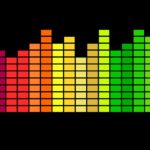
Powerful computing now facilitates the analysis of enormous healthcare datasets. Making sense of any healthcare data requires an understanding of the processes giving rise to the measurements. Where diagnoses, or judgments about the nature of a person’s state of health are concerned, this is especially complicated because different people can disagree about the correct diagnosis, diagnoses can change over time, and a person may receive multiple overlapping diagnoses.
Notwithstanding obvious cautions in considering psychiatric diagnosis as a process, diagnoses remain crucial to the organisation of psychiatric services. However, people who use mental health care with a particular diagnosis may therefore not represent the true group of people in the population with that disorder. This is important because presenting to services for a mental health problem, such as depression, might reflect not the factors that caused the depression, but more about the social and cultural circumstances in which the person finds themselves. Because of these issues, understanding mental illnesses occurring in the general population has typically involved recruiting a large number of participants and following them up with structured psychometric and health measures over time, or cross-sectional surveys of household residents in a given population of interest.
This raises the question, how closely do administrative diagnoses correspond to those assigned by researchers? This is in line with the broader debate about the relevance of administrative data for understanding healthcare outcomes and interventions. In particular, administrative data have been accused of being noisy, inconsistent, poor quality, and misleading. One way of addressing this is to better understand the processes underlying the generation of the data. A recent paper, by Davis et al (2018), assesses the correspondence of one type of administrative data on mental health service use, Hospital Episode Statistics, with detailed case review (review of notes).
The study used data from the CRIS system (a searchable and anonymised electronic database of mental health records from the South London and Maudsley NHS Foundation Trust) with routinely collected data on all people receiving specialist mental healthcare since 2006 in four boroughs of South East London (Lambeth, Southwark, Croydon, and Lewisham). For each patient, CRIS contains a broad range of information on social demographics, diagnostics, investigation results, treatment, and adverse events, among other things. The CRIS system is reported in an earlier paper by Stewart et al. (2009).
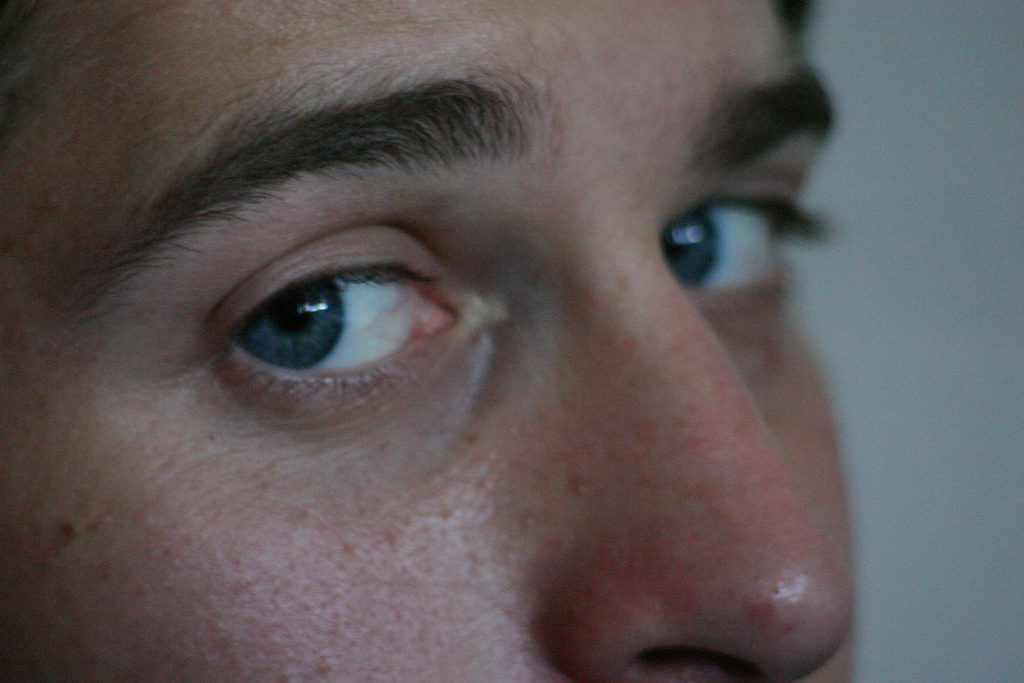
Existing methods for measuring the prevalence of mental illness in the population can be noisy, inconsistent, poor quality and misleading.
