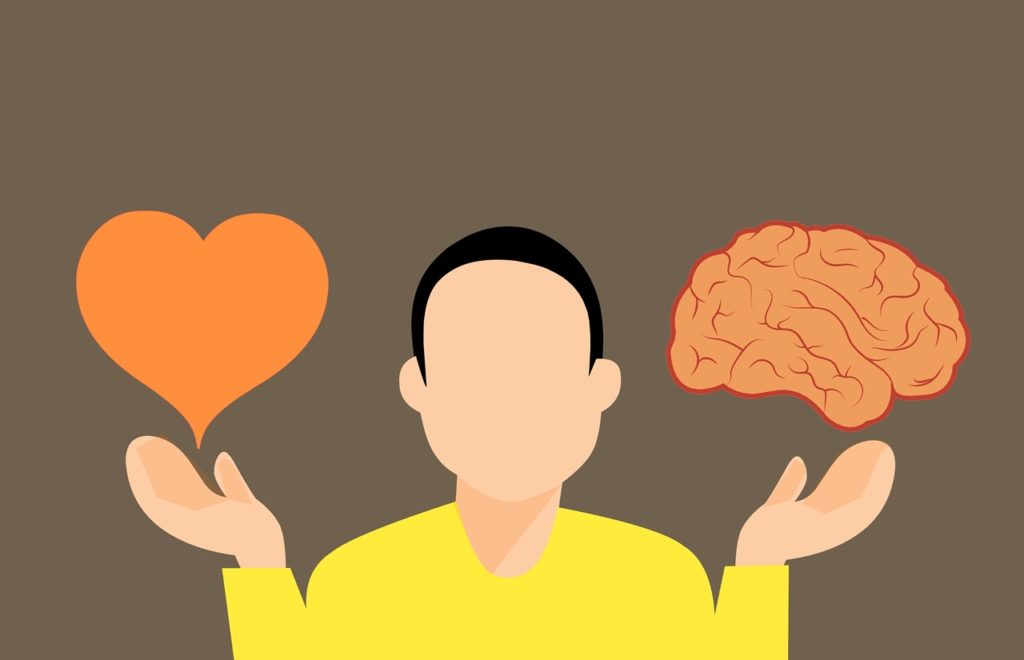
These results may prompt some serious questions about identity and politics, but also provide scope for clinically orientated intervention programs.
Conflicts of interest
I have made reference to a previous paper and blog by myself within the implications section of this blog. This is admittedly reference to subjective interpretation of other data, but has been presented with this uncertainty acknowledged and is a potential focus for debate.
Links
Primary paper
Other references
Elston, R et al (2012) ‘Genetic Terminology’ Methods Mol Biol 850:1-9
Hruby A, Frank B, Hu (2015) The Epidemiology of Obesity: A Big Picture’ Pharmacoeconomics 33(7): 673–689.
Janaway, BM (2018) Lifestyle training for schizophrenia: STEPWISE fails to make a difference’ The Mental Elf, Online (First accessed 4/2/19)
Jung, SJ et al (2017) ‘Association between body size, weight change and depression: systematic review and meta-analysis’ BJP 211:1
Ogunbode AM et al (2011) ‘Health Risks of Obesity’ Ann Ib Postgrad Med Dec; 7(2): 22–25
Romeral-Corral, A et al (2008) ‘Association between body size, weight change and depression: systematic review and meta-analysis.’ Int J Obes 32:6
Smith, GD (2016) What is Mendelian Randomization and How Can it be Used as a Tool for Medicine and Public Health? Opportunities and Challenge. CDC online: https://www.cdc.gov/genomics/events/precision_med_pop.htm (first accessed 17/3/19.)
Smith, GD et al (2008) ‘Mendelian Randomization: Genetic Variants as Instruments for Strengthening Causal Inference in Observational Studies’ National Research Council (US) Committee on Advances in Collecting and Utilizing Biological Indicators and Genetic Information in Social Science Surveys; National Academies Press, Washington, 16.
Steptoe, A et al (2015) ‘Subjective wellbeing, health, and ageing’ The Lancet 385:9968
van den Broek N et al (2018) Causal associations between body mass index and mental health: a Mendelian randomisation study’J Epidemiol Community Health 72:708-710.
Verbank, M et al (2018) Detection of widespread horizontal pleiotropy in causal relationships inferred from Mendelian randomization between complex traits and diseases’ Nature Genetics, 50:693–698