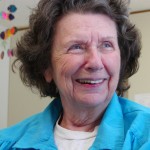
In this systematic review and meta-analysis, Tsoi and colleagues from Hong Kong aimed to assess the relative effectiveness of common cognitive tests at diagnosing dementia.
Dementia is an umbrella term for a number of different brain diseases that progressively affect a person’s ability to think and function independently. Alzheimer’s disease, for example, is the commonest cause of dementia. The symptoms and the impairment caused by dementia are a result of progressive damage to the brain and a loss of brain cells and connections.
The symptoms a particular person with dementia develops depends on where in the brain the disease is affecting. For example, early on in the disease course Alzheimer’s affects an area of the brain called the hippocampus, which is involved in storing memories about our lives. For this reason patients with Alzheimer’s disease get memory problems early on. By comparison, frontotemporal dementia affects the frontal area of the brain first and, as a result, these patients often have changes in personality and difficulties in planning long before they have difficulties with memory.
The way we diagnose and detect dementia, therefore, is by systematically assessing the function of various brain regions by using cognitive tests. ‘Cognitive’ here means the ‘higher brain functions’ I alluded to earlier; things like memory, numeracy, visual perception, personality change and planning, to name a few.
Obviously, an exhaustive assessment of a person’s cognitive function would take a very long time – hours, if not longer! While researchers may have hours to spend with patients, most busy clinicians do not and so the Holy Grail is finding a good, brief screening test of cognitive function that allows us to diagnose dementia.
The commonest cognitive test used is called the Mini-Mental State Examination (MMSE). In this test you can score up to 30 points by answering a range of questions that test your orientation to time and place, your memory, attention and so on. The test itself takes about 10 minutes to complete. As the authors of this paper state, the performance of the MMSE in detecting dementia as compared to other tests has not been systematically assessed and so, that is what they set out to do. One of the reasons to assess the relative merits of the MMSE is that it is a proprietary instrument, owned by ‘Psychological Assessment Resources’ meaning that it is not actually free for organisations to use.
In this paper, the authors completed a systematic review of the literature for studies that:
- Assessed the performance of the MMSE at being able to correctly detect dementia; and
- Compared it to other measures that fell into three categories; tests that took less than 5 minutes to complete, 10 minutes and 20 minutes
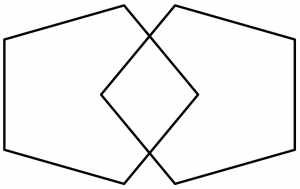
This systematic review compares the MMSE with other tools for detecting dementia. [Interlocking-Pentagons used in the Mini-Mental State Exam].
Methods
The reviewers included studies that:
- Looked for patients with either Alzheimer’s, vascular dementia or Parkinson’s disease in any clinical setting
- Assessed patients or carers face-to-face
- Used a standardised diagnostic criteria to diagnose dementia
- Published the outcome measures they were interested in.
They excluded:
- Non-English language papers
- Tests that took longer than 20 minutes to complete
- Tests that were only evaluated in four or less papers
- Any patients who were visually impaired.
In terms of how the search was performed, it looks very thorough. They searched MEDLINE, EMBASE, PsychoINFO and Google Scholar from the earliest available dates stated in the individual databases until 1 Sep 2014. Two authors independently assessed the search results and used a standardised data extraction sheet. The studies were also screened for quality and bias.
As outcomes they chose several different measures of diagnostic accuracy that can get a bit confusing. The perfect test should be able to tell you everyone who has the disease and correctly identify everyone who does not have the disease…easier said than done.
To understand what the results of this paper mean it is worth running through an imaginary scenario.
How do diagnostic tests work?
Let’s imagine 100 people come to a GP to get tested for ‘Disease X’. The GP decides to compare a new test he’s just bought with the gold-standard perfect test. Using the gold standard he finds out that 50 people have the dreaded ‘Disease X’ and 50 people do not. He then compares these results with his new test, which you can see in the table below.
People tested who do have Disease X (n = 50) | People tested who do not have Disease X
(n = 50) |
|
New test came back as positive | 35
These are true positives (TP) – this is good |
10
These are false positives (FP) – this is bad. |
New test came back as negative | 15
These are false negatives (FN) – this is really bad! |
40
These are true negatives (TN) – this is good too. |
From these kinds of tables you can work out how good a new/alternative diagnostic test is. As you can see from this imaginary scenario, the new test misdiagnosed 20 of the 100 people.
