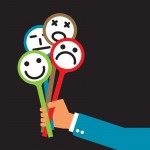
“I have the choice of being constantly active and happy or introspectively passive or sad. Or I can go mad by ricocheting in between.”
– Sylvia Plath, the Unabridged Journals of Sylvia Plath
Things were neater in medical school. People with affective disorders had affective problems, people with personality disorders had personality problems, and hearing voices meant you were psychotic etcetera etcetera. It worked for me, and it worked for the examiners.
But from my earliest days of real life psychiatric training, I could see the skill went far beyond simply deducing the diagnosis. Being a sensible psychiatrist meant always remaining open to whatever it was the client felt was their main concern, whether that ranked high up the ICD-10 list or not. Certain symptoms may not be inquired about if they are not expected for example, or seem less ‘important’ with a given diagnosis.
In a new paper in BMJ Open, Patel et al (2015) explore the prevalence and influence of one such symptom: ‘mood instability’.
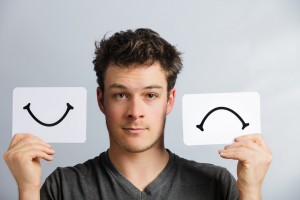
Mood instability is clinically important, but has received relatively little research attention up to now.
What did they want to know?
Of course, inquiring about mood is part of the routine mental state examination, but what about the specific experience of mood instability? The authors ask the following questions:
- How common is mood instability in people with mental illness, across a number of diagnoses?
- What is its relationship with the following outcomes:
- Days spent in hospital
- Frequency of admissions
- Likelihood of being sectioned
- Chance of being prescribed antipsychotics and mood stabilisers, up to 5 years after presentation?
Of note, this is also the first study to use an ‘automated information extraction method‘ to acquire data on mood instability from electronic health records.
Methods
Study design
This was an observational study covering 27,704 adults (size matters!) presenting to the South London and Maudsley NHS trust (SLAM) in the UK. SLAM is a large mental health provider in South London, with an urban catchment of 1.2 million people.
Who was included?
Adults aged 16-65 with diagnoses of schizophrenia and related disorders, bipolar disorder, depression (with or without psychosis) and any other affective (mood-related) disorder.
What were the timings?
Data was collected on people presenting between April 2006 and March 2013.
Follow-up data on service use was collected at one year (n=27,704), two years (n=24,848), three years (n=21,188), four years (n=17,130) and five years (n=13,032).
So what’s this electronic system all about?
The study was carried out using the SLAM Biomedical Research Centre (BRC) Case Register. The trust has used a single electronic health record system since 2006, and the BRC Case Register extracts anonymised data from it. Healthcare professionals use the free text to document clinical information. The study made use of the Clinical Records Interactive Search (CRIS), described as a ‘bespoke database search and assembly tool’, which has previously supported a range of studies.
What does automated data extraction involve?
Specialist software was used to search the free text of the electronic documentation to find information relating to three broad, relating terms: ‘mood’, ‘affect’ and ’emotion’, and the most frequent modifier words around them. The authors manually searched the results to find the modifier terms relevant to mood instability (see a list in the online supplement of the paper PDF). The resulting list is pretty thorough, and includes common misspellings of relevant words (e.g. ‘changeable’ and ‘changable’).
All sentences containing the selected terms/modifiers were extracted and used to develop applications that could identify constructs of instability of mood, affect and emotion.
For each application, a human annotator manually checked for the presence of the construct in 300 reference sentences. Inter-annotator agreement was strong. Next, they used a ‘supervised machine learning approach with active learning‘ to develop more sensitive and specific applications. The smart software achieved 90% precision (I have to admit such detailed descriptions of smart machines with active learning unsettled me a little: too much ‘Asimov’/Terminator).
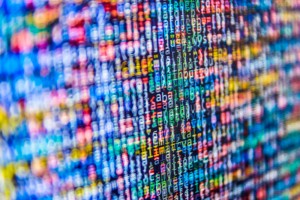
Natural language processing was used to identify the presence of mood instability.
