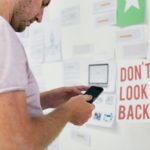
Smartphone ownership in the United States has increased from 35% in 2011 to 72% in 2016 (and higher in those under age 35 at 92%) (Poushter, 2016). “More than 90% of owners report having their phones charged, turned on, and constantly within arm’s reach” (Rainie & Zickuhr, 2015). Current smartphones are several times more powerful than the Cray-2 supercomputer, the 1980s fastest computer. Smartphones, have changed the game for digital interventions. These beloved tiny supercomputers present an opportunity for mental health to deliver ‘ecological; momentary’ interactions (EMIs) in harmony with the fabric of people’s lives.
Often in mental health when thinking about the development of health apps we find ourselves struggling to fully conceptualise what it is we are attempting to do and why. “Ecological momentary interventions for depression and anxiety” by Schueller et al (2017) brings together some useful ways of thinking about apps for mental health and how we might understand them.
The authors are keen that we review where we have been with digital mental health apps so that we might begin to develop a far more exciting digital mental health future. The paper includes a number of ideas useful to those of us looking to understand and develop ways of making people’s lives better using digital technologies. The paper also makes a number of useful distinctions between different types of interactions between patients and technology and explores how we might better understand them.
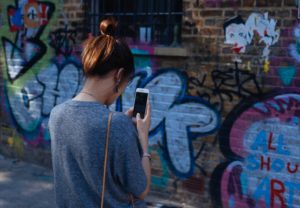
Ecological momentary interventions (EMIs) are treatments that are provided to people during their everyday lives (i.e. in real time) and in natural settings (i.e. real world) (Heron & Smyth, 2010).
Methods
Schueller et al discuss methods of understanding what digital interventions for depression and anxiety actually are, ways of evaluating these interventions and report recent evidence for the efficacy of such interventions. Their paper also suggests a future path for digital mental health application development.
A brave new world?
Smartphones make new kinds of health intervention possible. Rather than sitting down to do a health related task, interventions can be quick and take place in the context of other everyday activities. We make momentary ecological interventions with our smartphones hundreds of times a day; from firing off a quick email to checking our bank balance. Once the threshold for digital health was Ecological Momentary Assessments (EMAs). Such assessments might encourage people to answer a question about their current feelings or asking people to measure something such as heart rate or blood sugar, extending the ‘window of observation’ into people’s lives and allowing the collection of data by asking people to feedback via an app.
Schueller et al are not interested in EMAs, though. It’s Ecological Momentary Interventions (EMIs) that have caught their imagination. EMIs are “momentary health treatments provided via hand-held mobile technologies that deliver psychological interventions while people are engaged in their typical routines in their everyday life” (Heron & Smyth, 2010). The goal of EMIs is to provide relevant, useful intervention strategies in the context of people’s daily lives. EMIs consist of a combination of intervention options and decision rules that specify when and why those interventions will be deployed.
Technology, tailoring and integration into the lives of people
EMIs are integrated into the fabric of people’s lives. Say the authors: “EMIs, by their nature, aim to provide contextualized, personalized, and impactful experiences to each user.” The authors are keen to draw attention to the fact that EMIs can be dynamic, learning from their interactions with the patient and modifying how and when interventions are deployed.
The advent of powerful smartphones with a high user-base make tailored and dynamic interventions possible. As the authors state: “EMIs are deployed in people’s real lives and as such often require a great deal of data collection to inform their deployment and evaluation. Information can draw on both actively and passively collected data. Active data collection requires some action on the part of the user such as completing a survey. Passive data collection requires no action on the part of the user and may come from embedded phone sensors (e.g., GPS, phone activity, screen state) or other devices (such as smartwatches that may provide heart rate, accelerometer, etc.).” Complex EMIs have a “set of intervention options, decision points as to when those interventions can be potentially provided to a user, tailoring variables such as information about the user or the context, decision rules that link tailoring variables to intervention options.”
The paper discusses the emergence of a complex class of integrative EMIs where “a learning system evolves over time, continuously improving its responses to a user’s pattern of responses and interactions with the system.”
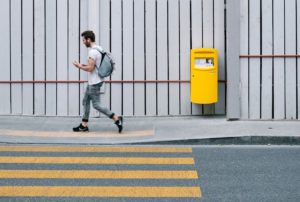
Ecological momentary interventions can be dynamic, learning from their interactions with the patient and modifying how and when interventions are deployed.
