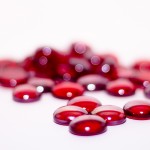
Bipolar disorder is characterised by alternating mania/hypomania and depression. It is diagnosed by direct observation or descriptions of the patient’s behaviour, and these overlap with other conditions, e.g. major depressive disorder (MDD) or schizophrenia (SZ). This can lead to misdiagnosis and inappropriate treatment.
Many publications have attempted to objectively describe bipolar disorder (neuroimaging, genetic risk factors/expression and protein biomarkers). Apart from a few recent exceptions, these have largely suffered from low sample numbers (usually <50), poor reproducibility and major confounding factors.
The authors of a new paper present the case for using a set of protein/peptides/molecules (collectively known as analytes) measured in blood serum. Their performance was tested in:
- Established bipolar disorder patients,
- New onset bipolar disorder,
- Patients with major depressive disorder (MDD) and
- Controls.
Methods
This retrospective study made use of data from several cohorts measured at different times and centres. The cohorts were compounded and used in panel discovery, validation or testing/application.
In the discovery stage, 183 established bipolar disorder patients (any mood state) were compared to 149 controls. The panel of analytes was validated in a further 66 bipolar disorder patients and 44 controls. The panel was tested in samples from bipolar disorder patients in a longitudinal study with patients who later received a bipolar disorder diagnosis. At the time of blood sampling they did not receive bipolar disorder medication. This is important because medication could have affected the measurements, leading to a bipolar disorder medication panel as opposed to a bipolar disorder panel. It was also tested in patients diagnosed with MDD who went on to develop mania or hypomania.
Participant’s serum was tested with a multiplex immunoassay platform, where several analytes were measured in tandem. Data in the discovery and validation stages were combined using an algorithm to compensate for batch effects. This aimed to make the subjects comparable within and between the studies.
They used lasso regression (a form of logistic regression) to highlight 20 proteins that strongly associate to bipolar disorder. Lastly, a receiver operating curve was used to assess the panel’s performance using the area under the curve (AUC) where 1.00 is perfect prediction and 0.50 is equivalent to a coin toss.
Results
In the discovery phase, 20 proteins had a commendable predictive ability (AUC=0.90 out of 1.0). Seven of these had proinflammatory activity, the rest were anti-inflammatory or involved in lipid transport and metalloprotease activity. These overlapped poorly with blood based panels from MDD (Papakostas et al, 2013) and SZ (Schwartz et al, 2012).
When tested in patients before they received a diagnosis of bipolar disorder the panel’s predictive ability bordered on good; AUC=0.79. When using the panel to distinguish between Pre-BD and schizophrenia patients, the panel performed with an excellent AUC of 0.91. When using the panel to differentiate between healthy controls and schizophrenia patients it performed poorly with an AUC of 0.52, the same as tossing a coin.
It was also tested on 102 samples from patients with first onset of depression, 12 of which, went on to develop BD. In these 12 patients, the performance of the model was very good (AUC=0.84). In the cohort’s remaining 90 patients in a depressive episode, the BD analyte panel performed poorly with an AUC=0.64.
Conclusions
The authors show, for the first time, that a blood-based protein panel can identify bipolar disorder and distinguish it against major depressive disorder or schizophrenia and this has potential use in differential diagnosis.
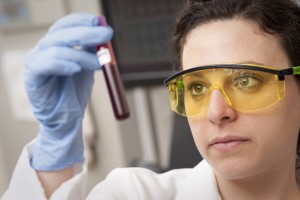
Could these findings be usefully applied to clinical practice?
Strengths and limitations
This paper pooled data from a number of cohorts and used meta-analysis techniques to build a model that biologically and objectively characterises bipolar disorder patients. However, the sheer heterogeneity of samples used to establish the panel is a weakness; the only common demographics in the study were sex and age.
The samples used in the study were collected from both bipolar disorder I and bipolar disorder II patients, at different test centres, in different countries, at different times,by different researchers using different methodologies, that tested samples on different versions of multiplexed immunoassay, with different diagnostic manuals and different practice habits, (applies to psychiatry in general) operating in various seasons with different inclusion/exclusion criteria. To make the measurements consistent across all cohorts the authors statistically adjust the measurements.