Results
- The presence of recorded mood instability within one month was 12.1%
- It was most common in young (16-25) women
- The strongest diagnostic association was with bipolar disorder (adjusted OR 3.42, 3.06 to 3.82). This seems fairly predictable at first, but it is worth noting they refer to mood instability outside of manic or depressive episodes
- It was also present in other conditions, but more commonly in schizophrenia and personality disorders (there is no breakdown into specific personality disorders)
- Having mood instability increased one’s likelihood of being prescribed antipsychotics (2.03, 1.75 to 2.35) and mood stabilisers (2.07, 1.77 to 2.41) over time. Of course, given the observational nature of the study they could not make inferences about whether the medications were being prescribed specifically for the mood instability or other aspects of the illness
- The presence of mood instability meant spending longer in hospital (β 18.5, 95% CI 12.1 to 24.8), being admitted more frequently (incidence rate ratio 1.95, 1.75 to 2.17) and an increased likelihood of being detained under Section of the Mental Health Act (OR 2.73, 2.34 to 3.19).
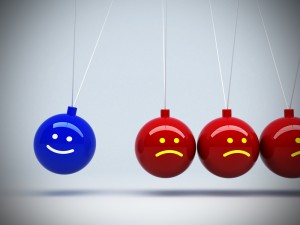
Mood instability was most frequently documented in people with bipolar disorder (23%), but was also common in people with personality disorder (18%) and schizophrenia (16%).
Strengths and limitations
Numbers aren’t everything, but they help. And a major strength of this data is the sample size (over 27,000). They made use of data entered routinely by clinicians, on patients who were ordinarily in contact with services (as opposed to being recruited for research purposes), which increases generalisability. In addition, the use of an automated service will have saved on time and money.
Any large database, especially one that is filled out routinely by clinicians, runs the risk of missing data. The authors took steps to show this was unlikely to have a significant impact on the results, but they were restricted by the fact.
Other limitations included the fact they only looked at mood instability over a one month period, only looked at it as being present or not (as opposed to degrees of mood instability for example) and they limited the list of diagnoses to those listed above. I would have been interested to see prevalence rates in other disorders such as obsessive compulsive disorder or ADHD (where mood instability has previously been pointed out to be a feature). I also wonder if emotionally unstable personality disorder warranted a category of its own, given the focus of the study.
Of course, any data collected from clinical records relies on how complete and thorough the clinicians are in filling them out. It is possible this is an underestimation of the prevalence of mood instability in those with mental disorders (other papers have quoted prevalence rates of up to 83.8% (Gilbert et al 2005). Getting a more accurate picture of mood instability would ultimately require use of a validated tool design to capture it, but a recent systematic review concluded ‘no single measure comprehensively assesses affective instability…and a new short measure…that is reliable and validated against external criteria is needed.’ (Marwaha et al 2014)
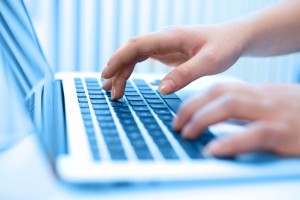
It’s possible that mood instability was not always recognised and documented in electronic health records.
Conclusion
In summary, this paper highlighted the benefits of using automated systems to search through large databases (where there is consent to do this of course), particularly when assessing ‘easy to capture’ outcomes such as service use. It serves as a solid starting point for further research into mood instability, crucially about what to actually do about it when it comes up in clinic. It adds to a wider discussion about disorders featuring mood changes, such as the ‘borderline versus bipolar’ question (Richardson & Tracy 2015).
I will make more of an effort to ask about it, especially in people with diagnoses that are not ‘traditionally’ associated with fluctuating mood. I will also make sure my medical students have a more realistic appreciation of the complexities of the human mind than I clearly did…
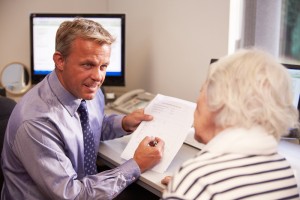
These findings suggest that clinicians should screen for mood instability across all common mental health disorders.