Thinking about how we measure digital interventions
Digital development often focuses on immediate user response to features and may not recognise whether the responses are effective proxies for improvement in conditions. Medical trials often do not recognise the individual actions that make up the overall experience of using a digital intervention, measuring only the overall outcome against the intended one. Taken together it can be challenging for those developing digital interventions to measure what is needed to both show efficacy and to improve the product or service in development.
In light of this, Schueller et al point out that it is important to distinguish between proximal and distal outcomes in our measurement of digital health interventions. The difference between the two measures could be phrased as ‘did this make you feel better right now or has it made you more well over time?’ Proximal outcomes are those recognised or reported immediately such as ‘did reading this text make me feel less worried?’ or ‘did completing this task improve my mood?’. Distal outcomes are the sum of such interactions over time and their effect upon a person’s overall condition.
The authors remark: “in mental health, distal outcomes often change slowly or have a period of time associated with their evaluation (e.g., past 2 weeks for a major depressive episode). Proximal outcomes can change more rapidly, sometimes in direct response to a specific intervention.”
Schueller et al maintain that where EMIs are dynamic they do not always fit well within existing trial methodologies. They state that randomised controlled trials (RCTs) tell us whether something was effective over time but “often do not provide sufficient information of what intervention to provide when and how much”. They also remark that behaviour change theories do not account for the highly interactive applications that tailor themselves to the individual.
An example of an intervention ill-fitted to an RCT would be an app using a “bandit algorithm” to tailor the chance of a particular intervention being given to the user based on data about what has worked in the past. In an intervention where there are instructions for deep breathing and progressive muscle relaxation, they might begin with a 50/50 chance of being served to the user. However, if deep breathing works for the user, the odds will be shifted in favour of serving the user that effective intervention. The impact of an intervention might be influenced by time of day, recent stressors, location or other variables. This poses a challenge for evaluating effectiveness as not every user gets the exactly the same intervention.
The authors use the behavioural intervention technology (BIT) model (Mohr et al, 2014) to help understand the components of EMIs. This model makes a distinction between BIT “treatments” and BIT “interventions.” A BIT intervention “refers to a specific single interaction between a user and an element of the technology, which might be, for example, a single text message or push notification”. In contrast, a BIT treatment is the sum of a number of such interactions over time.
The paper makes it clear that we are looking for different things when we are trying to measure the effect of a BIT intervention versus the effect of a BIT treatment. When we are measuring the effectiveness of a BIT intervention we are looking at proximal ‘in the moment’ outcomes, such as ‘does reading this message make someone carry out a coping strategy?’ or ‘does reacting to this piece of content raise mood?’. BIT treatments are measured over time and should focus on the distal long term treatment outcomes such as the reduction of symptoms or a change in diagnostic status.
The challenge, the authors believe, is that “on a group level what is being evaluated for an EMI is the sum of the interventions, tailoring variables, decision variables, and decision rules. The fact that sum of these aspects may evolve over the course of a trial (e.g., the decision rules may be updated based on new information gained) means that traditional RCTs may be a poor fit for early stages of EMI development.”
Where EMIs include learning models, where there is no one standard treatment but a tailored variety of interventions, the authors suggest inverting the direction taken by RCTs suggesting that evaluation methods should “focus on generating knowledge at the individual level first and then move to generalize to the population level. This is different than RCTs that make inferences on means (i.e., group-level analyses) first before conducting within-person or moderator analyses that might attempt to make recommendations to individuals.”
The authors suggest that microrandomized trials (MRTs): “a sequential factorial design that randomly assigns an intervention component to each individual at relevant time points” (Klasnja et al., 2015) may hold great promise for solving this problem. In an MRT, each individual is randomised multiple times. According to the authors: “MRTs determine the immediate (proximal outcomes) and long-term (distal outcomes) effects of a particular intervention component, how those effects change over time, and which variables indicate when and how to intervene most effectively.” The authors give the example of an MRT including three decision points in the use of an app, which each day would randomise each individual at each decision point to receive a specific intervention or no intervention at all. This makes it possible to measure both whether someone’s condition improved over time and to also measure the impact of individual interventions.
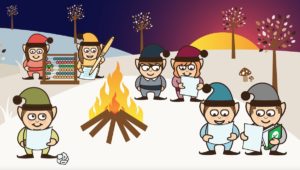
Microrandomized trials randomly assign intervention components to individuals at relevant time points. I’ve no idea why, but they’re immediately appealing to us little elves.
Results
The paper also reviews the evidence on efficacy on EMIs for depression and anxiety to date.
According to the authors: “EMIs intended to reduce depression do so through interventions aimed at various proximal outcomes such as
- Engagement in pleasurable activities,
- Increasing positive emotions,
- Or other pathways.