In this paper, they chose to look at a number of different options for assessing the effectiveness of each of the cognitive tests they were interested in. It’s probably not worth going through all the measures they used, but it’s worth knowing about two: sensitivity and specificity.
Sensitivity and specificity
Sensitivity determines what proportion of people who actually have the disease get a positive test. Or as a formula
- Sensitivity = TP / (TP + FN)
- So, in the example above for Disease X – the sensitivity of the new test is 35/(35+15) = 0.7 or 70%
Likewise specificity determines what proportions of people who actually do not have the disease get a negative test. Or as a formula:
- Specificity = TN/ (TN + FP)
- So, in the example above for Disease X – the specificity of the new test is 40/(40+10) = 0.8 or 80%
For both sensitivity and specificity; the higher the number, the better.
The paper also looks at other measures of the diagnostic accuracy but they are derived from the sensitivity and specificity. Without going into detail, the paper also reports Likelihood Ratios, diagnostic odds ratio and ‘AUC’ or area-under-the-curve.
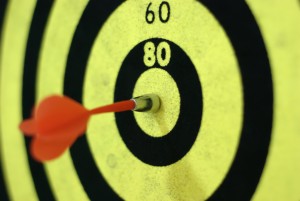
Accurate diagnostic tests have high sensitivity and high specificity.
Results
The initial search yielded 26,380 papers! After applying the inclusion/exclusion criteria they were left with 149 studies, which covered 11 different diagnostic tests and over 40,000 people from around the world.
MMSE
- The vast majority of the studies looked at MMSE (108 of 149)
- Sample size was 36,080 of whom 10,263 had dementia
- From these studies the:
- Mean sensitivity was 81% (CI was 78% to 84%)
- Mean specificity was 89% (CI was 87% to 91%)
- All other markers also showed good diagnostic accuracy (LR+ = 7.45, LR- = 0.21, diagnostic OR was 35.4 and AUC was 92%)
Mini-Cog and ACE-R (the best of the rest)
- Of the 11 remaining tests, two stood out as being ‘better’ than the MMSE
- Mini-Cog (brief test <5 min): sensitivity of 91% and specificity of 86%
- ACE-R (20 min test): sensitivity of 92% and specificity of 89%
- However where the MMSE data was drawn from hundreds of studies:
- Mini-Cog data was drawn from just 9 studies
- ACE-R was drawn from just 13 studies
For all three of the above tests, there was found to be a high degree of heterogeneity. In essence this is a statistical test telling us that between studies included in the analyses, the results were quite different from one study to another. Heterogeneity is not a good thing in systematic reviews.
Further analyses
The reviewers showed that the accuracy of the MMSE was not affected by geographical location or clinical site (i.e. it was as effective for hospital patients as community patients).
Finally they looked at the accuracy of diagnosing mild cognitive impairment (MCI); a risk state that precedes dementia. They didn’t really go into much detail in the methods of how they found the studies or how they defined MCI.
- Only 21 studies using MMSE were used to assess diagnostic accuracy for MCI giving:
- a sensitivity of only 62%
- and a specificity of 87%.
- An alternative test, the MoCA, was found to perform better (in 9 studies) with:
- a sensitivity of 89%
- and a specificity of 75%
- No data was provided on the other tests presumably because there weren’t enough studies.
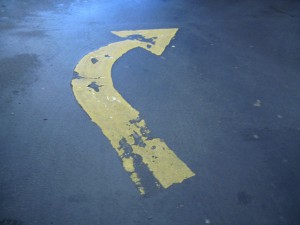
The freely available ACE-R and Mini-Cog instruments may be viable alternatives to the MMSE for detecting dementia.
Conclusions
In short, the MMSE is not a bad screening tool for dementia but it is not miles better than the rest; it’s just really commonly used, probably for historical reasons. The ACE-R and the Mini-Cog are both free to use and may be viable alternatives.
The MMSE is less good in mild cognitive impairment.
Strengths and limitations
What were some of the strengths of this paper?
- The literature search was done well. The authors should be commended for going through so many papers in such a systematic way
- The criteria for inclusion and exclusion were made clear and papers were assessed for quality and data was extracted in a reliable way by two authors
- The meta-analysis itself appears to have been done well
- The paper collates a huge amount of data pertinent to the question: data from over 40,000 people were included in the analysis.
What were the limitations?