Methods
Hospital Episode Statistics (HES) are nationally collected data on use of hospital care in the NHS, with data on diagnoses, admission dates, and duration of stay. In this paper, the investigators looked at HES records for 350 people with at least one HES admission for a diagnosis of schizophrenia, schizophrenia spectrum disorder, bipolar affective disorder, or unipolar depression. Having identified this group, the researchers collected HES-derived data on all available admissions drawn from the HES database on these individuals.
Having identified 250 of these admissions for validation, clinical notes were systematically examined by a psychiatrist, with the aim of identifying the most appropriate clinical diagnosis corresponding to that admission, and identifying a lifetime diagnosis. Where multiple symptom groupings were evident from the notes, an accepted hierarchy of diagnosis was used: this hierarchy tends to prioritise the diagnosis of schizophrenia-type disorders over the diagnosis of affective disorders, because schizophrenia-type disorders are considered more pervasive, severe and influential on lifelong functioning, and because they themselves influence the presentation of symptoms further down the hierarchy (such as affective symptoms).
Clinical information for providing the diagnosis was derived by the OPCRIT, a symptom checklist that systematically gathers symptoms information at the item level, and which can be used to derive clinical diagnoses in line with current diagnostic classifications. Clinical information on symptoms were summarised into a short abstract, which was then reviewed by a second psychiatrist, who applied their diagnosis, which was cross-checked with that of the first.
Results
Of 250 cases selected, insufficient information to provide a diagnosis was found in 8 cases, either because they were transferred out of the trust before a diagnosis could be made, or because clinical data for the admission was not found. This resulted in 242 research index diagnoses, and 246 lifetime diagnoses. Around half the research diagnoses were marked as uncertain, mainly in relation to whether the subject fulfilled criteria for one or other mental disorder, except in one case, whether there was uncertainty regarding whether the patient had a diagnosis of mental illness at all (“normal vs. abnormal”).
- Among the patients identified, those with a diagnosis of unipolar depression seemed to have shorter duration of contact with services
- Black ethnicity was more common in those with a diagnosis of schizophrenia and related disorders
- A fifth of records for HES diagnosis corresponded exactly to the clinical diagnoses assigned by the research team
- However, when they considered broader diagnostic groupings (depression, psychotic disorders, bipolar affective disorders) there was agreement in around two-thirds of patients. This suggests that at the finest grain level, correspondence between diagnoses was poor, but improved significantly when broader subgroups were used for comparison
- Disagreement was most common for the broader groupings of diagnosis, between circumstances where the researcher identified the diagnosis as schizophrenia, but the HES record determined that the diagnosis was schizophrenia spectrum disorders.
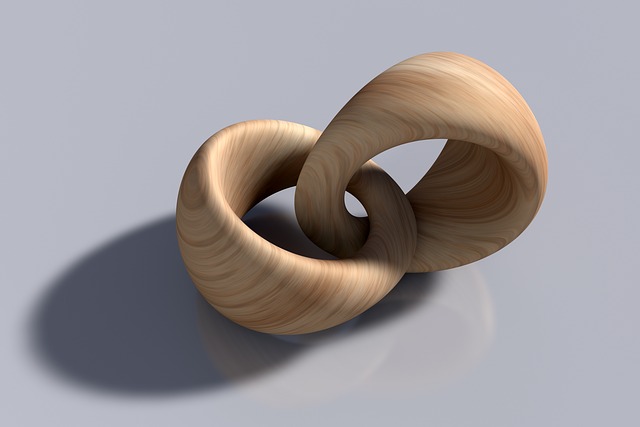
Correspondence between diagnoses was poor, but improved significantly when broader subgroups were used for comparison.
Conclusions
The authors conclude that administrative diagnoses from mental health hospital admissions in the UK NHS are broadly valid and useable for research. Indeed, the paper is reasonable evidence that, where a hospital diagnosis of depression, bipolar, schizophrenia, or schizophrenia spectrum disorder is concerned, that these diagnoses correspond pretty well to what would be reflected in the detailed clinical record.
Having a HES diagnosis of schizophrenia was accompanied by a greater than 90% probability of having this diagnosis based on the clinical records (“positive predictive probability”), which is quite encouraging. But if you have a HES diagnosis of bipolar affective disorder, there is a 70% chance of having this diagnosis, based on the clinical record.