EMIs for depression have also made use of diverse conceptual treatment strategies including:
- Acceptance and commitment therapy and
- Interpersonal therapy,
- But the majority have been cognitive-behavioral in focus including
- Cognitive-behavioral therapy (CBT),
- Behavioral activation,
- Relaxation, and
- Self-monitoring.”
The authors summarise a recent meta-analysis of EMI studies targeting symptoms of depression and other associated mental health outcomes (Versluis et al, 2016). The meta analysis identified 33 studies, 17 of which included a measure of depression as an outcome with levels ranging from mild symptoms to those meeting criteria for major depressive disorder, with a low quality rating of the studies analysed on a six point scale. EMIs had a small-to-medium effect on within-person change in depressive symptoms with supported interventions tending to be more efficacious than unsupported interventions.
The authors suggest that EMIs are likely “more effective when they complement and extend the treatment rather than serving as a separate and disjointed adjunct. If a provider does not or is not skillful at integrating it into treatment, as might be the case in usual care, the EMI might not be as effective as a more integrated program… The strongest predictor of compliance and benefit from assigned homework tends to be therapist factors such as reviewing assignments and general therapeutic skill” (Bryant et al, 1999). The authors suggest that better integrating EMIs with human provided therapeutic support might yield the best results.
Regarding EMI interventions for anxiety, the same meta-analysis (Versluis et al., 2016) found 15 studies that examined anxiety symptoms and found a level of within-person change near identical to the depression studies. Another systematic review (Gee et al, 2016) explored reduction in generalised anxiety identifying seven studies that favoured EMIs over comparison or control conditions. Results for other anxiety disorders were mixed, but EMIs targeting stress were significantly superior to comparison conditions. The authors wonder whether stress might be more responsive to EMIs because it may function better as a proximal outcome rather than other symptoms of anxiety which are measured over time. The authors remark that an analysis of the features included in the EMIs found only two used automated sensors despite advances in technology making passive data collection increasingly possible.
They conclude that:
EMIs previously developed and evaluated have shown similar benefits for depression and anxiety to those found in other BITs such as websites and mobile apps.
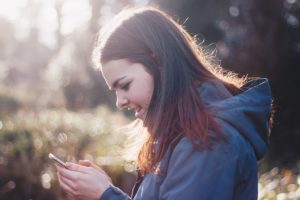
EMIs offer unique challenges and unique promise, because they can be deeply integrated into the fabric of people’s day-to-day lives.
Conclusions
Emerging technologies have the potential to offer real benefits to people with mental health difficulties made possible by two factors: the increase in sophistication of smartphones and their penetration into the lives of a majority of people in western countries. More people carry smartphones and the smartphones they do carry are increasing in sophistication.
Schueller et al make it clear that smartphone technology has extended the horizon of possibility for treatment and also for the monitoring or tailoring of treatment because modern apps can both measure our responses to interventions and also modify those interventions in light of direct feedback. To understand this we must understand how interventions are delivered to individuals, how they might be measured for effectiveness both in the moment and over time.
The paper itself is very much on the side of pushing forwards to the future and makes a number of suggestions that may help to clear current log-jams in utilising technology for better mental health outcomes. The authors betray a certain exasperation at the current state of EMIs, describing them as “somewhat limited”. Many current EMIs “require some initiation by the user either in the form of a request or assessment and interventions can be somewhat clunky usually pulling from a prepopulated option created without knowledge of each user and each context.” Future EMIs should be able to interact with and adapt to the people who use them.
The authors set out a compelling vision of the future of digital mental health interventions where “advances in EMIs are likely to take us one step closer to personal digital mental health assistants. These assistants will listen to people through sensed data, learn from people in the context of their daily lives, and guide people in directions that will support their mental health. Such personal digital mental health assistants will still be made up of combinations of interventions, decision points, tailoring rules, and decision rules but powered by advances in technologies and analytics that make each of these more personalized and more data-driven.”
They believe that a new generation of highly integrative, deeply personalised EMIs is possible if certain advances in technology can be incorporated. The first vital technology is passive detection derived from smartphone sensors. As the authors suggest; “the EMIs of the future might not need to ask people what they need, but be able to tell them based on their ability to collect and process passive data.” The second vital technology is the utilisation of machine learning and algorithms to deeply personalise decisions rules in EMIs so that interventions can be delivered in intelligent and responsive ways while still remaining evidence based.