However, this heterogeneity may act as a strength as the panel performed considerably well to identify bipolar disorder patients and consistently failed to categorise controls/MDD/SZ as bipolar disorder. This also hints at the possibility of filtering out drug effects on blood serum; considering bipolar disorder is treated with mood stabilisers, antidepressants, antipsychotics, anti-epileptics and sedatives, it is unlikely that any of these presented a signal that could have made it to the validated panel.
A notable strength is the panel’s performance in pre-diagnostic bipolar disorder patients, this also works to confirm that the panel was detecting bipolar disorder and not bipolar disorder medication. Additionally, the panel could prove the bipolar disorder analyte fingerprint is relatively stable in reference to disease progression, as patients with established long-standing bipolar disorder tested comparably to those with early stage/pre-symptomatic bipolar disorder.
The main weakness of this study is that it ultimately relies on subjective diagnoses. This produces a circular argument whereby the authors prove they can build an objective model based on subjective diagnoses.
Summary
The difficulties surrounding differential diagnosis are not specific to bipolar disorder, it extends to all psychiatric disorders. Furthermore, outside of psychiatry, no medical condition is diagnosed with a set of questions and behavioural observations; an archaic, non-objective method to assess debilitating conditions.
This could be used as a tool to aid diagnosis; it would not replace psychiatrists. However, I feel it will have a slow uptake in the clinic even if it improves disease management because the model is quite complicated and requires a trained statistician to interpret the data to give a score for how likely that patient is to have bipolar disorder. This complexity and innovation add to barriers in clinical application. However, its requirement for change of current practice; shifting it to a more evidence-based approach, should hasten its adoption.
The authors successfully show us that bipolar disorder can be biologically identified even though we do not fully understand how these markers relate to symptoms or disease aetiology.
Ultimately, we should enable patients to choose how they receive a diagnosis and something like this could speed this up from years to weeks.
Links
Primary paper
Haenisch et al. (2015) Towards a blood-based diagnostic panel for bipolar disorder. Brain Behav Immun, 2015, doi: 10.1016/j.bbi.2015.10.001
Other references
Papakostas et al. (2013) Assessment of a multi-assay, serum-based biological diagnostic test for major depressive disorder: a pilot and replication study. Mol Psychiatry, 2013, 18, pp. 332–339 [Abstract]
Schwartz et al. (2012) Identification of a blood-based biological signature in subjects with psychiatric disorders prior to clinical manifestation. World J. Biol. Psychiatry, 2012, doi:10.3109/15622975.2011.599861 [Abstract]
Photo credits
Improving diagnosis of bipolar disorder: can blood-based diagnostic panels… https://t.co/S3oe2uZcYZ #MentalHealth https://t.co/OfPnjvO56C
Improving diagnosis of bipolar disorder: can blood-based diagnostic panels help? @MentalElf looks at the evidence https://t.co/wVqtfkbcLt
@TheMMP1 remember that blog i was tell you about…https://t.co/ohOuxJUHC1
https://t.co/ohOuxJUHC1
@Amardeep131313
@t_saif
مقالي الجديد يشرح بحث جديد لتشخيص مرض القطبية عن طريق عينة دم. ارجو ان ينال اعجابكم و مشكور للنشر
https://t.co/ohOuxJUHC1
Don’t miss: Improving diagnosis of bipolar disorder: can blood-based diagnostic panels help? https://t.co/0iB9XG15VA #EBP
@Mental_Elf This is quite interesting – I’ll read the full paper tomorrow. Thanks for illuminating explanatory post.
Improving diagnosis of bipolar disorder: can blood-based diagnostic panels help? https://t.co/sVKolUfxK1 via @sharethis
Improving diagnosis of bipolar disorder: can blood-based diagnostic panels help? https://t.co/kX0BYpYRxl #EBP via @Mental_Elf
Improving diagnosis of bipolar disorder: can blood-based diagnostic panels help? https://t.co/PEiJFhfDal #EBP via @Mental_Elf
Improving diagnosis of bipolar disorder: can blood-based diagnostic panels help? https://t.co/TtlOU27i9q