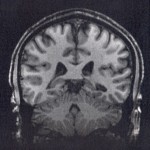
Papa Elf sent me a paper (Kambeitz et al, 2015) looking at a meta-analysis of a technique involving brain imaging to try and successfully classify study participants as meeting the criteria for schizophrenia or not.
The study uses a type of diagnostic screening tool using brain imaging combined with “multi-variate pattern recognition” which usually includes a “machine learning algorithm” to improve predictive accuracy. This involves looking at interconnected regions of the brain for patterns that tend to be associated with the diagnosis of schizophrenia in the participants in the study, which differentiates them from participants who did not meet the criteria. If a machine learning algorithm program is used, this will keep trying to improve its’ performance by successfully classifying the subjects.
It’s important to remember that these changes in structure are not necessarily the cause of schizophrenia, but may be the result of a process (not necessarily biological) leading to a diagnosis of schizophrenia.
This is a meta-analysis looking at the effectiveness of a diagnostic screening tool for schizophrenia using multivariate pattern recognition techniques on brain images. This is part of the largely unsuccessful quest for clinically useful biomarkers in psychiatry for “functional” disorders (i.e. no obvious “organic” cause) like schizophrenia.
Fortunately for me, the EBMH Journal recently published an article about how to appraise a meta-analysis of diagnostic screening tools, so I have used this approach to help write my blog (Takwoingi et al, 2015).
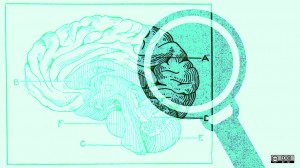
Could neuroimaging become part of the routine diagnostic process in the future?
Methods
I will quote the paper directly as to which studies are included:
To be included in the meta-analysis a paper needed to report results of a neuroimaging-based multivariate classification model separating patients with schizophrenia from HC {Healthy Controls}. We included all available multivariate approaches such as support-vector machines, random forests, discriminant analysis, logistic regression, neural networks, as well as combinations thereof.
There is also a further condition that results must be expressed in a way to allow calculation of “true positives (TP), true negatives (TN), false positives (FP), and false negatives (FN)” (or the authors had responded to requests for this data).
Reasonably comprehensive search strategies were used and the quality of the studies was assessed using a reasonably established technique. Data extraction was done by two researchers separately and any disagreements resolved using a conference consensus. A bivariate model with random effects was used to analyse the statistics and an appropriate statistics package was used (Takwoingi et al, 2015). Studies were grouped according to the type of brain imaging used and combined for an overall result.
Studies from 1991-2013 were used, but crucially little information in the paper was given as to which diagnostic criteria were used for schizophrenia (I suspect DSM-IV for the majority of studies, but this is just an informed guess on my part).
Secondary analysis looked at various patient characteristics to see if they influenced sensitivity or specificity of the tests such as age, gender, severity of symptoms, first episode vs chronic schizophrenia, or amount of medication prescribed. Differences in performances between different imaging techniques were examined.
Results
399 initial papers were whittled down to 38 studies with a total of 1,602 participants who met criteria for schizophrenia and 1,637 healthy controls. The data from these 38 studies was then entered into the analysis.
Sensitivity in this paper is the proportion of people that the screening test correctly identifies as meeting criteria for schizophrenia, expressed as a percentage. Specificity is the proportion of people the screening test correctly identifies as not meeting criteria for schizophrenia, expressed as a percentage.
To quote the paper:
Across all studies, neuroimaging-based classifiers separated SZ [schizophrenia] from HC {healthy controls} with a sensitivity of 80.3% (95% CI: 76.7 to 83.5%) and a specificity of 80.3% (95% CI: 76.9 to 83.3%).
In the secondary analysis, older patients demonstrated higher sensitivity; patients with a higher positive to negative symptom ratio had greater specificity; chronic schizophrenia participants had greater sensitivity than first episode participants and people on higher doses of antipsychotics had greater specificity than those on low doses. It may be that medication altered brain structure in people on it for longer or at higher doses making it easier for the test to differentiate participants with schizophrenia from healthy controls (Ho et al, 2011). Year of publication was not associated with significantly different performance.
There was overlap in confidence intervals of sensitivity and specificity but resting state functional MRI was superior to structural MRI in terms of sensitivity. Visual inspection of Forrest plots suggested publication bias was unlikely.
Conclusion
As an overall conclusion
-
80% of participants with schizophrenia and 80% of “healthy controls” were correctly identified as such, which means:
- 20% of participants with schizophrenia were incorrectly identified as being a “healthy control”
- 20% of participants without schizophrenia were incorrectly classified as having schizophrenia
Strengths and limitations
- This meta-analysis used a standardised and clear methodology to identify studies
- The perennial bugbear of meta-analysis: were they counting apples and oranges as the same? It’s not clear how different the types of multi-variate pattern recognition types were from each other. Using the Mark 1 Eyeball and looking at individual results, there was some heterogeneity of sensitivity and specificity
- The biggest weakness is use of different diagnostic criteria in different studies. This type of bivariate meta-analysis can only be done if the same diagnostic threshold and criteria are used in all studies (Takwoingi et al, 2015). Although there is likely to be significant overlap between patients diagnosed as having schizophrenia by related criteria such as DSM II, DSM IIR and DSM- IV, the amount of heterogeneity still makes a combined meta-analysis of diagnostic tests using different diagnostic criteria difficult to perform. It would be better to either use only the studies with a particular diagnostic criteria (e.g. DSM-IV) or perform separate meta-analysis for the different diagnostic criteria.