The paper itself contains a number of examples of actual ecological momentary interventions, but there is a sense that the authors feel that we are only at the beginning of the process of fully developing mental health digital treatments that truly belong to the 21st century.
Given much evaluation of mental health apps has amounted to clinical safety and data governance without a strong line toward establishing clinical efficacy; the clarity with which Schueller and his colleagues set out the issues for evaluating such technology is valuable.
When we look at existing interventions in mental health that are currently available to the public it is difficult not to share at least some of the frustration which underpins this paper while also ingesting its optimism. In a marketplace where people are still lauding mood tracking apps and electronic bulletin boards as dangerously modern it is heartening to see some engaged in mental health technology suggesting a practical line to a more exciting and clinically effective future.
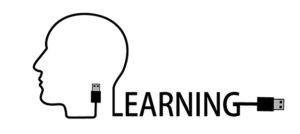
Future EMIs might reduce user burden and increase intervention personalisation and sophistication by leveraging digital sensors and advances in natural language processing and machine learning.
Links
Primary paper
Schueller SM, Aguilera A, Mohr DC. (2017) Ecological momentary interventions for depression and anxiety. Depression Anxiety. 2017; 00 :1-6. https://doi.org/10.1002/da.22649
Other references
Poushter J. (2016) Smartphone ownership and Internet usage continues to climb in emerging economies. Washington, DC: Pew Research Center. Retrieved from http://www.pewglobal.org/2016/02/22/smartphone-ownership-and-internet-usage-continues-to-climb-in-emerging-economies/
Rainie L, Zickuhr K. (2015) Americans’ views on mobile etiquette. (2015). Washington, DC: Pew Research Center. Retrieved from http://www.pewinternet.org/2015/08/26/americans-views-on-mobile-etiquette/
Heron KE, Smyth JM. (2010) Ecological momentary interventions: incorporating mobile technology into psychosocial and health behaviour treatments. Br J Health Psychol. 2010 Feb;15(Pt 1):1-39. doi: 10.1348/135910709X466063. Epub 2009 Jul 28.
Mohr DC, Schueller SM, Montague E, Burns MN, Rashidi P. (2014) The Behavioral Intervention Technology Model: An Integrated Conceptual and Technological Framework for eHealth and mHealth Interventions. J Med Internet Res 2014;16(6):e146 DOI: 10.2196/jmir.3077
Klasnja P, Hekler EB, Shiffman S, Boruvka A, Almirall D, Tewari A, Murphy SA. (2015) Microrandomized trials: An experimental design for developing just-in-time adaptive interventions. Health Psychology, 34(S), 1220–1228
Versluis A, Verkuil B, Spinhoven P, van der Ploeg MM, Brosschot JF. (2016) Changing mental health and positive psychological well-being using ecological momentary interventions: A systematic review and meta-analysis. J Med Internet Res 2016;18(6):e152 DOI: 10.2196/jmir.5642
Bryant MJ, Simons AD, Thase ME. (1999) Therapist skill and patient variables in homework compliance: Controlling an uncontrolled variable in cognitive therapy outcome research. Cognitive Therapy and Research, 23(4), 381–399. [Abstract]
Gee BL, Griffiths KM, Gulliver A. (2016) Effectiveness of mobile technologies delivering Ecological Momentary Interventions for stress and anxiety: A systematic review. Journal of the American Medical Informatics Association, 23, 221–229. Retrieved from http://doi.org/10.1093/jamia/ocv043
Photo credits
- Photo by rawpixel.com on Unsplash
- Photo by Clem Onojeghuo on Unsplash
- Photo by Samuel Zeller on Unsplash
- Photo by Luke Porter on Unsplash
Really interesting review, thank you Mark. Now very interested in MRTs and their potential for a much richer source of meaningful data, as it feels like we have been at an impasse as to understanding what MH EMIs work and why and for whom. Thanks again!
Thanks for this Mark, very helpful. As you say, it’s very positive to see more sophisticated e-mental health approaches opening up. A couple of reflections… I suppose one of the risks with intelligent devices, machine learning etc is that it makes users feel paranoid and like they are under surveillance. Tech is a common trope within psychosis already, and I wonder whether for some people this kind of functionality might be aggravating.
The second thing is that I’m interested in the focus amongst e-mental health folks on how we can create extra, specific tech that supports mental health, rather than looking at how ‘general’ tech can undermining wellbeing. Smart-phone addiction, social media pressure etc are becoming genuinely endemic and we know that many of the tech companies are deliberately using techniques from gambling to increase dependency. Are there agencies / individuals from the mental health sector who are working to make mainstream tech more supportive of wellbeing?