The authors suggest that the results of this study conform to those of a systematic review of administrative diagnoses validity they same authors carried out previously. The results provide an encouraging comparison of clinical practice with the data that is typically gathered. For clinical practice in general, it emphasises the utility of schizophrenia and other broad diagnostic groupings, possible over narrower diagnostic codes. HES data are therefore a reliable indicator of clinical practice.
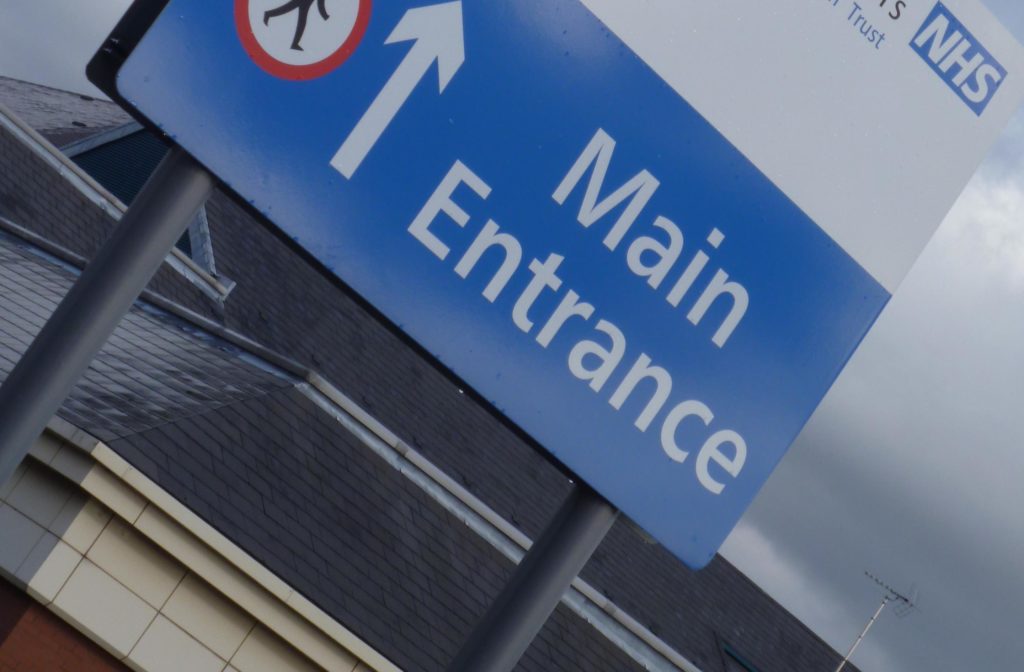
This study suggests that administrative diagnoses from mental health hospital admissions in the UK NHS are broadly valid and useable for research.
Strengths and limitations
The authors point to some limitations: the reliability between the raters was lower than expected, and there was more-than-expected disagreement between the raters. The authors also suggest that the results may not be generalisable to other centres, on account of the study being set in a Trust widely regarded as a “centre of excellence” in psychiatry. The question is therefore raised whether the results would be similar if the same study were to have been carried out in other parts of the UK mental health services. In the majority of cases, a secondary diagnosis was not recorded, reflecting the clinical reality where secondary or comorbid diagnoses are probably under-used. The researcher diagnoses themselves were considered to be uncertain in around half of cases. The frequent diagnostic uncertainty is borne out in clinical experience; and it may be that this could have been better handled in this paper. Could diagnostic uncertainty be more realistically considered a spectrum, rather than a yes/no phenomenon?
Nevertheless, this paper emphasises that hospital diagnoses of psychiatric diagnoses correspond well to clinical details in the notes. This means that these diagnostic data could be useable for research, as they are comparable to the diagnoses that psychiatrists would make based on the clinical information.
On the other hand, there is a risk of this paper concealing considerable complexity underlying both processes of data collection; we actually still know relatively little about both types of measurements made in this study. On the one hand, the way in which diagnoses are entered in HES is not made clear in the paper, and we are given relatively little information on the psychiatric assessor, or information on whether they are likely to represent the best quality or most representative diagnostic test of clinical records; given they are a researcher, working in a research-oriented mental health trust, with specific levels of seniority, level of training, and range of prior experience.
So interpretation of the results should be cautious and combined with other kinds of research, which focus on how diagnoses are derived in clinical settings, and how they correspond to the diagnoses that are made in research studies (rather than based on review of clinical records, as done here). The subject of understanding data-generating processes is topical: a recent paper in the British Medical Journal (Agniel et al, 2018) examined electronic health record data for survival in people undertaking 272 lab tests, finding that factors reflecting healthcare processes, such as whether the person undertook the test or not, and the timing of the test during the day, were more strongly associated with survival at three years than the results of the tests themselves.
