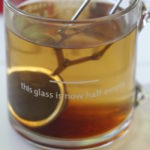
Suicide is the biggest killer of young people in the UK, and accounts for a significant proportion of deaths in every other age group. Many risk factors for suicide in the general population are well established and quite obvious, for example mental illness, drug misuse, trauma, and poverty, but there is a much smaller literature base on the risk factors for suicide in psychiatric inpatients. This is important, because psychiatric wards are exactly where risk assessment and intervention for suicide happen most intensively. A clear idea of who to focus those interventions on, of who is “high risk”, would be very useful.
Therefore, Large et al have undertaken a meta-analysis of studies (2018) that define a high risk model for inpatient suicide, and test the strength of that model in predicting suicide. They note that a 2011 meta-analysis, which shares the same first author as their own paper (Large et al, 2011), had some methodological drawbacks and that several new pieces of primary research have been published since then.
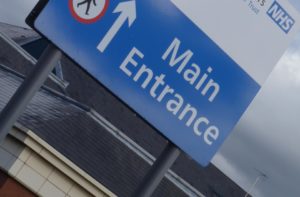
Risk factors for suicide in the general population are well established, but we know relatively little suicide risk factors for psychiatric inpatients.
Methods
The reviewers searched study databases for peer-reviewed, published, cohort or case-controlled studies that:
- Compared variables of psychiatric inpatients who died by suicide during their admission to those who did not
- Used two or more of those variables to define a “high risk” group either by a cut-off scoring system or by multivariate statistical methods
- Calculated true and false positive and negative rates, or sensitivity and specificity, for membership of this high risk group as a predictor for suicide.
For the statistics experts amongst you, the strength of each study was rated using the Newcastle-Ottawa scale, and publication bias was assessed using Egger’s regression and Duval and Tweedie’s trim and fill method.
Results
17 studies reporting 18 samples of patients were identified, published between 1998 and 2016. Some studies collected data prospectively and others retrospectively, but all studies only defined their “high risk” criteria after the data collection had happened; none did it before and so none tested them in real time.
191,944 patients were examined across all studies, with huge variation between studies (from 36 to 126,103 patients in the control groups). 1,718 patients who died by suicide were examined across the studies, again with variation between studies (from 19 to 326). Six studies were cohort designs and the other 12 were case-control designs, accounting for some of the variation in group sizes between studies.
A mean of 78.6 variables were collected on each patient (range 14-272) and a mean number of 6.1 variables were included in the high-risk models (range 2-16).
The pooled odds ratio of dying by suicide in a high risk group (compared to not being high risk) was 7.1, and there was wide heterogeneity between studies (first quartile 3.4, median 8.8, third quartile 26.1, highest 94.8).
The pooled odds ratio for case-control studies was much higher (9.7) than cohort studies (1.7).
The pooled sensitivity for high risk categorisation was 53.1% (range 10-90). That is, 53.1% of patients who died by suicide were deemed “high risk”. The pooled specificity for low risk categorisation was 84.2% (range 65-100). That is, 84.2% of patients who did not die by suicide were deemed “low risk”.
The positive predictive value in the cohort studies was 0.43%. That is, 0.43% of patients deemed “high risk” died by suicide.
The 18 samples used a total of 110 variables as part of their high risk criteria:
- 16 used recent or previous suicidal behaviour
- 11 used depressed mood or affective disorder
- “Indicators of more psychiatric hospital care” were used in eight
- Male gender was used in seven
- Social stress or loss was used in six
- Schizophrenia was used in five
- Going AWOL, aggression, family history of suicide, lack of insight, involuntary detention, substance abuse and various others were used less frequently
Regression models were used to define the high-risk groups in 11 studies, and a simple cut-off score was used in the other seven studies, with no significant difference in pooled odds between these two groups.
Egger’s regression analysis was not statistically significant, but the Duval and Tweedie’s trim and fill method identified four hypothetically missing studies with a weaker association between being high risk and dying by suicide, which if included would have reduced the adjusted odds ratio to 5.1.
Studies that gathered their data prospectively (mostly cohort studies, which were also much larger) had significantly lower pooled odds of suicide than studies which were retrospective. 11 studies scored two (out of five) on the Newcastle-Ottawa scale for study strength, six scored three and one scored four. There were no statistically significant differences on study results mediated by these scores.
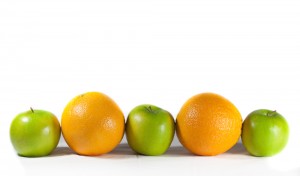
The reviewers concluded: “the very high degree of observed heterogeneity indicates uncertainty about our ability to meaningfully distinguish inpatients according to suicide risk.”
Discussion
This paper is an honest attempt to do what the authors state: to analyse and synthesise models of “high risk” to see how accurately they predict suicide on psychiatric wards. They appear to have done a good job of including relevant studies, processing all the data, and communicating the results. They note that a degree of publication bias, retrospective data collection and chance associations may have inflated the key findings somewhat. These are fair points; nonetheless the basic methodology of their paper is sound enough.