As in my last blog, I typed the figures from the study into www.clinicalutility.co.uk. Yet again it classified the usefulness of this type of biomarker as “Fair”. So even with clever algorithms and fancy dan brain imaging, I would suggest that this type of test is not ready for routine use. The lack of association of different performance with year of publication suggests that we may not see any improvement in performance of this technique in the future.
It looks like I don’t get to be a “proper doctor” and order diagnostic imaging scans for schizophrenia just yet.
Links
Primary paper
Kambeitz J, Kambeitz-Ilankovic L, Leucht S, Wood S, Davatzikos C, Malchow B, Falkai P, Koutsouleris N. Detecting neuroimaging biomarkers for schizophrenia: a meta-analysis of multivariate pattern recognition studies (PDF). Neuropsychopharmacology. 2015 Jun;40(7):1742-51. doi: 10.1038/npp.2015.22. Epub 2015 Jan 20.
Other references
Ho BC, Andreasen NC, Ziebell S, Pierson R, Magnotta V. Long-term antipsychotic treatment and brain volumes: a longitudinal study of first-episode schizophrenia. Arch Gen Psychiatry. 2011 Feb;68(2):128-37. doi: 10.1001/archgenpsychiatry.2010.199.
Sarpal DK, Argyelan M, Robinson DG, Szeszko PR, Karlsgodt KH, John M, Weissman N, Gallego JA, Kane JM, Lencz T, Malhotra AK. (2015) Baseline Striatal Functional Connectivity as a Predictor of Response to Antipsychotic Drug Treatment. Am J Psychiatry. 2015 Aug 28:appiajp201514121571. [Epub ahead of print]
Takwoingi Y, Riley RD, Deeks J. (2015) Meta-analysis of diagnostic accuracy studies in mental health. Evid Based Mental Health ebmental-2015-102228 Published Online First: 7 October 2015 doi:10.1136/eb-2015-102228
Photo credits
Rise of the machine learning algorithm: the future of diagnosing… https://t.co/jT8FzmJA3p #MentalHealth https://t.co/zVel25ZF9m
RT iVivekMisra Rise of the machine learning algorithm: the future of diagnosing… https://t.co/QNp9ZfhGtr #MentalH… https://t.co/d3gIHzXtqv
Are brain scans and computer algorithms the future Of diagnosing schizophrenia ? My latest elf blog https://t.co/AaeJy4TGET
The diagnostic issue is not distinguishing people with schizophrenia from healthy controls; it’s distinguishing between different diagnoses in people who present with mental health problems. I guess before you can do that you might want studies that do the HC vs Sz comparison, but the fact that performance is at best ‘fair’ on that discrimination confirms my suspicions that this is a hopeless approach for clinical diagnosis.
This is completely true – at the moment those doing machine learning rarely take on the difficult challenge of distinguishing patients with different diagnoses. There are some interesting approaches though that are trying to use these techniques to distinguish patients who go on to have different outcomes, which is likely to be more successful as well as clinically more useful!
@AllenFrancesMD is brain scanning plus machine learning algorithms the future of diagnosing schizophrenia ? https://t.co/AaeJy4TGET
Rise of the machine learning algorithm: the future of diagnosing schizophrenia? https://t.co/umSft36D1Y via @sharethis
RT @Mental_Elf: Neuroimaging biomarkers for detecting schizophrenia – a “hopeless approach for clinical diagnosis” says @deevybee https://t…
Today @SameiHuda on meta-analysis looking at detecting neuroimaging #biomarkers for #schizophrenia https://t.co/TLUDLF5SUJ
Dorothy Bishop is on the mark. There are no prizes for distinguishing Sz from normal with fancy scans. Easiest way to do that would be to sit down with the persons over a beer – you would beat the machine learning algorithm every time.
Besides, the algorithm gives the clinician no context – no family history, no illness history, no nuances of symptoms, no data on stability of diagnosis, no test-retest reliability, and so on. It’s a disembodied scholastic exercise predicated on an invalid gold standard.
Meta-analysis of multivariate pattern recognition studies aims to detect neuroimaging biomarkers for schizophrenia https://t.co/TLUDLF5SUJ
Rise of the machine learning algorithm: the future of diagnosing schizophrenia? https://t.co/8MsO5uE5eb
Can brain imaging help diagnose schizophrenia? https://t.co/TLUDLF5SUJ
Don’t miss: Rise of the machine learning algorithm: the future of diagnosing schizophrenia? https://t.co/TLUDLF5SUJ #EBP
Rise of the machine learning algorithm: the future of diagnosing schizophrenia? https://t.co/0y9NLTXNmo
[…] 1Machine learning algorithm: future of diagnosing schizophrenia? […]