In mental healthcare, it might be possible, for example, that psychiatric diagnoses made in clinical practice could reflect a person’s social circumstances as well as the symptoms with which they present.
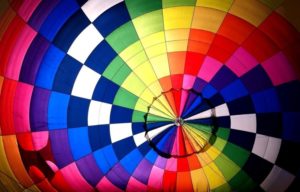
Could diagnostic uncertainty be more realistically considered a spectrum, rather than a yes/no phenomenon?
Implications for practice
If we assume that HES diagnoses are derived mostly from clinicians, this research implies that the diagnoses among clinicians broadly agree, especially for schizophrenia-type disorders. Although this is encouraging, clinicians should also pay attention to how we arrive at diagnoses in clinical settings, and how these diagnoses might be used for research, or to inform policy, in the future. For researchers in the future, there needs to be clearer understanding of how clinical diagnoses correspond to diagnoses applied by research studies in research interviews, in order to use clinical diagnoses for research purposes.
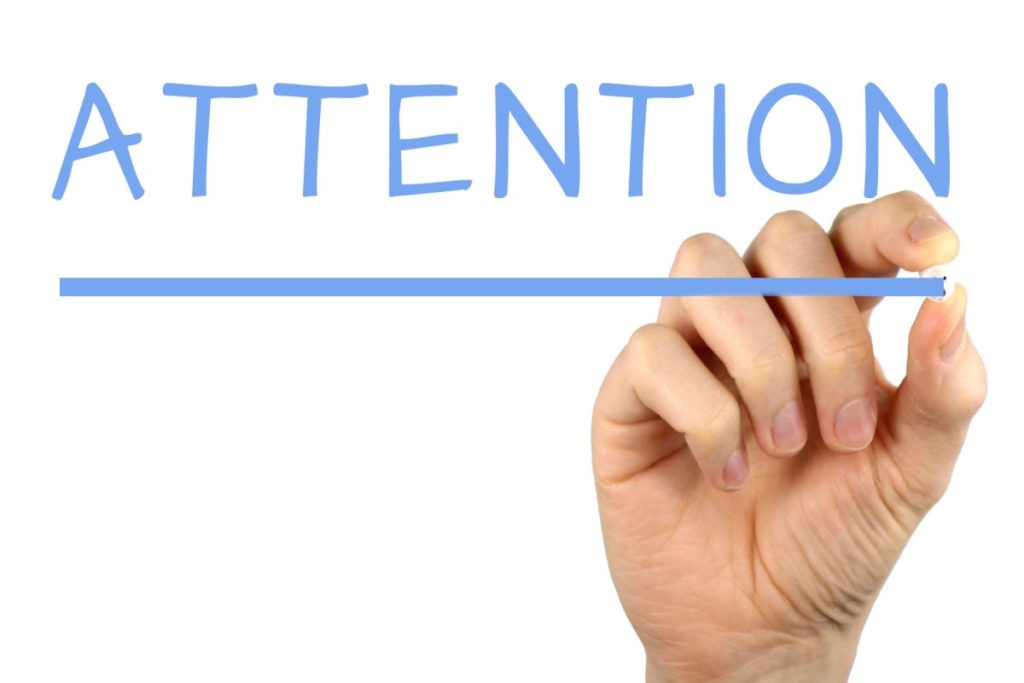
Clinicians should pay attention to how we arrive at diagnoses in clinical settings, and how these diagnoses might be used for research, or to inform policy in the future.
Links
Primary paper
Davis KAS, Bashford O, Jewell A, Shetty H, Stewart RJ, Sudlow CLM, et al. (2018) Using data linkage to electronic patient records to assess the validity of selected mental health diagnoses in English Hospital Episode Statistics (HES). PLoS ONE 13(3): e0195002. https://doi.org/10.1371/journal.pone.0195002
Other references
Agniel, D., Kohane, I. S. & Weber, G. M. 2018. Biases in electronic health record data due to processes within the healthcare system: retrospective observational study. BMJ, 361, k1479.
Stewart, R., Soremekun, M., Perera, G., Broadbent, M., Callard, F., Denis, M., Hotopf, M., Thornicroft, G. & Lovestone, S. 2009. The South London and Maudsley NHS foundation trust biomedical research centre (SLAM BRC) case register: development and descriptive data. BMC psychiatry, 9, 51.