The real talking point is the lack of practical application of this area of research. They haven’t tried to sugar-coat their thoughts on this, and I won’t either. In simple terms, they found that although a “high risk” patient was seven times more likely to die by suicide during their admission than a “low risk” patient, the majority of risk factors for suicide were so common, like depression, previous attempts at suicide, and social stress or loss, that they were no use for predicting suicide in individual patients. Only one in 230 patients classified as high risk by these models would die by suicide during their admission (about one patient every two or three years on an average ward), and the models still missed about half of suicides. This makes it impossible to use these models to predict suicide or target suicide reduction interventions at specific patients in clinical practice.
The pessimism around predictive power that this study discusses is already shared by guideline writers and experts around the world. NICE have long advised against using risk assessments to predict the future likelihood of suicide, and the National Confidential Enquiry into Suicide and Safety in Mental Health make it clear that risk assessment should not be predictive or a “checklist”, but a personalised, dynamic, family-involved process to inform a collaborative approach to management.
It is from such an angle that suicide-reduction research should now be approached. It has proven fruitless to search huge cohorts of patients for top-down factors that increased suicide risk and can predict suicide in others. Instead, we should be focusing on individuals, in a bottom-up approach to identifying their own, personal risk factors as a means of reducing them in a bespoke way. Interventions for assisting with this process could be and should be tested naturalistically. After all, as Large et al (2018) mention, what even is the point of identifying a high risk group if we don’t even know what might help them?
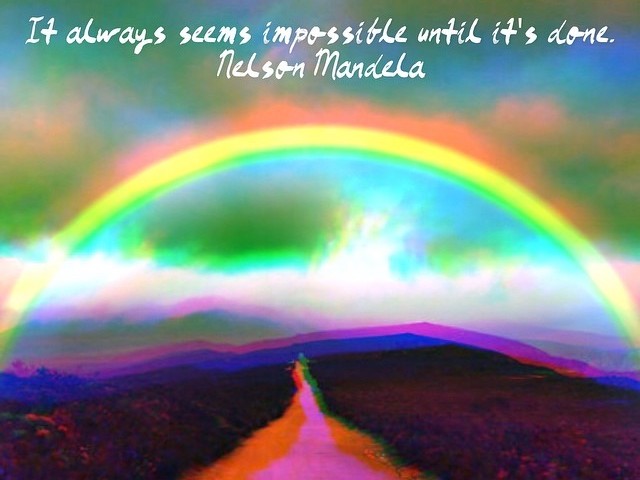
It’s impossible to use the suicide risk assessment models in this review to predict suicide or target suicide reduction interventions at specific patients in clinical practice.
Conflicts of interest
Alex Langford is running four marathons and three ultramarathons in 2019 in aid of suicide prevention charity Papyrus. Mental Elf founder André Tomlin has made a small personal donation to this worthy cause and you are very welcome to donate too!
https://www.justgiving.com/fundraising/alexlangford
Links
Primary paper
Large, M., Myles, N., Myles, H., Corderoy, A., Weiser, M., Davidson, M., & Ryan, C. (2018). Suicide risk assessment among psychiatric inpatients: A systematic review and meta-analysis of high-risk categories. Psychological Medicine, 48(7), 1119-1127. [PubMed abstract]
Other references
Large M, Smith G, Sharma S, Nielssen O and Singh SP (2011) Systematic review and meta-analysis of the clinical factors associated with the suicide of psychiatric in-patients. Acta Psychiatrica Scandinavica 124, 18–29.
National Institute for Health and Care Excellence (NICE). Self-harm in over 8s: long-term management. Clinical guideline. London: NICE, 2011.
The assessment of clinical risk in mental health services. National Confidential Inquiry into Suicide and Safety in Mental Health (NCISH). Manchester: The University of Manchester, 2018.
Photo credits
Is it true that suicide often happens when a person is in a partial recovery phase (and therefore has more capability to do it)? (if so these higher risk periods could be highlighted and managed?)
Has any psychiatrist considered that the appalling things done to psychiatric patients might be a major contributory factor?
Think all of us who have been forcibly detained or have experienced our loved ones forcibly detained would like to see solid research in to the harm coercive practices have and when these become suicide triggers. If only because we despair that so much more money seems to be put in to research than it does on regulating institutions and staff who are in effect abusive. Don’t know why studies keep looking at risk prediction when all studies say usual tools arent safe. Now if they asked patients to design assessments without doubt the violence of wards and complicity or abuse by staff would jump to top of all lists. I know ward staff arent the slightest bit interested in what keeps patients safe. What would really like to see is every consultant, every RMN, every advocate and every single AMHP raise safeguarding and incident reports as all policies tell them to. That wpuld be so much more effective at preventing serious harm and suicide. For me, I have never experienced violence like that from MH nurses to the point I am far too terrified too ask for help when at risk. Maybe more time with those of us who have survived suicide would inform services on how to prevent. But they ALL have to want to and the culture is not one that gives me hope