- All meta-analyses inherit the limitations of the papers they include. In this case the most obvious limitation is the relative lack of data on alternative cognitive tests like the ACE or Mini-Cog
- The authors mention that the cut-off scores for diagnosing dementia change from study to study. Unlike the example I gave earlier these tests are not simply positive or negative. They give a score (from 0 to 30 in the case of the MMSE) and so the cut-off needs to be determined by the user. In the case of the MMSE, the commonest cut-off was less than 23 or 24, but this was not the case in all of the studies included. This has obvious effects on diagnostic accuracy.
- The authors chose to include Parkinson’s disease in the search criteria, but not Lewy Body dementia or frontotemporal dementia, which I can’t understand given how common they are.
- I didn’t really find the section on mild cognitive impairment very helpful because it seemed like an afterthought. The search terms used to collect the data didn’t seem to be wide enough to capture all the relevant studies for example.
Final thoughts
It’s important to add that whilst this paper focussed on cognitive screening tests, which play an important part in diagnosis, a full clinical assessment of someone with suspected dementia requires a much more detailed approach. Combining information from the history, examination, investigations and cognitive tests greatly improve the diagnostic accuracy. Also where the screening tests are not clear, patients can be referred for much more detailed assessments of cognition performed by neuropsychologists.
Also it is important to remember that the diagnosis of dementia requires evidence of a progressive illness. This means that repeating cognitive tests and looking for change is often more helpful than just a snapshot. This aspect was not covered in this systematic review.
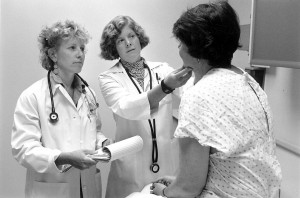
A full clinical assessment of someone with suspected dementia involves much more than a simple cognitive test.
Links
Primary paper
Tsoi KF, Chan JC, Hirai HW, Wong SS, Kwok TY. Cognitive Tests to Detect Dementia: A Systematic Review and Meta-analysis. JAMA Intern Med. Published online June 08, 2015. doi:10.1001/jamainternmed.2015.2152. [Abstract]
Other references
Alzheimer’s Society. The Mini Mental State Examination (MMSE). Website last accessed 27 Jan 2016.
Cognitive tests for dementia: MMSE, Mini-Cog and ACE-R https://t.co/iGCfQH5vu7 #MentalHealth https://t.co/eUt9D5Ikeo
Now using ACE-III
Today @_a_nair asks: Which cognitive test is best for detecting dementia & mild cognitive impairment? https://t.co/KPUC8iyFqW
Meta- analysis of cognitive tests for dementia: MMSE, Mini-Cog and ACE-R https://t.co/2e8X4QOqAY @Mental_Elf
SR suggests freely available ACE-R & Mini-Cog instruments may be viable alternatives to MMSE for detecting dementia https://t.co/KPUC8iyFqW
@Mental_Elf Do you mean the ACE-III? The ACE-R was replaced because it *included* the MMSE and therefore breached copyright.
Cognitive tests for dementia: MMSE, Mini-Cog and ACE-R https://t.co/pYDeszrsja
Cognitive #tests for #dementia: MMSE, Mini-Cog & ACE-R https://t.co/BMw1TMPNs2 @Mental_Elf looks at the #evidence from a #systematicreview
Read our summary of a systematic review & meta-analysis of cognitive tests to detect #dementia https://t.co/KPUC8iyFqW
‘MMSE:not a bad screening tool for dementia but it is not miles better than the rest; it’s just really commonly used’https://t.co/FA7IQlQRd0
El Mini-Cog y ACE-R [de acceso libre] pueden ser alternativas viables al mini-mental para la detección de demencias https://t.co/aDXToC5ADA
Struggling to understand diagnostic tests? #JargonBin sensitivity & specificity. Our blog today will help you! https://t.co/KPUC8iyFqW
Don’t miss: Cognitive tests for dementia – MMSE, Mini-Cog and ACE-R https://t.co/KPUC8iyFqW
@_a_nair explores the results of a systematic review of cognitive tests for #dementia & mild cognitive impairment https://t.co/KPUC8iQgiu
And RBANS :)
Cognitive tests for dementia: MMSE, Mini-Cog and ACE-R https://t.co/RhH73UUKig
Thank you for reminding us as you go about the statistical definitions you’re using. That really helps, and you do it without getting in the way of your discussion. Other Elf bloggers, please note.
Hi @_a_nair Here’s a #StatsBadger for yr blog abt cognitive tests for dementia. Great work! https://t.co/KPUC8iyFqW https://t.co/KTkQZeWEj8
very useful