Links
Primary paper
Patel R, Lloyd T, Jackson R, Ball M, Shetty H, Broadbent M, Geddes JR, Stewart R, McGuire P, Taylor M. Mood instability is a common feature of mental health disorders and is associated with poor clinical outcomes. BMJ Open. 2015 May 21;5(5):e007504. doi: 10.1136/bmjopen-2014-007504.
Other references
Marwaha S, He Z, Broome MR, Singh SP, Scott JE, Wolke D. (2014) How is affective instability defined and measured? A systematic review (PDF). Psychological Medicine, Volume 44 (Number 9). pp. 1793-1808.
Gilbert P, Allan S, Nicholls W, Olsen K. (2005) The assessment of psychological symptoms of patients referred to community mental health teams: distress, chronicity and life interference. Clin. Psychol. Psychother., 12: 10–27. doi: 10.1002/cpp.426 [Abstract]
Mood Matters: mood instability is common and associated with poor outcomes http://t.co/GPZ9rK2gr4 #MentalHealth http://t.co/1V7xPcY5EN
Morning @OxPsychiatry Look! @FarhanaMann has blogged about your mood instability paper today http://t.co/WqN9CEOzWM What d’you think?
@FarhanaMann great to see you’ve joined the @Mental_Elf clan! Hope all is well with you Farhana ?
Today @FarhanaMann on a recent @BMJ_Open observational study of mood instability in people with mental illness http://t.co/WqN9CEOzWM
Mood instability is emerging as an important phenomenon – @FarhanaMann blogs for @Mental_Elf
http://t.co/7bsNBIp21U
1 month EHR study finds mood instability documented in bipolar (23%), personality disorder (18%), schizophrenia (16%) http://t.co/WqN9CEOzWM
Mood Matters: #mood instability is common and associated with poor outcomes via @Mental_Elf http://t.co/6fjLO1Weva
Read the excellent: Mood Matters: mood instability is common and associated with poor outcomes https://t.co/0sEwM5wtEI
Nice reminder that syptoms/phenomena (mood instability here) important; diagnosis alone is not enough https://t.co/6SLSDDDMW7 via @sharethis
Innovative study says that clinicians should screen for mood instability across all common mental health disorders http://t.co/WqN9CEOzWM
Mood instability is assoc w/ poor functioning, unhappiness, low self-esteem, > use of health services & suicidality http://t.co/WqN9CEOzWM
During the mental hospital era, the patients ended there, when families could not cope and care anymore. Recently a study on newly diagnosed with schizophrenia only 37 % of psychoses were hospitalized (1). Suicide risk and danger to others seemed to be the reason for admission I guess it became to difficult for the people in the surroundings.
As the young women from 16 years and above had the most changes of mood, one can suspect that the admissions were made to relieve relatives.
Too bad that in studies like this not to study if you live alone or with family. Would guess that to live alone and without contact with others provides some protection against hosptalization . In schizophrenia, there may be negative with untreated psychosis cared by oneself. So I wonders how psychoses as “cared” at home without psychiatry intervention affect functional capacity in the longer term. In Sweden a person with schizophrenia have on average 1.4 psychiatrists visits per year and 16 nurse meetings per year not much (depotinjections?). But some get assessed once a month for menthal status.
So my question is Are the people with mental illness that are hospitalized most ill or are they the most supervised by others?
1. http://archpsyc.jamanetwork.com/article.aspx?articleid=2323629
Read our blog of the first study to investigate mood instability from health records of ppl w/ mental illness http://t.co/WqN9CEOzWM
Don’t miss: Mood Matters: mood instability is common and associated with poor outcomes http://t.co/WqN9CEOzWM #EBP
@Mental_Elf I walk the dark road of MH. The ground is pitted with pot holes. My journey is without end as at present it has no destination.
Summary of fascinating machine learning analysis of “mood instability” in clin notes: common & not just BPD/#bipolar http://t.co/u37DSY210U
Mood Matters: mood instability is common and associated with poor outcomes https://t.co/zYvwS6gOkF via @sharethis #mentalhealth #treatment
Mood Matters: mood instability is common and associated with poor outcomes https://t.co/Gw5nMAAq0e via @sharethis